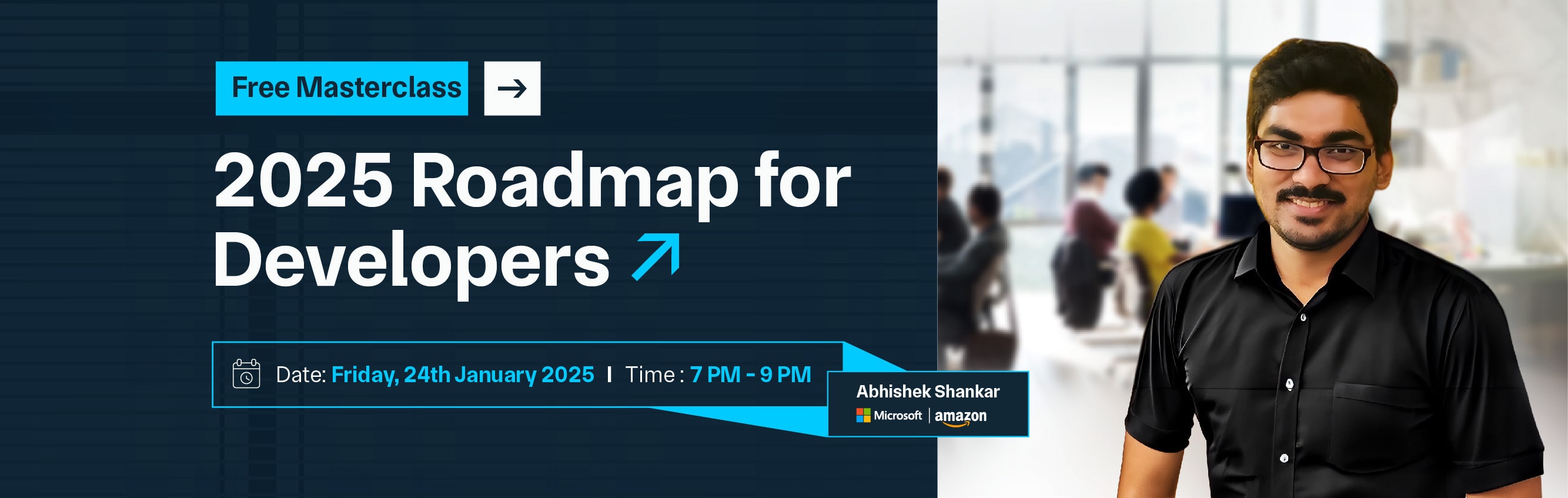

Linear regression is a fundamental statistical method used in data science to model the relationship between a dependent variable and one or more independent variables. The goal is to find a linear equation that best predicts the dependent variable based on the values of the independent variables. This is typically represented in the form Y=β0+β1X1+β2X2+...+βnXn+ϵY = \beta_0 + \beta_1X_1 + \beta_2X_2 + ... + \beta_nX_n + \epsilonY=β0+β1X1+β2X2+...+βnXn+ϵ, where YYY is the predicted value, β0\beta_0β0 is the intercept, β1,β2,...,βn\beta_1, \beta_2, ..., \beta_nβ1,β2,...,βn are the coefficients, X1,X2,...,XnX_1, X_2, ..., X_nX1,X2,...,Xn are the independent variables, and ϵ\epsilonϵ represents the error term.
The method relies on the least squares approach to minimize the sum of the squared differences between the observed values and the predicted values. Linear regression assumes a linear relationship between variables and is widely used due to its simplicity and interpretability.
It serves as a foundational tool for more complex models. It is applicable in various fields, including economics, biology, and social sciences, making it essential for predictive analytics and decision-making processes in data-driven environments.
Linear regression is a statistical method used to model the relationship between a dependent variable (often called the target or response variable) and one or more independent variables (predictors or features). Here are the basics:
1. Equation: The linear regression equation is typically expressed as:
Y=β0+β1X1+β2X2+...+βnXn+ϵY = \beta_0 + \beta_1X_1 + \beta_2X_2 + ... + \beta_nX_n + \epsilonY=β0+β1X1+β2X2+...+βnXn+ϵ
Here, YYY is the dependent variable, X1, X2,..., XnX_1, X_2, ..., X_nX1, X2,..., Xn are the independent variables, β0\beta_0β0 is the intercept, β1,β2,...,βn\beta_1, \beta_2, ..., \beta_nβ1,β2,...,βn are the coefficients (which represent the impact of each independent variable), and ϵ\epsilonϵ is the error term.
