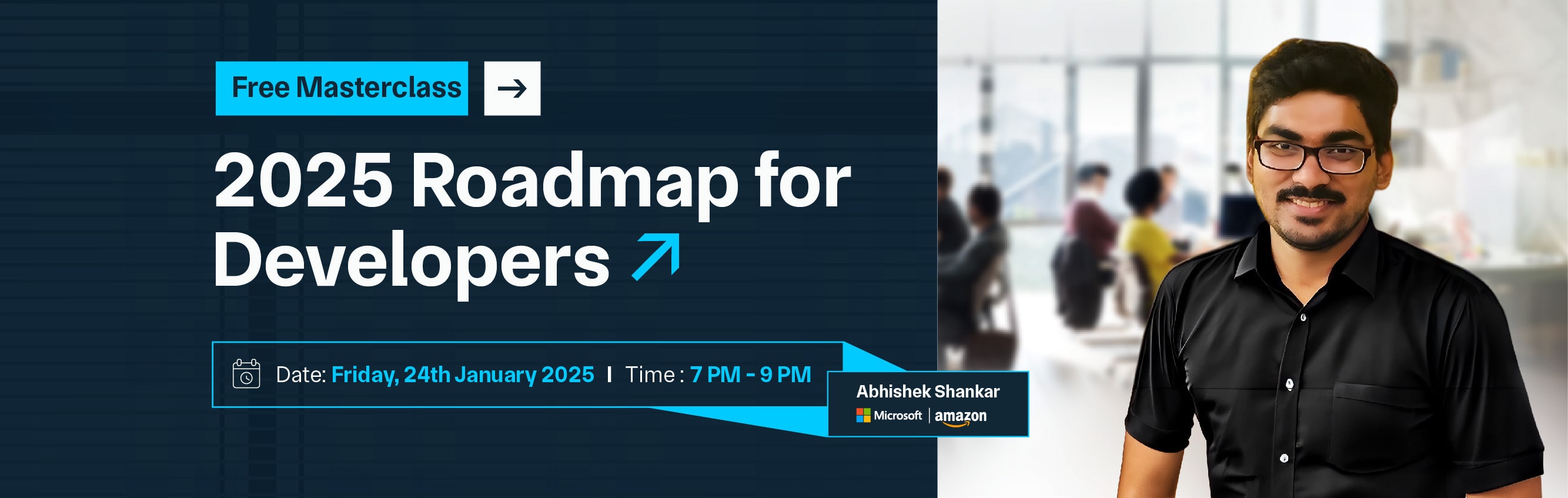

Statistics and Probability for Data Science form the foundation of data analysis, enabling data scientists to extract meaningful insights from raw data. Statistics involves the collection, analysis, interpretation, and presentation of data, helping to summarize large datasets and draw conclusions. Key concepts in statistics include measures of central tendency (mean, median, mode), measures of variability (variance, standard deviation), and inferential statistics, which help in making predictions or generalizations about a population based on sample data.
Techniques like hypothesis testing, confidence intervals, and regression analysis are commonly used to evaluate data and make data-driven decisions. Probability, on the other hand, is the study of uncertainty and the likelihood of events occurring. It provides a mathematical framework for modeling uncertainty and making predictions. Concepts like conditional probability, Bayes’ theorem, and probability distributions (such as normal, binomial, and Poisson distributions) are essential for building predictive models in data science.
Understanding probability allows data scientists to assess risks, quantify uncertainties, and build more accurate models, particularly in areas like machine learning and AI. Together, statistics and probability are indispensable tools in data science, helping professionals analyze data, identify patterns, and make informed predictions and decisions that drive business outcomes.
Data refers to raw facts, figures, or information that can be collected, analyzed, and interpreted to generate meaningful insights. It can take various forms, such as numbers, text, images, audio, or even sensor readings. On its own, data may only have much value once it is processed, analyzed, or organized in a way that makes it useful for decision-making or problem-solving.
