
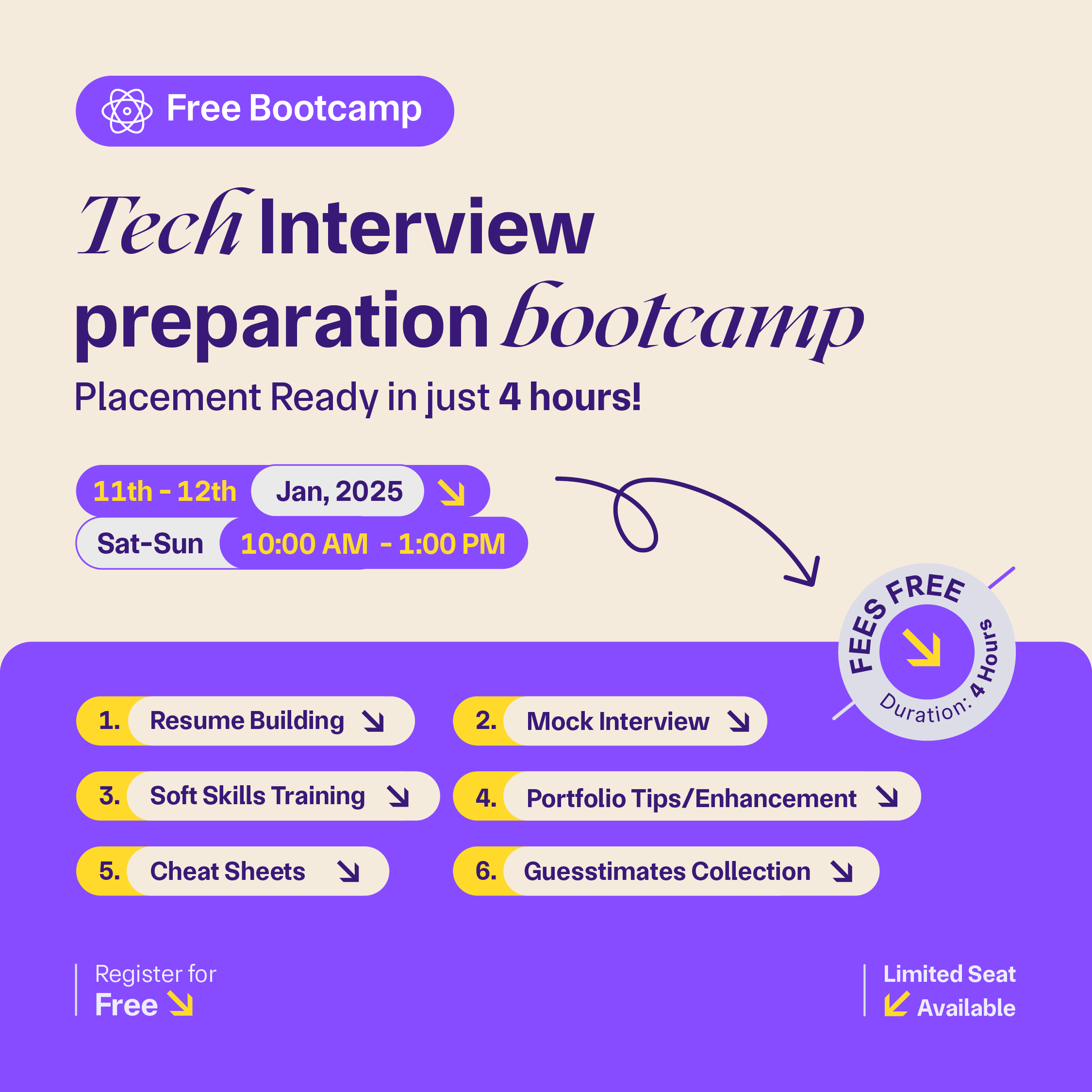
Time series data visualization is a crucial technique for analyzing data points collected over time, allowing for a clearer understanding of trends, patterns, and anomalies. By representing this data graphically, analysts can easily identify long-term trends and seasonal variations that may not be apparent in raw data. Common visualization methods include line charts, which effectively display continuous data, and bar charts, which are useful for discrete time intervals.