2. Assumptions: Linear regression relies on several assumptions:
3. Fitting the Model: The model is typically fitted using the least squares method, which minimizes the sum of the squared differences between observed and predicted values.
4. Interpretation: The coefficients indicate the direction and strength of the relationship between each independent variable and the dependent variable. A positive coefficient suggests a direct relationship, while a negative coefficient indicates an inverse relationship.
5. Evaluation: Common metrics for evaluating model performance include R-squared (which indicates how well the model explains the variability of the dependent variable), Mean Absolute Error (MAE), and Root Mean Squared Error (RMSE).
Linear regression is widely used due to its simplicity and effectiveness in many scenarios, making it a foundational tool in data analysis and predictive modeling.
The mathematical foundation of linear regression involves several key concepts and principles that form the basis for the model. Here’s a breakdown:
The linear regression model can be represented mathematically as:
Y=β0+β1X1+β2X2+...+βnXn+ϵY = \beta_0 + \beta_1X_1 + \beta_2X_2 + ... + \beta_nX_n + \epsilonY=β0+β1X1+β2X2+...+βnXn+ϵ
The goal of linear regression is to minimize the sum of squared residuals (the differences between observed and predicted values):
Minimize S=∑i=1n(Yi−Y^i)2\text{Minimize } S = \sum_{i=1}^{n} (Y_i - \hat{Y}_i)^2Minimize S=i=1∑n(Yi−Y^i)2
where Y^i\hat{Y}_iY^i is the predicted value from the linear model.
To find the coefficients (β\betaβ), we derive the normal equations by setting the gradient of the sum of squared residuals to zero. This leads to:
β^=(XTX)−1XTY\hat{\beta} = (X^TX)^{-1}X^TYβ^=(XTX)−1XTY
Where:
Linear regression relies on key assumptions:
Common metrics for assessing the model's performance include:
Linear regression can be categorized into several types based on the number of independent variables and the nature of the relationship being modeled. Here are the main types:
This involves a single independent variable used to predict a dependent variable. The relationship is modeled as follows:
Y=β0+β1X+ϵY = \beta_0 + \beta_1X + \epsilonY=β0+β1X+ϵ
Where YYY is the dependent variable, and XXX is the independent variable. It's suitable for understanding the effect of one predictor.
In this case, two or more independent variables are used to predict a dependent variable. The model is expressed as:
Y=β0+β1X1+β2X2+...+βnXn+ϵY = \beta_0 + \beta_1X_1 + \beta_2X_2 + ... + \beta_nX_n + \epsilonY=β0+β1X1+β2X2+...+βnXn+ϵ
This type allows for a more comprehensive analysis of how multiple factors influence the outcome.
When the relationship between the independent and dependent variables is non-linear, polynomial regression can be used. It incorporates polynomial terms of the independent variable(s):
Y=β0+β1X+β2X2+...+βnXn+ϵY = \beta_0 + \beta_1X + \beta_2X^2 + ... + \beta_nX^n + \epsilonY=β0+β1X+β2X2+...+βnXn+ϵ
This allows for modeling more complex relationships while still retaining a linear form in terms of coefficients.
A type of regularized regression that includes a penalty term to reduce model complexity and prevent overfitting. The objective function becomes:
Minimize S+λ∑j=1nβj2\text{Minimize } S + \lambda \sum_{j=1}^{n} \beta_j^2Minimize S+λj=1∑nβj2
Where λ\lambdaλ is the regularization parameter, ridge regression is particularly useful when multicollinearity exists among predictors.
Another form of regularized regression, Lasso (Least Absolute Shrinkage and Selection Operator), adds a penalty that can shrink some coefficients to zero, effectively performing variable selection:
Minimize S+λ∑j=1n∣βj∣\text{Minimize } S + \lambda \sum_{j=1}^{n} |\beta_j|Minimize S+λj=1∑n∣βj∣
This is useful when you want a simpler model with fewer predictors.
Combines the penalties of both Ridge and Lasso regression, allowing for a balance between the two:
Minimize S+λ1∑j=1n∣βj∣+λ2∑j=1nβj2\text{Minimize } S + \lambda_1 \sum_{j=1}^{n} |\beta_j| + \lambda_2 \sum_{j=1}^{n} \beta_j^2Minimize S+λ1j=1∑n∣βj∣+λ2j=1∑nβj2
Elastic Net is particularly effective in scenarios with highly correlated predictors.
