
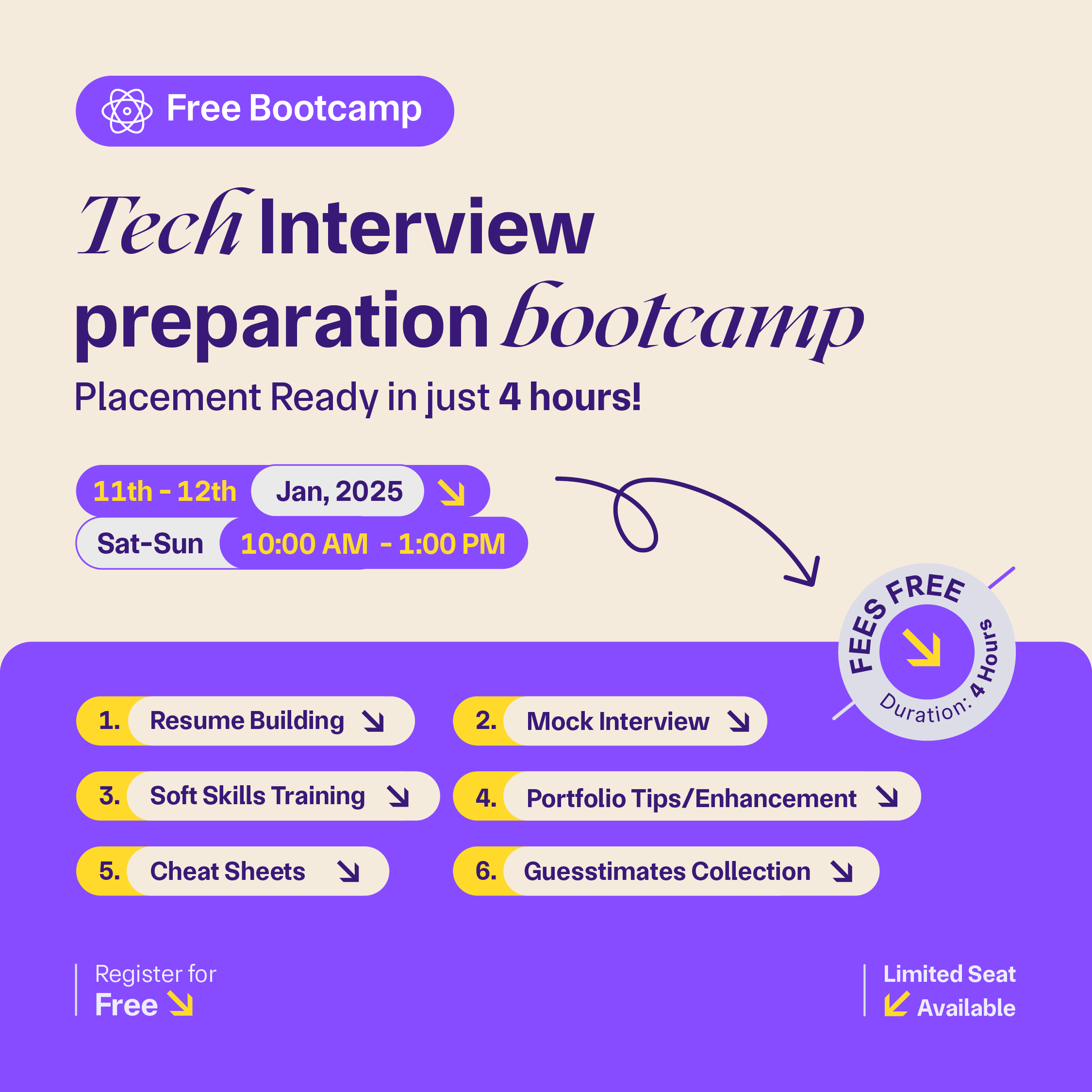
Linear algebra is a fundamental area of mathematics that plays a crucial role in data science. It provides the theoretical foundation for many techniques used in machine learning, artificial intelligence, and statistical modeling. At the core of linear algebra are concepts like vectors, matrices, and operations such as matrix multiplication, addition, and inversion. These operations are essential for manipulating and transforming data.