Area charts emphasize cumulative data, while candlestick charts are favored in finance for showing price movements. Heatmaps offer a visual representation of data intensity over time, helping to spot patterns at a glance. Effective time series visualization also involves best practices such as clear labeling, consistent time intervals, and the judicious use of color to enhance readability.
These visual tools not only aid in data analysis but also support forecasting and decision-making by presenting complex information in an easily digestible format. Ultimately, mastering time series visualization empowers businesses and researchers to derive actionable ins.
A time series is a sequence of data points collected or recorded at successive points in time, typically at uniform intervals. This type of data is used to track changes over time and is prevalent in various fields such as finance, economics, environmental studies, and healthcare.
Key characteristics of time series data include:
Time series analysis helps identify these components, enabling better forecasting, anomaly detection, and informed decision-making based on historical data trends.
Time series data can be categorized into various types based on different criteria. Here are the primary types of time series data:
Univariate time series data involves a single variable recorded over time, allowing for focused analysis on that specific data point. This type of data is essential for understanding how a single metric changes, making it ideal for tasks like forecasting and trend analysis.
For instance, daily stock prices of a particular company can provide insights into market behavior and investor sentiment. By examining patterns, anomalies, and trends in univariate data, analysts can make informed decisions, such as when to buy or sell assets.
Multivariate time series data includes multiple variables recorded simultaneously over time, providing a more comprehensive view of complex relationships. This type of analysis helps identify how different factors interact and influence each other.
For example, analyzing economic indicators such as GDP, inflation rates, and unemployment rates together can reveal insights into economic health and policy effectiveness. Multivariate time series models can help forecast future trends by considering the interdependencies between variables, making them invaluable in fields like economics and finance.
Continuous time series data consists of observations recorded at any moment, creating a fluid representation of changes over time. This type of data is particularly useful for applications that require real-time monitoring or tracking of dynamic systems.
For example, temperature readings taken every minute can help understand fluctuations throughout the day and identify patterns such as daily cycles. Continuous data provides a detailed view that allows for precise analysis, although it may require advanced techniques for effective visualization and interpretation.
Discrete-time series data involves observations recorded at specific, often equally spaced intervals. This type of data is common in business and finance, where measurements are taken at regular intervals, such as monthly sales figures or quarterly earnings reports.
Discrete-time series allows for straightforward analysis of trends and seasonal patterns, making it easier to identify cyclical behaviors over fixed periods. While discrete data may not capture real-time fluctuations as finely as continuous data, it provides clarity and ease of interpretation in trend analysis.
Seasonal time series data exhibits regular patterns or fluctuations at specific intervals due to seasonal effects. This type of data is essential for businesses that experience periodic changes, such as retail stores that see increased sales during holiday seasons.
For instance, ice cream sales typically peak in the summer months, reflecting consumer behavior influenced by temperature and seasonal activities. Understanding these patterns allows businesses to plan inventory, marketing strategies, and resource allocation effectively.
Non-seasonal time series data does not exhibit regular patterns or fluctuations over time, making it more unpredictable. Various external factors can influence this type of data, but it lacks a consistent cycle.
For example, annual GDP growth rates may fluctuate due to economic conditions but do not follow a predictable seasonal pattern. Analyzing non-seasonal time series requires different approaches, as the absence of regular cycles can complicate forecasting and trend identification.
Stationary time series data is characterized by constant statistical properties, such as mean and variance, over time. This type of data is crucial for many time series analysis techniques, as many statistical models assume stationarity.
For instance, daily returns on stock might exhibit random fluctuations around a constant mean, making them suitable for analysis using methods like ARIMA. Stationary data allows analysts to make reliable predictions, as the underlying statistical characteristics do not change over time.
Non-stationary time series data features changing statistical properties over time, such as trends or varying variance. This type of data often requires transformation (e.g., differencing) to achieve stationarity before analysis. For example, a company’s revenue might tend to grow year over year, reflecting an upward trend. Recognizing and addressing non-stationarity is crucial for accurate forecasting and modeling, as it impacts the validity of many statistical techniques.
Analysts must apply appropriate methods to handle non-stationary data effectively. Understanding these different types of time series data allows analysts to select the right analytical methods and visualization techniques, ultimately leading to more accurate insights and informed decision-making.
