
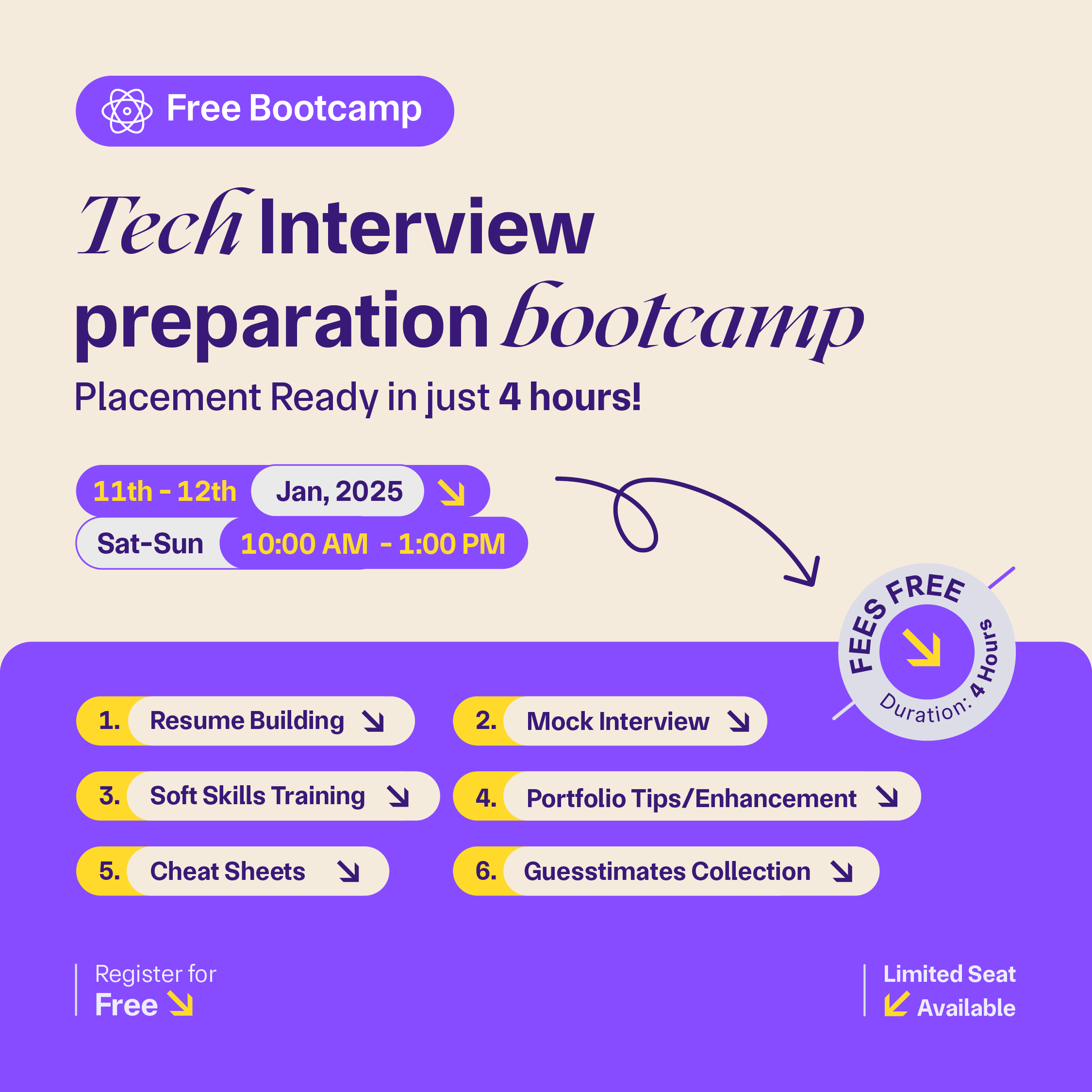
Generative AI can't make decisions or understand complex situations like humans can. One key limitation of current generative AI applications is their inability to understand the context and exhibit genuine creativity or intuition. While AI models, such as language models or image generators, can produce impressive outputs based on patterns they have learned from vast datasets, they need more true comprehension. These models do not "understand" the meaning behind their creations in the way humans do. Generative AI can't make decisions or understand complex situations like humans can.
For instance, when generating text or art, AI relies on statistical correlations and patterns, but it cannot grasp the deeper intent or emotional resonance behind a specific request. Moreover, generative AI applications need help with tasks requiring reasoning, common sense, or long-term planning. While they can simulate these capabilities to some extent, they cannot consistently apply context in the same way a human would.