In data science, vectors are used to represent data points in high-dimensional space. In contrast, matrices are used to represent datasets where rows correspond to individual observations and columns correspond to features. Many machine learning algorithms, such as linear regression, support vector machines, and neural networks, rely heavily on matrix operations for tasks like optimization and solving systems of equations.
Eigenvalues and eigenvectors, also important concepts in linear algebra, help in dimensionality reduction techniques like Principal Component Analysis (PCA), which reduces the number of features in a dataset while retaining the most important information. Singular Value Decomposition (SVD) is another technique rooted in linear algebra that is widely used in data science for tasks such as collaborative filtering in recommendation systems. Overall, a solid understanding of linear algebra is essential for data scientists to efficiently process, analyze, and model large datasets.
Linear algebra is a cornerstone of data science, providing the mathematical tools necessary for working with large datasets and building machine learning models. In data science, data is often represented as vectors, matrices, and higher-dimensional tensors. Linear algebra enables the manipulation of these structures efficiently, which is vital for various data processing tasks.
Key concepts in linear algebra used in data science include:
Linear algebra is fundamental for efficiently analyzing, modeling, and understanding data in data science, making it a key area of study for anyone in the field.
Linear algebra is essential in data science due to its ability to handle large datasets and solve complex problems efficiently. It provides the mathematical foundation for many algorithms and techniques used in machine learning, statistical analysis, and data processing. Here's why it's so important:
Linear algebra is foundational to data science, enabling the efficient analysis, transformation, and modeling of data, and is essential for developing and deploying machine learning algorithms.
The following table outlines key concepts in Linear Algebra and their applications in Data Science. These fundamental concepts provide the mathematical tools for representing, transforming, and analyzing data.
Understanding these concepts is essential for tasks such as machine learning, optimization, data preprocessing, and dimensionality reduction. The table highlights each concept's definition and its practical use in real-world data science applications.
Linear algebra plays a crucial role in data science by providing the mathematical foundation for representing and manipulating data. Key applications include:
Linear algebra is fundamental to processing, analyzing, and solving complex data science problems efficiently.
In data science, advanced linear algebra techniques are often employed to handle large, complex datasets, optimize models, and extract meaningful insights.
These techniques extend the fundamental concepts of linear algebra and are key to tackling challenging problems in machine learning, optimization, and data analysis. Here are some of the most important advanced techniques:
SVD is a powerful matrix factorization technique that decomposes a matrix AAA into three matrices: A=UΣVTA = U \Sigma V^TA=UΣVT, where:
Eigenvalues and eigenvectors are key concepts in linear algebra used in various data science techniques. They are especially useful in:
Matrix factorization techniques like LU decomposition, QR decomposition, and Cholesky decomposition are used to decompose a matrix into simpler components to solve linear systems and optimize computations. These methods are frequently used in machine learning algorithms:
NMF is a variant of matrix factorization where the factorized matrices are constrained to have non-negative entries. This technique is useful when the data is non-negative (e.g., word counts, pixel intensities). NMF is widely used in text mining, image processing, and topic modeling.
Tensors are multi-dimensional arrays and tensor decomposition generalizes matrix factorization to higher dimensions. Techniques like CANDECOMP/PARAFAC and Tucker decomposition decompose multi-way data into components.
This makes it useful for analyzing complex, multi-dimensional data, such as in multi-modal machine learning problems or high-dimensional time series analysis.
Advanced linear algebra techniques are often used in optimization problems, which are central to machine learning. Some key techniques include:
Markov Chains model processes that move between states in a probabilistic manner. Linear algebra techniques like matrix powers and eigenvalues are used to analyze the long-term behavior of Markov Chains. Transition matrices, which describe the probabilities of moving between states, are manipulated using matrix multiplication.
In big data scenarios, matrix multiplication can become computationally expensive. Techniques such as Strassen’s algorithm and Coppersmith-Winograd's algorithm offer faster methods of matrix multiplication, which can speed up computations in data science, particularly when working with large datasets or deep learning models.
Graph-based techniques use linear algebra to represent and analyze networks. The Laplacian matrix of a graph is used in spectral clustering, community detection, and graph partitioning. Eigenvectors of the Laplacian matrix help find clusters or communities in graphs, which are useful in social network analysis and recommendation systems.