Here are several effective ways to visualize time series data, each serving different analytical purposes and presenting data in unique formats:
Line charts are the most common method for visualizing time series data. They display data points along a continuous line, which allows for easy identification of trends, fluctuations, and patterns over time.
This format is particularly effective for showcasing continuous data, such as stock prices or temperature changes, where the focus is on how the variable evolves. By connecting individual data points, line charts help viewers quickly grasp the overall direction and dynamics of the data.
Area charts share similarities with line charts but add a filled area beneath the line, emphasizing the magnitude of changes over time. This visualization effectively highlights cumulative totals, making it easier to see how values accumulate.
Area charts are particularly useful for visualizing total sales over a period, illustrating both trends and the scale of changes in a visually impactful way. The filled areas help convey volume and provide a clearer context for the data’s progression.
Bar charts display discrete time intervals using bars to represent values, making them ideal for comparing different categories across time.
These charts can be oriented vertically or horizontally and are especially effective for visualizing monthly sales figures or yearly revenue, as they allow for straightforward comparisons between distinct periods. By clearly delineating each time interval, bar charts make it easy to identify peaks and troughs in the data.
Candlestick charts are widely used in financial markets to convey the open, high, low, and close prices of securities over specific time intervals. Each "candlestick" provides a visual representation of price movement, with the body of the candlestick indicating the range between opening and closing prices.
This format is essential for traders who analyze price patterns, as it allows for a quick assessment of market behavior and potential future movements based on historical data.
Heatmaps use color gradients to represent data values across two dimensions, with time typically on one axis and another variable on the other. This visualization technique is particularly effective for identifying patterns in large datasets, such as website traffic by hour and day.
By employing color to indicate intensity or frequency, heatmaps enable analysts to quickly pinpoint peak activity periods, facilitating deeper insights into user behavior and trends over time.
Scatter plots are used to visualize the relationship between two variables, with time represented on one axis. This type of visualization is beneficial for observing correlations or trends over time, as it allows for the identification of patterns among data points.
For example, a scatter plot can show the relationship between time and sales growth, helping analysts detect trends or anomalies in the data and providing valuable insights for decision-making.
Time series decomposition plots break down a time series into its fundamental components: trend, seasonality, and residuals. This type of visualization is invaluable for understanding the underlying patterns in the data.
By isolating these components, analysts can more clearly see how trends and seasonal effects influence the overall data. This clarity aids in forecasting and helps stakeholders make informed decisions based on the identified components.
Box plots provide a summary of the distribution of data points over time, highlighting the median, quartiles, and outliers. This visualization technique is useful for summarizing variability across different periods, such as monthly sales performance.
By presenting data in this way, box plots allow analysts to quickly assess the spread and central tendency of the data, making it easier to identify trends and anomalies that warrant further investigation.
Combination charts overlay multiple types of visualizations, such as line and bar charts, to present a comprehensive view of the data. This approach is beneficial for showing relationships between different datasets, such as total sales represented by a bar chart alongside profit margins displayed as a line chart.
By combining visual elements, combination charts facilitate easier comparisons and a more nuanced understanding of how different metrics interact over time.
Streamgraphs are a dynamic visualization technique that represents data over time as a flowing, organic shape. This format is a variation of area charts and is particularly effective for showcasing changes in categories over time, such as media consumption across different platforms.
Streamgraphs emphasize the overall flow of data, making it easier to see how categories rise and fall relative to one another. This visually appealing representation helps engagingly convey complex information.
Here’s a look at some of the best platforms for data visualization, each offering unique features and capabilities to help users create impactful visual representations of their data:
Tableau is a leading data visualization tool known for its powerful capabilities and user-friendly interface. It allows users to create interactive and shareable dashboards that can display trends, variations, and insights in data.
With a wide range of visualization options and the ability to connect to various data sources, Tableau is suitable for both beginners and advanced users. Its drag-and-drop functionality makes it easy to create complex visualizations without extensive coding knowledge.
Microsoft Power BI is a robust business analytics tool that enables users to visualize data and share insights across their organization. It integrates seamlessly with other Microsoft products and offers a range of visualization options, including charts, graphs, and maps.
Power BI's interactive dashboards and real-time data updates make it an excellent choice for business intelligence, allowing users to make data-driven decisions quickly.