This results in occasional inaccuracies or outputs that seem disjointed or irrelevant, especially when dealing with complex, nuanced scenarios. While AI can mimic creativity or knowledge, it needs to have true awareness or the capacity for human-like innovation and judgment, which remains a fundamental gap in current generative AI technology. This is the critical area where human intelligence continues to outperform machines.
Generative AI refers to a class of artificial intelligence algorithms that are designed to create new content, such as text, images, music, or even code, by learning from existing data. Unlike traditional AI models that focus on recognizing patterns or making predictions, generative AI models can generate entirely new, original outputs. These models are trained on large datasets and use statistical methods to understand the underlying structures and relationships within the data. Generative AI works primarily through machine learning techniques, particularly deep learning and neural networks.
One of the most popular approaches is using Generative Adversarial Networks (GANs) or transformer-based models like OpenAI’s GPT (for text) or DALL·E (for images). GANs consist of two components: a generator, which creates new content, and a discriminator, which evaluates the content for authenticity. Through iterative training, the generator learns to produce content that becomes increasingly realistic while the discriminator gets better at distinguishing fake from real.
In the case of transformer-based models, they are trained on vast amounts of text data and use attention mechanisms to generate contextually relevant and coherent responses. The model learns to predict the next word or token in a sequence based on the previous ones, allowing it to generate creative and context-aware text. Overall, generative AI works by learning from patterns in data and using that knowledge to produce new, original content, making it a powerful tool in fields like art, entertainment, and business automation.
No, generative AI cannot think like humans. While generative AI has made impressive strides in producing text, images, music, and even code, it operates fundamentally differently from human thought processes.
AI systems are based on algorithms that process and generate content based on patterns and correlations learned from large datasets. Here are a few key reasons why AI cannot think like humans:
Human thought involves consciousness the awareness of one's existence and the ability to reflect on one's thoughts, emotions, and experiences. AI, on the other hand, lacks self-awareness. It doesn't "think" or have subjective experiences.
It follows programmed rules and statistical patterns from the data it has been trained on, but this is not the same as human thought, which involves introspection, emotions, and complex reasoning.
Humans often rely on emotions, intuition, and empathy in their thinking, which influence decision-making, creativity, and relationships. AI does not have emotions.
While it can simulate empathy (for instance, by generating text that seems empathetic), it doesn’t actually "feel" anything. It simply recognizes patterns in data and mimics behaviors based on those patterns without any emotional context or understanding.
AI can generate creative outputs such as art, music, or writing but this creativity is derived from patterns in data rather than independent thought.
While AI might appear creative by combining existing ideas in novel ways, it does not have any other original thought or intuition that humans use when creating something truly new. Human creativity is influenced by life experiences, emotions, and abstract thinking, areas where AI falls short.
Human thinking involves deep reasoning and critical thinking, particularly in situations that require evaluating ethical dilemmas, long-term consequences, or abstract concepts.
AI, in contrast, operates primarily through pattern recognition and optimization based on the data it has. It can simulate reasoning within the scope of its training, but when faced with new or ambiguous situations, AI may struggle to respond appropriately.
AI generates outputs based on data, but it does not truly "understand" the context in which it operates. For example, while an AI language model might generate a sentence that appears contextually appropriate, it needs to comprehend the subtleties, emotions, or social dynamics behind the words. Human thinking involves understanding context, culture, and emotions, which AI needs to improve.
Generative AI has gained widespread attention for its ability to create original content such as text, images, and music. While it offers significant potential, there often needs to be more clarity between the hype and reality of its capabilities. Below is a comparison between the hype surrounding generative AI and the reality of its current limitations.
One of the primary limitations of current generative AI models is their need for deeper understanding. Despite their ability to generate text, images, and other content that may seem highly sophisticated, AI models do not truly "understand" the information in the way humans do. They operate based on patterns and correlations learned from large datasets rather than any real comprehension or contextual insight. For example, a model like GPT-4 can generate coherent text, but it needs an understanding of the concepts or emotions behind the words.
It predicts the next word in a sequence based on probabilities without grasping the deeper meaning of the sentence or the broader context in which it is used. This limitation results in AI being able to mimic creativity or intelligence but not truly possess it. This lack of deep understanding is evident in the AI’s inability to reason, make ethical judgments, or comprehend abstract concepts in the same way a human would.
Moreover, AI can struggle with tasks that require intuition or a nuanced grasp of complex situations. As a result, generative AI often produces content that might be technically accurate or plausible on the surface but can lack the subtleties and nuances that come from genuine human understanding. Ultimately, this gap in comprehension is a significant challenge for advancing AI to the point where it can truly replace human thought processes or achieve true creativity.