There are two main types of data:
In the context of data science, data is the foundation for performing analyses, building predictive models, and deriving insights that can guide business decisions, improve processes, and drive innovation. Data can be collected from various sources, such as surveys, experiments, transactions, sensors, or social media, and it is often stored in databases or data warehouses for easy access and analysis.
Quantitative data refers to data that is expressed in numerical terms, allowing for mathematical calculations and statistical analysis.
1. Discrete Data: This type of data consists of distinct, countable values. Discrete data represents whole numbers.
2. Continuous Data: Continuous data can take any value within a range and is measurable with precision. It is typically represented in decimal or fractional form.
Qualitative data is non-numeric and describes qualities or characteristics. This type of data can be used to classify and categorize information.
1. Nominal Data: Nominal data consists of categories with no inherent order or ranking. It is used for labeling or naming things.
2. Ordinal Data: Ordinal data represents categories with a meaningful order or ranking, but the intervals between categories need to be more consistent.
Binary data is a special case of categorical data where there are only two possible categories, typically represented by two states.
Time series data refers to a sequence of data points collected or recorded at regular time intervals. It is used to analyze trends over time.
Textual data consists of words, sentences, or documents. It is often unstructured and requires techniques such as natural language processing (NLP) to analyze and extract insights.
Spatial data is information that has a geographic or spatial component. It represents the location or shape of objects in space.
Structured data refers to data that is organized in a predefined format, often in rows and columns, such as in relational databases. It is highly organized and easy to search and process.
Unstructured data does not have a predefined format and is often more difficult to analyze. It includes text, images, video, audio, and other non-tabular formats.
Semi-structured data contains elements of both structured and unstructured data. It doesn’t conform to the rigid structure of a database but has some organization, such as tags or markers, to separate elements.
Relational data refers to data that is stored in a relational database, where data is organized into tables that are related to each other through keys (such as primary and foreign keys). This type of data is often used in applications requiring structured relationships between datasets.
A database for an e-commerce store that links customer details to their orders through an Order ID
In statistics, the mean, median, and mode are fundamental measures of central tendency. These measures help describe the center of a dataset, summarizing a large amount of data into a single value that represents the "average" or central point. Let’s take a closer look at each:
The mean is the most commonly used measure of central tendency. It is calculated by adding up all the values in a dataset and then dividing by the number of values.
Mean=∑XNMean=N∑X
Suppose you have the following dataset: 2, 4, 6, 8, 10.
Mean=305=6Mean=530=6
So, the mean is 6.
When to use the Mean:
The mean is useful when the data is symmetrically distributed, and there are no extreme outliers, as outliers can significantly skew the mean.
The median is the middle value in a dataset when the values are ordered from smallest to largest (or vice versa). If there is an odd number of values, the median is the value at the center. If there is an even number of values, the median is the average of the two middle values.
Steps to Calculate the Median:
Dataset: 1, 3, 5, 7, 9
So, the median is 5.
Dataset: 1, 3, 5, 7
So, the median is 4.
The median is particularly useful when there are outliers, or the dataset is skewed. Unlike the mean, the median is not affected by extremely high or low values.
The mode is the value that appears most frequently in a dataset. A dataset can have one mode, more than one mode, or no mode at all.
Dataset: 2, 3, 4, 4, 5
Dataset: 1, 2, 2, 3, 3, 4
Dataset: 1, 2, 3, 4, 5
The mode is useful when you want to know the most frequent or popular item in a dataset. It’s often used for categorical data where the values represent categories, like voting preferences, product choices, or survey responses.
Variance and Standard Deviation are both measures of dispersion or spread in a dataset. They provide insights into how much the values in a dataset deviate from the mean. While they are closely related, they are presented in different forms, and each has its use case.
Variance is a statistical measure that describes the average squared deviation of each data point from the mean of the dataset. It gives an idea of how spread out the data is. A higher variance indicates that the data points are more spread out, while a lower variance indicates that the data points are closer to the mean.
Population Variance (for the entire population):
s2=1n−1∑i=1n(Xi−Xˉ)2
s2=n−11i=1∑n(Xi−Xˉ)2
Consider the dataset: 2, 4, 6, 8, 10
So, the variance is 8 (for the population variance).