Google Data Studio is a free, web-based data visualization tool that allows users to create customizable dashboards and reports. It integrates well with other Google services, such as Google Analytics and Google Sheets, making it a convenient option for those already using the Google ecosystem.
Users can create interactive visualizations with drag-and-drop features, and the platform supports collaboration, enabling teams to work together on data presentations.
D3.js (Data-Driven Documents) is a powerful JavaScript library for producing dynamic and interactive data visualizations in web browsers. It allows developers to create highly customizable visualizations using HTML, SVG, and CSS.
While it requires coding knowledge, D3.js offers unparalleled flexibility for creating unique visual representations and is widely used by developers for advanced data visualizations on websites.
Plotly is a versatile graphing library that enables users to create interactive plots and dashboards in Python, R, and JavaScript. It provides a wide array of visualization options, including 3D charts, heat maps, and geographical maps.
Plotly's ease of use and interactive features make it suitable for both data scientists and analysts who want to present complex data in an accessible format.
Qlik Sense is a data visualization and business intelligence tool that allows users to create interactive reports and dashboards. Its associative data model enables users to explore data from multiple angles, providing deeper insights.
Qlik Sense also offers strong collaboration features, allowing teams to share insights and analyses easily. The platform is designed for both novice and experienced users, making it a flexible choice for organizations.
Chart.js is a simple yet flexible JavaScript library for creating animated, interactive charts. It is easy to use and integrates well with web applications, making it a great choice for developers who want to add data visualizations to their projects without extensive overhead.
Chart.js supports various chart types, including line, bar, radar, and pie charts, allowing for straightforward data representation.
Infogram is a user-friendly web-based tool designed for creating infographics and interactive visualizations. It provides a wide range of templates and design elements, making it easy for users to create visually appealing presentations without needing design skills.
Infogram is particularly useful for marketers and educators looking to present data engagingly.
Sisense is a business intelligence platform that offers powerful data visualization capabilities. It allows users to create dashboards and reports that can analyze large datasets efficiently. Sisense’s embedded analytics capabilities enable organizations to integrate data visualizations directly into their applications, providing users with seamless access to insights within their workflows.
Looker is a business intelligence tool that focuses on data exploration and visualization. It allows users to create interactive dashboards and reports using a modeling language called LookML, which simplifies data manipulation.
Looker’s integration with various data sources and its emphasis on collaboration make it a strong choice for teams looking to analyze and visualize their data effectively.
Here are some compelling examples of time series data visualization across various contexts, illustrating how different visualization techniques can effectively convey insights:
A line chart is a classic visualization used to depict the daily stock prices of a company over a year. This type of visualization is particularly effective for investors, as it allows them to quickly identify trends in stock prices, such as upward or downward movements.
By connecting data points representing daily closing prices, the line chart clearly illustrates significant fluctuations and patterns, enabling investors to make informed decisions based on historical performance and market behavior.
An area chart displaying total monthly sales for a retail store over the past two years is an excellent way to visualize sales data. By filling the area under the line, this chart emphasizes cumulative sales trends, making it easier for managers to spot seasonal peaks and troughs.
This visualization is beneficial for understanding how sales fluctuate throughout the year, helping businesses plan inventory, optimize marketing strategies, and forecast future sales based on past performance.
A heatmap showing hourly temperature variations throughout a month provides an intuitive way to visualize temperature changes over time. By using color gradients to represent different temperature values, this visualization enables viewers to quickly identify the hottest and coldest times of the day, as well as observe trends over different days.
Such insights are particularly valuable for meteorologists, researchers, and anyone interested in understanding climate patterns.
Candlestick charts are widely used in financial markets to display price movements of cryptocurrencies over specific time intervals, such as a week. Each candlestick provides detailed information about the opening, closing, and high and low prices during that period.
This rich visualization helps traders analyze market behavior, allowing them to identify trends, reversals, and potential trading opportunities based on historical price patterns and volatility.
A scatter plot visualizing website traffic over a year, with time on the x-axis and visitor count on the y-axis, is a valuable tool for web analysts. This visualization helps reveal correlations between traffic spikes and specific events, such as marketing campaigns or product launches.