Linear regression relies on several key assumptions to ensure the validity of the model and the reliability of the results. Here are the primary assumptions:
The relationship between the independent and dependent variables is linear. This means that a change in the predictor(s) will result in a proportional change in the response variable. This can be assessed using scatter plots or residual plots.
Observations are assumed to be independent of one another. This means that the value of the dependent variable for one observation does not influence the value for another. Violations can occur in time series data or clustered data.
The residuals (errors) of the model should have constant variance across all levels of the independent variables. If the variance of the residuals changes (i.e., heteroscedasticity), it can lead to inefficient estimates and affect hypothesis tests.
The residuals should be normally distributed, especially for small sample sizes. This assumption is important for conducting hypothesis tests about the coefficients. Normality can be checked using Q-Q plots or statistical tests like the Shapiro-Wilk test.
In multiple linear regression, the independent variables should not be highly correlated with each other. High multicollinearity can inflate the variances of the coefficient estimates, making them unstable and difficult to interpret. This can be assessed using variance inflation factor (VIF) values.
In time series data, the residuals should not be correlated with one another. Autocorrelation occurs when the residuals of one observation are correlated with those of another, which can violate the independence assumption. The Durbin-Watson test is often used to detect autocorrelation.
Implementing linear regression involves several key steps, from data preparation to model evaluation. Here’s a step-by-step guide using Python and the popular library scikit-learn.
First, you need to import the necessary libraries:
Import numpy as np
Import pandas as pd
import matplotlib.pyplot as plt
From sklearn.model_selection import train_test_split
from sklearn.linear_model import LinearRegression
From sklearn.metrics import mean_squared_error, r2_score
Load your dataset and prepare it for modeling. For this example, we’ll assume you have a CSV file.
# Load dataset
data = pd.read_csv('your_data.csv')
# Preview the data
print(data.head())
# Select independent and dependent variables
X = data[['feature1', 'feature2']] # Replace with your feature names
y = data['target'] # Replace with your target variable
Divide the dataset into training and testing sets to evaluate model performance.
X_train, X_test, y_train, y_test = train_test_split(X, y, test_size=0.2, random_state=42)
Instantiate and fit the linear regression model.
# Create a Linear Regression model
model = LinearRegression()
# Fit the model on training data
model.fit(X_train, y_train)
Use the model to make predictions on the test set.
# Predict on the test set
y_pred = model.predict(X_test)
Assess the model’s performance using metrics like Mean Squared Error (MSE) and R-squared.
# Calculate Mean Squared Error and R-squared
mse = mean_squared_error(y_test, y_pred)
r2 = r2_score(y_test, y_pred)
print(f'Mean Squared Error: {mse}')
print(f'R-squared: {r2}')
Plot the actual vs. predicted values to visualize performance.
plt.scatter(y_test, y_pred)
plt.xlabel('Actual Values')
plt.ylabel('Predicted Values')
plt.title('Actual vs Predicted')
plt.plot([y.min(), y.max()], [y.min(), y.max()], color='red', linestyle='--') # Line of equality
plt.show()
You can access the coefficients to understand the impact of each feature.
print('Intercept:', model.intercept_)
print('Coefficients:', model.coef_)
Interpreting the results of a linear regression model involves analyzing the model's output, including coefficients, metrics, and visualizations. Here’s a guide on how to do this effectively:
Examine the residuals (the differences between observed and predicted values) to check the assumptions of linear regression:
While linear regression is a powerful and widely used statistical method, it has several limitations that can impact its effectiveness and applicability. Here are some key limitations:
Linear regression assumes a linear relationship between the independent and dependent variables. If the true relationship is non-linear, the model may perform poorly. In such cases, polynomial regression or other non-linear models may be more appropriate.
Linear regression is sensitive to outliers, which can significantly skew results. A few extreme values can disproportionately affect the slope of the regression line, leading to misleading interpretations.
The model assumes that the observations are independent of each other. In cases of time series data or clustered data, this assumption may be violated, leading to inaccurate results.
In multiple linear regression, high correlation among independent variables (multicollinearity) can make it difficult to determine the individual effect of each predictor. This can inflate standard errors and lead to unreliable coefficient estimates.