Variance is particularly useful in assessing the overall spread of a dataset. However, because the units of variance are the square of the units of the original data (for example, if the data is in meters, the variance is in square meters), it can be difficult to interpret directly in real-world terms.
The standard deviation is simply the square root of the variance. It measures the average amount by which each data point in a set differs from the mean. Since the standard deviation is in the same units as the original data, it is often more interpretable than variance.
Population Standard Deviation:
Sample Standard Deviation:
From the previous calculation, the variance was 8. Now, to find the standard deviation:
Standard Deviation=8≈2.83
Standard Deviation=
8
≈2.83
So, the standard deviation of this dataset is approximately 2.83.
Here’s a table comparing Population Data and Sample Data:
Probability is a branch of mathematics that deals with the likelihood or chance of an event occurring. It provides a quantitative description of the likelihood of various outcomes in uncertain situations. Probability is used to model random events and is foundational to fields like statistics, finance, science, and many areas of decision-making.
1. Event:
2. Sample Space (S):
3. Outcome:
4. Probability of an Event (P):
5. Complementary Events:
6. Independent Events:
7. Dependent Events:
1. Classical Probability:
2. Empirical (Experimental) Probability:
3. Subjective Probability:
Conditional probability is the probability of an event occurring, given that another event has already occurred. It answers the question: What is the probability of an event.
A
A happening given that event
B
B has occurred?
The notation for conditional probability is
P(A∣B)
P(A∣B), which reads as "the probability of
A
A given
B
B". This concept is essential in various fields like statistics, machine learning, and decision-making, as it allows us to refine predictions based on new information.
The conditional probability of an event
A
An occurrence is given that the event
B
B has already occurred, is given by the formula:
P(A∣B)=P(A∩B)P(B)
P(A∣B)=
P(B)
P(A∩B)
Note: This formula is valid only if
P(B)>0
P(B)>0 because the denominator cannot be zero.
To understand conditional probability more intuitively, let's consider an example:
Suppose you have a standard deck of 52 playing cards, and you want to find the probability of drawing a King given that you have already drawn a Heart.
The intersection of events
The probability of drawing any Heart (13 hearts in total) is:
So, the probability of drawing a King, given that the card is a Heart, is
113
13
1
.
Bayes' Theorem is a fundamental concept in probability theory that describes how to update the probability of a hypothesis (or event) based on new evidence. It provides a way to calculate conditional probabilities by relating prior knowledge with the likelihood of observed data.
Mathematically, Bayes’ Theorem is expressed as:
P(A∣B)=P(B∣A)⋅P(A)P(B)
P(A∣B)=
P(B)
P(B∣A)⋅P(A)
Statistics and probability are essential in data science, enabling professionals to analyze and interpret data effectively. Statistics provides tools for summarizing data, making inferences, and testing hypotheses, while probability models uncertainty and helps in predicting outcomes. Together, they empower data scientists to make data-driven decisions and solve complex problems.
Copy and paste below code to page Head section
Statistics helps data scientists analyze, summarize, and draw insights from data. It provides methods for making inferences about populations based on sample data, testing hypotheses, and evaluating model performance.
Probability models uncertainty and randomness, helping data scientists predict outcomes, estimate risks, and build predictive models. It’s crucial in machine learning, decision-making, and understanding data distributions.
Descriptive statistics summarize and describe data (e.g., mean, variance), while inferential statistics use sample data to make predictions or generalizations about a population (e.g., hypothesis testing, confidence intervals).
Bayes' Theorem is a method for updating the probability of a hypothesis based on new evidence. It is widely used in machine learning, particularly in classifiers like Naive Bayes, and for probabilistic decision-making.
Probability helps in building models that can predict outcomes based on uncertain or incomplete data. It’s essential for techniques like regression, classification, and anomaly detection, allowing models to handle uncertainty and make informed predictions.
Hypothesis testing involves evaluating two competing hypotheses using sample data to determine if there is enough evidence to support a specific claim about a population. It helps make decisions or inferences from data.