Randomized methods provide an efficient way to perform linear algebra computations, particularly for large-scale problems where exact methods would be computationally expensive. Techniques like randomized SVD and random projections can be used to approximate large matrices and speed up computations in big data contexts.
These methods are used to solve large linear systems of equations, especially when the matrix is sparse. Krylov subspace methods, such as GMRES and Conjugate Gradient, use iterative approaches to approximate solutions to systems of equations, which is valuable in machine learning optimization problems.
In linear algebra, various problems can be represented using mathematical objects such as vectors, matrices, and systems of linear equations. These representations form the foundation for solving real-world problems in areas like data science, engineering, and physics. Below are key types of problems and their representations in linear algebra:
One of the most fundamental problems in linear algebra is solving systems of linear equations. A system of equations can be represented in matrix form as:
Ax=bAx = bAx=b
Where:
This matrix equation can be solved using methods like Gaussian elimination, LU decomposition, or matrix inversion (if AAA is invertible). Solving such systems is a key task in linear algebra, with applications in optimization, physics, and machine learning.
Linear algebra is used to represent and solve problems involving matrix operations such as addition, multiplication, and inversion. Each operation corresponds to a different transformation of data. For instance:
These matrix operations are crucial for various applications in computer graphics, machine learning, and data transformation.
Eigenvalues and eigenvectors are used to represent problems where linear transformations act on vectors in a way that only stretches or shrinks them without changing their direction. The equation for eigenvalues and eigenvectors is:
Av=λvA v = \lambda vAv=λv
Where:
This concept is particularly useful in principal component analysis (PCA) for dimensionality reduction, where eigenvectors represent directions of maximum variance in the data.
Principal Component Analysis (PCA) is an application of linear algebra to reduce the dimensionality of large datasets while preserving the most important information. In PCA, data points are projected onto a new set of axes (principal components) that are eigenvectors of the covariance matrix of the data:
X=W⋅ZX = W \cdot ZX=W⋅Z
Where:
This transformation helps in visualizing high-dimensional data and reducing computational complexity in machine learning algorithms.
In regression problems, particularly when data is overdetermined (more equations than unknowns), the least squares method is used to find the best-fitting solution. The problem can be represented as:
minx∥Ax−b∥22\min_x \|Ax - b\|_2^2xmin∥Ax−b∥22
Where:
The solution is obtained by minimizing the squared error between the predicted and actual values, which can be solved using matrix operations.
SVD decomposes any matrix AAA into three components: A=UΣVTA = U \Sigma V^TA=UΣVT. This decomposition is used to solve problems involving low-rank approximations, noise reduction, and dimensionality reduction. In data science, SVD is frequently used in recommendation systems and for efficiently solving linear systems.
Optimization problems often require minimizing or maximizing a function subject to certain constraints. These problems can often be formulated using linear algebra. For example, in linear programming (LP), the goal is to optimize a linear objective function subject to linear constraints:
Maximize subject toAx≤b\text{Maximize } c^T x \quad \text{subject to} \quad Ax \leq maximize subject toAx≤b
Where:
Linear algebra methods such as the Simplex method are used to solve LP problems.
Graphs are represented using adjacency matrices or Laplacian matrices, where the structure of the graph (nodes and edges) is encoded in a matrix format.
The Laplacian matrix L of a graph is defined as L=D−AL = D - AL=D−A, where DDD is the degree matrix (diagonal matrix with node degrees), and AAA is the adjacency matrix. This matrix is used in spectral clustering and community detection problems in network analysis.
Markov chains are modeled using transition matrices. These matrices represent the probabilities of moving from one state to another. For example, the transition matrix PPP for a Markov chain can be used to predict the state of the system at time t+1t+1t+1 from its state at the time it as:
xt+1=P⋅xt\mathbf{x}_{t+1} = P \cdot \mathbf{x}_txt+1=P⋅xt
Where:
Markov chains are used in various applications like page rank algorithms and modeling stochastic processes.