By plotting data points in this manner, analysts can easily identify patterns and anomalies in visitor behavior, allowing businesses to assess the effectiveness of their strategies and optimize future campaigns.
A time series decomposition plot that breaks down air quality index (AQI) data into its trend, seasonality, and residual components provides essential insights for public health officials.
By isolating these components over several years, stakeholders can better understand the underlying factors affecting air quality, such as seasonal variations or long-term trends. This understanding is crucial for developing effective policies and public health initiatives aimed at improving air quality and protecting community health.
A box plot displaying daily energy consumption across different months summarizes the distribution of energy usage effectively. By highlighting the median, quartiles, and potential outliers, this visualization allows energy managers to assess variability in consumption patterns.
Such insights can inform energy management strategies, enabling organizations to identify trends in usage and implement measures to optimize energy consumption during peak periods.
An area chart illustrating the cumulative number of COVID-19 cases over time in a specific region serves as a powerful visualization for understanding the pandemic's impact.
This chart provides a clear visual representation of the rise in cases, helping public health officials and the general public grasp the severity of the situation and the effectiveness of various interventions. By highlighting trends and fluctuations, the area chart aids in planning and response efforts during public health crises.
A streamgraph is a dynamic visualization that represents the changing popularity of different streaming services over time. This flowing, organic shape effectively illustrates how usage patterns for platforms like Netflix, Hulu, and Disney+ evolve, highlighting competitive trends and viewer preferences.
By visualizing this data, stakeholders can gain insights into market dynamics, consumer behavior, and the effectiveness of content strategies in the competitive streaming landscape.
A comprehensive dashboard that combines various time series visualizations, such as GDP growth, unemployment rates, and inflation rates, is invaluable for economists and policymakers. This multi-faceted view allows users to analyze how these indicators interact over time, providing deeper insights into economic conditions.
By visualizing this data together, stakeholders can make informed decisions regarding economic planning, policy formulation, and resource allocation based on the relationships and trends observed in the data.
Time series visualization plays a crucial role in data analysis and decision-making across various fields. Here are some key points highlighting its importance:
Time series visualization allows analysts to easily identify trends over time, whether upward, downward, or cyclical. By visually representing data points in a chronological sequence, stakeholders can quickly grasp the overall direction of the data.
This ability to detect trends is vital for forecasting future behavior, whether in financial markets, sales, or environmental data.
Many time series datasets exhibit seasonal patterns, where certain behaviors or values recur at regular intervals. Visualization techniques, such as line or area charts, help in illustrating these seasonal effects clearly.
By recognizing these patterns, businesses and researchers can plan for fluctuations, optimize inventory, and implement targeted marketing strategies during peak seasons.
Visualizing time series data enables users to spot anomalies or outliers effectively. Sudden spikes or drops in data can be easily identified through graphical representations, which can signal potential issues or opportunities.
For example, a sudden increase in website traffic might indicate a successful marketing campaign, while a sharp decline could suggest a problem that needs immediate attention.
Time series visualizations make it easier to compare different datasets over the same period. For example, businesses can compare sales figures across multiple products or regions, allowing for more informed decision-making.
By placing multiple time series on the same graph, stakeholders can evaluate relationships and performance relative to one another, gaining insights that need to be included in tabular data.
Visualizations communicate complex data in a more digestible format, making it easier for stakeholders, including non-technical audiences, to understand insights.
Effective visual representation of time series data can help convey the story behind the numbers, facilitating discussions and presentations. This clarity can lead to better decision-making at all levels of an organization.
Time series visualization lays the foundation for predictive analytics by illustrating historical patterns and trends. By understanding how data has behaved over time, analysts can apply forecasting models to predict future outcomes.
This capability is essential for various applications, such as demand forecasting, financial projections, and resource allocation.
Visualizing time series data allows analysts to explore the data interactively. Tools like dashboards and dynamic visualizations enable users to filter and drill down into specific periods or data subsets.
This exploratory approach can reveal hidden insights, correlations, or factors influencing the data, enhancing overall analysis.
In business and policy-making contexts, time series visualization aids strategic planning by providing a historical context for decision-making.
By visualizing past performance and trends, organizations can set realistic goals, allocate resources effectively, and anticipate challenges. This strategic insight is invaluable for long-term success.