No, current AI systems cannot develop ideas from their "mind" because they do not have minds or consciousness in the way humans do. AI lacks the cognitive faculties, self-awareness, and intrinsic understanding that are essential for creative interpretation and generating truly novel ideas. AI, including generative models, operates by recognizing patterns and relationships in large datasets. It generates outputs based on statistical probabilities rather than a deep, subjective experience or personal insight.
Human creativity stems from a combination of life experiences, emotions, intuition, and a capacity for abstract thinking, all of which AI lacks. When humans create, they draw from complex mental processes, including memory, reasoning, and emotional resonance. In contrast, AI systems like GPT or DALL·E analyze vast amounts of data to generate content, but they do not "experience" or "understand" the content they produce.
Their outputs are purely derivative, relying on the patterns and information they have been trained on rather than any internalized creative thought. While AI can assist in creative tasks such as generating art, writing, or music it does so by mimicking human creativity, not originating it from an internal "mind" or genuine creative process. In other words, AI can simulate creativity but cannot truly innovate or come up with original ideas that arise from personal experiences or emotional depth.
AI’s ability to understand even the most complex situations is still limited. While current AI models, particularly those based on machine learning and deep learning, can process vast amounts of data and identify patterns, they do not truly "understand" situations in the way humans do. AI can recognize correlations, predict outcomes, and simulate intelligent behavior based on the data it has been trained on. Still, it lacks true comprehension, intuition, and the ability to reason through ambiguous or novel situations.
In complex scenarios, such as understanding human emotions, navigating ethical dilemmas, or making decisions with long-term consequences, AI often struggles. For example, while AI can analyze medical data to suggest potential diagnoses, it cannot grasp the nuanced human context of a patient’s experience, such as emotional factors, lifestyle, or personal values. Similarly, in legal or ethical contexts, AI may follow predefined rules or patterns, but it cannot navigate moral ambiguity or provide context-aware judgments the way a human can.
AI’s understanding is confined to the data and instructions it has received, and it cannot extrapolate knowledge or adapt to new situations without being retrained or reprogrammed. In contrast, humans can draw upon intuition, prior experiences, and emotional intelligence to make decisions in complex, unforeseen circumstances. Until AI can achieve a level of general intelligence capable of human-like reasoning, its understanding will remain constrained to patterns and information within its training scope.
AI bias and ethical concerns are significant challenges surrounding the development and deployment of AI technologies. These issues arise primarily due to the data-driven nature of AI systems and the ways in which they are trained and utilized.
AI systems learn from large datasets, and if these datasets contain biases whether due to historical inequalities, societal stereotypes, or unbalanced representation—the AI models can perpetuate and even amplify these biases. For example, facial recognition software has been found to have higher error rates for people with darker skin tones, largely due to underrepresentation in the training data. Similarly, natural language processing models like GPT may generate text that reflects gender, racial, or cultural biases present in the data they were trained on.
These biases can lead to unfair or discriminatory outcomes, particularly in sensitive areas like hiring, criminal justice, lending, or healthcare, where biased algorithms might impact people's lives and opportunities. The lack of diversity in AI training data, combined with the difficulty in detecting and mitigating biases, makes AI systems prone to reinforcing existing societal prejudices.
AI raises several ethical concerns, particularly regarding privacy, accountability, and the potential for misuse. For example:
To address AI bias and ethical concerns, developers, policymakers, and researchers are working on improving transparency, fairness, and accountability in AI systems. This includes diversifying training data, developing algorithms to detect and mitigate biases, creating robust regulations around data privacy and AI usage, and ensuring that AI development aligns with human values and societal goals.
However, while progress is being made, the ethical and social implications of AI remain a complex and ongoing challenge, requiring continuous attention as the technology evolves.
The challenge of moral judgments and affective intelligence in AI is one of the most complex and debated aspects of the technology. While AI can perform sophisticated tasks in data analysis, decision-making, and even creative generation, it cannot fundamentally make moral or ethical judgments in the way humans do.
This is due to several reasons, primarily the absence of emotions, intuition, and deep contextual understanding, all of which are central to human moral reasoning.
Moral decisions often involve navigating complex ethical dilemmas, where there are no clear right or wrong answers, and outcomes may depend on values, empathy, and long-term consequences. For example, in healthcare, AI might be tasked with determining the best treatment plan for a patient. Still, it cannot consider the patient's emotional state, personal values, or family dynamics in the same way a human doctor can. Similarly, in autonomous vehicles, decisions about how to react in a crash scenario involve making morally complex trade-offs (e.g., who to prioritize in an accident).