Linear regression assumes that the variance of the residuals is constant across all levels of the independent variables (homoscedasticity). If this assumption is violated (heteroscedasticity), it can affect the validity of hypothesis tests and confidence intervals.
While the normality of residuals is not a strict requirement for predictions, it is important for hypothesis testing. If residuals are not normally distributed, it can lead to inaccurate inferences about the model coefficients.
Advanced techniques in linear regression and regression analysis allow for better modeling of complex data relationships and can improve predictive accuracy. Here are some of the key advanced techniques:
Polynomial regression extends linear regression by adding polynomial terms of the independent variables, allowing the model to capture non-linear relationships. For example:
Y=β0+β1X+β2X2+…+βnXn+ϵY = \beta_0 + \beta_1X + \beta_2X^2 + \ldots + \beta_nX^n + \epsilonY=β0+β1X+β2X2+…+βnXn+ϵ
This technique can be useful when the relationship between the predictor and the response variable is curved.
Regularization methods add a penalty to the loss function to prevent overfitting, especially in models with many predictors:
When the effect of one independent variable on the dependent variable depends on another independent variable, interaction terms can be included in the model:
Y=β0+β1X1+β2X2+β3(X1×X2)+ϵY = \beta_0 + \beta_1X_1 + \beta_2X_2 + \beta_3(X_1 \times X_2) + \epsilonY=β0+β1X1+β2X2+β3(X1×X2)+ϵ
This technique captures more complex relationships between predictors.
GLMs extend linear regression to accommodate different types of response variables. For example, logistic regression (a type of GLM) is used for binary outcomes, while Poisson regression is used for count data. The model takes the form:
g(Y)=β0+β1X1+…+βnXng(Y) = \beta_0 + \beta_1X_1 + \ldots + \beta_nX_ng(Y)=β0+β1X1+…+βnXn
Where G is a link function that relates the mean of the response to the linear predictor.
Quantile regression estimates the relationship between variables for different quantiles of the response variable rather than just the mean. This is particularly useful for understanding the impact of predictors across the distribution of the outcome variable.
Stepwise regression automatically selects the most significant variables to include in the model by adding or removing predictors based on specific criteria (like AIC or BIC). This helps in model simplification and can improve interpretability.
Using techniques like k-fold cross-validation helps assess the model’s predictive performance and reduces the risk of overfitting. The dataset is split into k subsets, and the model is trained and tested k times, each time using a different subset as the test set.
Bayesian approaches incorporate prior distributions for the coefficients and update these beliefs based on the observed data. This can provide a more comprehensive view of uncertainty in the model parameters.
Regression analysis is a cornerstone of data science, providing powerful tools for understanding relationships between variables and making predictions. Its simplicity and interpretability make it an essential starting point for many analytical tasks. By applying techniques like linear regression, data scientists can uncover insights, quantify relationships, and inform decision-making processes across various domains.
Despite its strengths, regression has limitations, such as assumptions of linearity, independence, and homoscedasticity. Advanced techniques like polynomial regression, regularization methods, and generalized linear models help address these limitations and improve model accuracy and interpretability. Additionally, incorporating practices like cross-validation ensures robust model evaluation and generalizability.
Copy and paste below code to page Head section
Regression analysis is a statistical method used to model and analyze the relationships between a dependent variable and one or more independent variables. It helps in predicting outcomes and understanding how different factors influence a particular response.
The most common types include: Linear Regression: Models a linear relationship between variables. Multiple Linear Regression: Involves two or more independent variables. Polynomial Regression: Models non-linear relationships using polynomial terms. Ridge and Lasso Regression: Regularized methods that help prevent overfitting. Logistic Regression: Used for binary outcome prediction.
Key assumptions include: Linearity: The relationship between predictors and the dependent variable is linear. Independence: Observations are independent of each other. Homoscedasticity: Constant variance of residuals. Normality: Residuals should be normally distributed.
Common evaluation metrics include: R-squared: Indicates the proportion of variance explained by the model. Mean Squared Error (MSE): Measures the average squared difference between actual and predicted values. Root Mean Squared Error (RMSE): The square root of MSE, providing error in the same units as the dependent variable.
Multicollinearity occurs when independent variables are highly correlated. It can make coefficient estimates unstable and increase standard errors, making it difficult to determine the individual effect of predictors.
Outliers can be addressed by: Transforming the data (e.g., using logarithmic transformations). Removing outliers if they are errors. Using robust regression techniques that are less sensitive to outliers.