Here are some common visualization techniques for time series data, each with its strengths and applications:
Line charts are the most popular and straightforward method for visualizing time series data. They plot individual data points along a continuous line, making it easy to identify trends and fluctuations over time.
Line charts are particularly effective for showing continuous data, such as stock prices, temperature changes, or sales figures, as they clearly convey the overall direction of the data.
Area charts are similar to line charts but fill the area beneath the line, emphasizing the volume of change over time. This visualization technique is useful for showing cumulative totals and highlighting the magnitude of trends.
Area charts can be particularly effective in representing total sales or resource usage over a specific period, allowing for quick visual assessments of growth.
Bar charts are useful for visualizing discrete time intervals, with bars representing values for specific periods. They can be either vertical or horizontal and are particularly effective for comparing different categories over time.
For instance, a bar chart can show monthly sales figures or yearly revenue, making it easy to identify peaks and troughs across different periods.
Heatmaps use color gradients to represent data values across two dimensions, typically with time on one axis and another variable on the other.
This visualization technique is effective for identifying patterns and trends in large datasets, such as website traffic by hour and day. Heatmaps provide a quick visual cue about the intensity of values, making it easier to spot trends over time.
Candlestick charts are widely used in financial markets to display price movements over time. Each "candlestick" provides information about the opening, closing, high, and low prices for specific time intervals.
This format helps traders analyze market behavior and identify potential trading opportunities by providing a clear picture of price fluctuations.
Scatter plots can be useful for visualizing the relationship between two variables over time, with one variable represented on the x-axis (time) and another on the y-axis.
This technique allows analysts to identify correlations and patterns in data. For example, a scatter plot can reveal how time relates to sales growth, helping to identify potential trends or anomalies.
Box plots summarize the distribution of data points over time by highlighting the median, quartiles, and outliers. This technique is useful for understanding the variability of data across different periods, such as monthly energy consumption or sales performance.
Box plots can provide insights into the spread and central tendency of data, making them valuable for identifying trends and anomalies.
These plots break down a time series into its components: trend, seasonality, and residuals. This decomposition helps analysts understand the underlying patterns in the data.
By visualizing these components separately, stakeholders can gain insights into how trends and seasonal effects influence the overall data, aiding in forecasting and analysis.
Streamgraphs are a variation of area charts that display data over time as a flowing, organic shape. They are particularly effective for visualizing changes in categories over time, showing how different segments rise and fall relative to one another.
This dynamic representation is useful for exploring trends in media consumption or demographic changes over time.
Combination charts overlay multiple visualization types, such as line and bar charts, to provide a comprehensive view of the data. This approach allows for easy comparisons between different datasets, such as total sales represented by bars alongside profit margins displayed as a line.
Combination charts enhance the viewer’s understanding of the relationships between different variables over time.
Time series visualization is an essential tool for analyzing data that changes over time. By employing various visualization techniques such as line charts, area charts, and heatmaps analysts can effectively communicate complex patterns and trends, making data more accessible and understandable.
These visualizations not only help identify key insights, such as seasonality and anomalies but also support predictive analytics and informed decision-making.
Copy and paste below code to page Head section
Time series data consists of observations collected sequentially over time, often at regular intervals. Examples include daily stock prices, monthly sales figures, and yearly temperature readings.
Time series visualization is important because it helps identify trends, seasonality, and anomalies in data. Visual representations make it easier to communicate insights, support predictive analytics, and facilitate informed decision-making.
Common techniques include line charts, area charts, bar charts, heat maps, candlestick charts, scatter plots, box plots, time series decomposition plots, streamgraphs, and combination charts. Each technique serves different purposes and can highlight specific aspects of the data.
Choosing the right visualization method depends on your data type, the insights you want to convey, and your audience. Consider what aspects of the data you want to emphasize trends, comparisons, distributions, or relationships and select the visualization that best communicates those insights.
Yes, you can visualize multiple time series on the same chart using combination charts or overlaying line charts. This allows for easy comparison and helps identify relationships between different datasets.
There are several tools available for time series visualization, including Tableau, Microsoft Power BI, Google Data Studio, D3.js, Plotly, and many others. The choice of tool often depends on user preferences, technical expertise, and specific project requirements.