Linear algebra is fundamental to data science, serving as the backbone for numerous algorithms and techniques used in data analysis, machine learning, and statistical modeling. Here’s a detailed explanation of how linear algebra is applied in various aspects of data science:
In data science, data is often represented in vector and matrix formats:
Linear algebra enables efficient manipulation of these representations through operations like matrix multiplication, addition, and inversion.
Linear algebra is crucial for developing and understanding machine learning models, especially for regression, classification, and neural networks:
Linear algebra is key to dimensionality reduction techniques, which are used to simplify high-dimensional data while preserving important patterns:
Optimization problems, which are central to many machine learning algorithms, heavily rely on linear algebra:
In collaborative filtering and recommender systems, linear algebra plays a vital role in making predictions about items a user might like based on their previous behavior:
In NLP, text data is converted into numerical representations (such as word embeddings) using linear algebra:
Graph data can be represented using adjacency matrices or Laplacian matrices, which are manipulated through linear algebra:
Images are often represented as matrices, where pixel values are organized into a grid. Linear algebra techniques are used for image manipulation and transformation:
Markov chains, used for modeling stochastic processes, rely on transition matrices. Linear algebra helps analyze the long-term behavior of Markov processes:
Linear algebra enables efficient computation with large datasets, often represented as sparse matrices where most of the elements are zero:
Linear algebra is an essential mathematical foundation for data science, providing the tools and techniques needed to analyze and interpret data effectively. Its applications are wide-ranging, from representing and transforming data using vectors and matrices to solving complex problems in machine learning, optimization, and dimensionality reduction. Key areas such as machine learning algorithms, dimensionality reduction techniques (like PCA and SVD), and data representation rely heavily on linear algebra concepts to process and manipulate large datasets efficiently.
By enabling tasks like solving systems of linear equations, optimizing models, and reducing the complexity of high-dimensional data, linear algebra empowers data scientists to create more accurate and scalable models. Whether it's in recommendation systems, image processing, natural language processing, or network analysis, linear algebra's role in handling and processing large amounts of data is undeniable.
Copy and paste below code to page Head section
Linear algebra is a branch of mathematics concerned with vector spaces, linear transformations, and systems of linear equations. It is critical in data science because it provides the tools to represent, manipulate, and analyze data efficiently. Vectors and matrices are used to represent data, and operations on these structures (like matrix multiplication, inversion, and eigenvalues) are foundational to many algorithms used in machine learning, optimization, and data analysis.
Some key concepts in linear algebra used in data science include: Vectors: One-dimensional arrays that represent data points or features. Matrices: Two-dimensional arrays used to represent datasets, systems of equations, or transformations. Matrix Multiplication: Essential for combining transformations and applying models. Eigenvalues and Eigenvectors: Used in dimensionality reduction techniques like PCA. Determinants and Inverses: Used in solving systems of equations and model optimization.
Linear algebra is fundamental in machine learning for tasks such as: Model Representation: Data, features, and predictions are often represented as vectors or matrices. Linear Regression: Solving linear systems to estimate the parameters of a regression model. Optimization: Gradient descent, which updates model parameters, relies heavily on vector calculus and matrix operations. Principal Component Analysis (PCA): A linear algebra technique used for dimensionality reduction by finding eigenvectors of the covariance matrix.
Matrices are used in data science to represent datasets, transformations, and systems of linear equations. Each row in a matrix might represent a data point, and each column represents a feature. Operations like matrix multiplication, inversion, and decomposition help in transforming the data, solving optimization problems, and making predictions.
SVD is a matrix factorization technique that decomposes a matrix into three components: U, Σ\SigmaΣ, and VTV^TVT. It is widely used for: Dimensionality Reduction: Reducing the number of variables in large datasets while retaining the most important features. Noise Reduction: SVD can be used to filter out noise from data, especially in applications like image compression and collaborative filtering (e.g., recommendation systems). Latent Factor Models: Decomposing user-item matrices to make predictions, commonly used in recommender systems.
Eigenvalues and eigenvectors are fundamental concepts used in various data science applications. Eigenvectors represent directions along which a linear transformation acts by stretching or compressing. In PCA, eigenvectors represent the directions of maximum variance in data, and the corresponding eigenvalues indicate the magnitude of variance along those directions. This allows for dimensionality reduction by focusing on components with the highest eigenvalues.