These types of moral dilemmas require judgment calls based on human values and ethics, which AI systems, no matter how advanced, cannot fully grasp or execute. AI systems typically operate on algorithms and rules derived from historical data, but these rules lack the nuance that moral decisions often require. Additionally, AI cannot experience human emotions, which are central to moral judgment, as empathy, compassion, and understanding of others' suffering are often essential to making ethically sound decisions.
Affective intelligence refers to the ability to understand and respond to emotions. For humans, emotions play a critical role in decision-making, interpersonal relationships, and moral judgment. While AI can be programmed to recognize and simulate emotional cues—such as tone of voice or facial expressions, it does not actually "feel" emotions.
This makes it difficult for AI to understand the emotional nuances of a situation or respond in a truly empathetic or emotionally intelligent way. For example, an AI chatbot may be able to identify that a user is upset based on their language. Still, it cannot genuinely empathize or offer a response that comes from an emotional understanding of the user's experience.
The inability of AI to make moral judgments or possess affective intelligence has significant implications, especially in fields where human emotion and ethical decision-making are vital. In areas like healthcare, criminal justice, or customer service, the lack of moral reasoning and empathy in AI could lead to decisions that are cold, impersonal, or even harmful. Moreover, there is a risk of over-reliance on AI for complex, morally sensitive tasks without adequate human oversight.
As AI continues to evolve, there is growing interest in integrating ethics and empathy into AI design. However, even with advances in these areas, AI will only partially replicate the depth of human moral reasoning or emotional intelligence. For the foreseeable future, human involvement and oversight will remain crucial in decision-making processes where ethics and emotions are key factors.
The future of generative AI holds immense potential, but overcoming its current limitations will be crucial to realizing its broader applications and addressing concerns. At the same time, generative AI has made significant strides in producing realistic text, images, music, and even code.
However, its limitations, such as lack of deep understanding, bias, and the inability to make moral or emotional judgments, still present major challenges. Overcoming these barriers will require advances in several areas of AI research, development, and ethical consideration.
One of the key hurdles is the AI’s inability to understand the context of complex situations truly. To overcome this, future generative AI systems will need to incorporate more advanced contextual awareness and reasoning capabilities. This could involve improving training techniques that help models develop a deeper understanding of cause and effect, abstract concepts, and relationships beyond simple data patterns.
Integrating multi-modal learning, where AI processes and understands various forms of data (text, audio, images) in context, might also improve its ability to interpret complex situations more accurately.
AI systems are often criticized for reflecting societal biases found in the training data. To reduce these biases, the development of more diverse and representative datasets is essential.
Furthermore, AI models will need improved bias-detection algorithms to ensure that the output does not perpetuate harmful stereotypes or unfair practices. Research is underway to create AI systems that can self-correct and adjust for bias, making AI outputs more equitable and just.
As generative AI is deployed in fields like healthcare, finance, and law, it must be able to make ethical decisions or at least provide recommendations that align with human values. The future of generative AI will likely include more advanced algorithms that consider ethical frameworks and human values in decision-making processes.
Collaborative efforts between ethicists, AI developers, and lawmakers will be crucial in creating guidelines and regulations that help AI systems navigate morally complex situations.
While AI currently lacks emotional intelligence, future advancements could lead to emotion-aware AI that can better respond to human feelings. Although AI will never "feel" emotions, it could become better at recognizing and responding to emotional cues, such as tone, body language, and context, in a more human-like way.
This could enhance AI's role in customer service, healthcare, and mental health applications, where empathy and emotional connection are critical.
Looking further into the future, the development of Artificial General Intelligence (AGI) AI that can perform any intellectual task a human can could radically transform generative AI.
AGI would not just rely on pattern recognition but would possess a level of cognitive flexibility, creativity, and reasoning that current AI lacks. While AGI is still a distant goal, its realization could allow for AI systems that are more adaptive, intuitive, and capable of handling diverse and unpredictable challenges.
As AI continues to evolve, the most effective use cases will likely involve human-AI collaboration rather than AI functioning autonomously.
AI can assist by handling repetitive tasks, generating content, or processing vast datasets, while humans provide the emotional intelligence, critical thinking, and decision-making required for complex situations. This collaboration could enhance productivity, creativity, and problem-solving across industries.
The inability of AI to understand its output can lead to several significant consequences, especially in sensitive, high-stakes applications. Since generative AI needs more true comprehension of the content it produces, it often generates results based on patterns rather than context or intent. Here are some potential consequences:
AI-generated content can sometimes be factually incorrect, misleading, or incoherent. Since AI does not understand the information it produces, it might generate text or outputs that sound plausible but need to be corrected.
In contexts like news generation, medical advice, or legal documents, this can lead to misinformation, potentially causing harm to individuals or society.
AI systems often reflect the biases present in their training data. Since AI doesn't understand the social, cultural, or ethical implications of its outputs, it may produce biased, discriminatory, or unethical content.
For instance, a generative AI might generate text or images that perpetuate stereotypes or unfairly represent certain groups of people. These outputs can reinforce harmful biases and social injustices, particularly in sensitive areas like hiring, law enforcement, or healthcare.
AI models generate content based on statistical patterns rather than understanding the context in which the content is used. This can result in content that is inappropriate, irrelevant, or insensitive.
For example, an AI might generate responses to customer queries that sound logical but are out of context, leading to customer frustration. In creative fields, AI-generated content might be tone-deaf or disconnected from cultural or emotional nuances, reducing its effectiveness.
In cases where AI systems produce harmful or illegal content, such as defamatory statements, biased hiring decisions, or discriminatory marketing strategies, it becomes difficult to assign accountability.
Since the AI does not have a sense of responsibility, the creators, operators, or users of the system are left with the burden of addressing any legal consequences. This raises significant concerns around liability, especially when AI systems are deployed in critical industries like healthcare, finance, or autonomous driving.
AI models may unintentionally generate harmful outputs in complex or high-risk situations. For example, in autonomous vehicles, a lack of deep understanding could lead to poor decision-making during critical moments (like an accident) where a human would make a morally or ethically informed decision.
Similarly, in AI-assisted healthcare, errors in diagnosis or inappropriate treatment suggestions could harm patients. These situations underscore the need for human oversight to ensure that AI systems operate safely and effectively.
If AI systems consistently produce outputs that are confusing, inaccurate, or inappropriate, they can erode trust in the technology. Users may need to be convinced of AI's ability to handle complex tasks or make meaningful contributions.
This can slow down the adoption of AI in sectors like education, customer service, healthcare, and creative industries, where reliability and accuracy are crucial.
AI systems are increasingly used to personalize content, such as in recommendations for movies, music, or products. However, since the AI needs an understanding of user preferences and emotions, it might offer irrelevant or off-base recommendations.
This reduces the effectiveness of personalization and can lead to frustration or disengagement by users who expect more tailored experiences.
One thing current generative AI applications need help understanding the context and meaning behind the content they generate. Despite their ability to create text, images, or even music that appear coherent and creative, AI systems do not possess true comprehension or awareness. They generate outputs based on patterns and data correlations and need to understand the deeper significance, emotions, or intentions behind those outputs.
This lack of deep understanding limits AI's ability to navigate complex, nuanced situations and make morally or contextually informed decisions. As advanced as generative AI may be, it cannot replicate human reasoning, intuition, or emotional insight, which are essential for fully grasping the meaning of content and making decisions that align with human values.
Copy and paste below code to page Head section
The primary limitation of current generative AI applications is that they need help understanding the content they generate. AI cannot comprehend the context, emotions, or deeper meanings behind the outputs it creates. It operates based on patterns in data rather than any form of real awareness or cognitive insight.
Generative AI models are designed to predict and generate content based on statistical patterns found in the data they were trained on. While they can produce outputs that seem coherent or creative, these models do not have consciousness, self-awareness, or the ability to process information with human-like understanding or emotional intelligence.
No, generative AI cannot think like humans. It cannot reason, reflect, or experience emotions. While it can mimic human behavior to some extent by generating text or images that appear human-like, it does so by following learned patterns, not through independent thought or creativity.
No, generative AI cannot make moral or ethical decisions on its own. AI lacks the emotional intelligence and moral reasoning necessary to navigate complex ethical dilemmas. It operates based on pre-defined rules or patterns but without ta rue understanding of the ethical implications of its actions.
While future advancements may improve AI's ability to simulate deeper understanding or context awareness, current generative AI is fundamentally limited by its lack of consciousness and emotional insight. Overcoming this would require breakthroughs in artificial general intelligence (AGI) and is still far from realization.
AI generates content by analyzing vast amounts of data and learning patterns within it. For example, a language model like GPT generates text based on the likelihood of word sequences. In contrast, an image-generating model like DALL·E creates images by recognizing relationships between visual elements. However, this process is purely data-driven, with no real "understanding" of the content.