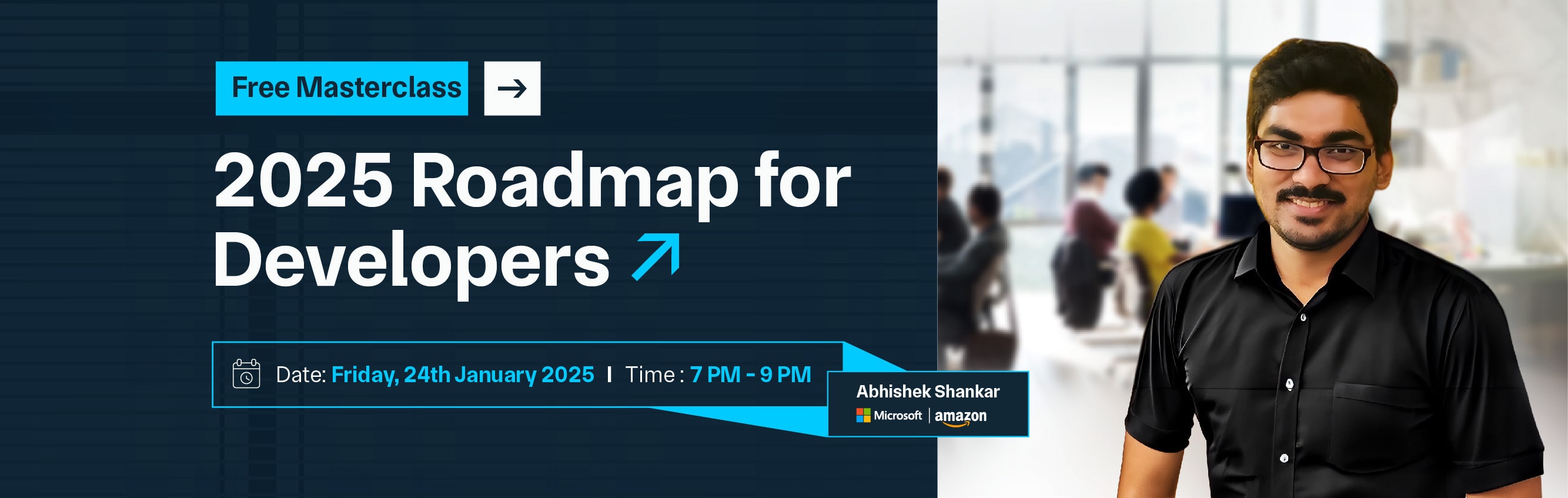

Hypothesis testing is a crucial aspect of data science, providing a structured framework for making informed decisions based on data analysis. It begins with formulating two competing hypotheses: the null hypothesis (H₀), which posits no effect or relationship, and the alternative hypothesis (H₁), which suggests otherwise. The process involves selecting an appropriate significance level (α), commonly set at 0.05, to determine the threshold for statistical significance.
Data is then collected through well-designed experiments or observational studies, ensuring high quality and relevance. Various statistical tests, such as t-tests, ANOVA, and chi-squared tests, are employed depending on the nature of the data and research question. These tests calculate a test statistic and corresponding p-value, allowing researchers to assess the likelihood of observing the data if H₀ is true.
If the p-value is less than α, H₀ is rejected in favor of H₁, indicating significant findings. However, it is essential to consider the context and practical implications of results, as statistical significance does not always equate to real-world relevance. Understanding the limitations of hypothesis testing, such as Type I and Type II errors, is also vital for accurate interpretation and application in data-driven decision-making.
Hypothesis testing is a statistical method used to make decisions or inferences about a population based on sample data. The process involves formulating two competing hypotheses:
Formulating hypotheses is a critical step in the hypothesis-testing process, as it sets the foundation for the entire analysis. Here’s a structured approach to formulating hypotheses:
Identifying the research question is the first step in hypothesis formulation. A clear, specific question guides the study and focuses the analysis. For example, rather than asking if students perform better, a more precise question could investigate how tutoring affects test scores.
This specificity ensures the research is targeted meaningful, and contributes to existing knowledge, setting a solid foundation for developing testable hypotheses.
The null hypothesis (H₀) serves as a baseline for testing, asserting that there is no effect, difference, or relationship between variables. It represents the status quo and provides a framework for comparison.
For instance, in studying tutoring impacts, H₀ might state that tutoring does not affect test scores. This formulation allows researchers to evaluate the evidence against H₀, guiding statistical analysis and ensuring rigorous conclusions.
The alternative hypothesis (H₁ or Hₐ) proposes that there is an effect, difference, or relationship between variables, challenging the null hypothesis. It can be directional (specifying the expected outcome) or non-directional (indicating a difference without specifying direction).
For instance, H₁ could state that tutoring improves test scores. A clear alternative hypothesis focuses the analysis, guiding researchers toward specific expectations and facilitating meaningful interpretation of results.
Clarity and specificity are essential in formulating hypotheses to avoid ambiguity and misinterpretation. Each hypothesis should be clearly stated and well-defined, outlining the variables and expected relationships.
For example, instead of a vague statement about teaching methods, a specific hypothesis might assert that interactive methods lead to higher test scores. This precision enhances data collection, analysis, and the communication of findings, contributing to the study’s overall rigor.
Considering the context is vital when formulating hypotheses, as it ensures relevance and applicability. Researchers should understand the theoretical framework and existing literature related to their question.
This background helps identify knowledge gaps and informs hypothesis development. For instance, examining prior studies on educational interventions can shape more targeted hypotheses. Contextual awareness enhances the significance of findings, making them valuable to practitioners and contributing to the field's understanding.
Aligning hypotheses with appropriate statistical tests is crucial for rigorous analysis. Different tests suit specific data types and research questions, influencing how hypotheses are structured. For example, if comparing means, a t-test might be used, requiring hypotheses that reflect this focus.
Understanding the assumptions of various tests helps refine hypotheses, ensuring they are testable and relevant. This alignment enhances the reliability of conclusions drawn from data, contributing to robust research findings.
Hypothesis testing is a fundamental aspect of statistical analysis that enables researchers to conclude populations based on sample data. By evaluating whether observed effects or differences are statistically significant, hypothesis testing provides a structured approach to decision-making in various fields, from healthcare to social sciences.
Central to this process is the selection of appropriate statistical tests, which can be categorized into parametric and non-parametric tests. Understanding these tests is crucial for accurate analysis and interpretation of data, ensuring that findings are both reliable and meaningful.
Parametric tests assume that the data follows a specific distribution, typically a normal distribution. They are used when the data meets certain criteria regarding scale, distribution, and variance. Common parametric tests include:
Non-parametric tests do not assume a specific distribution and are often used when the data does not meet the assumptions of parametric tests. They are more flexible and applicable to ordinal data or non-normally distributed data. Common non-parametric tests include:
Selecting the appropriate test depends on several factors, including:
By understanding the types of tests and their applications, researchers can make informed choices in their analysis, leading to valid and reliable conclusions.
Hypothesis testing is a systematic process used to evaluate assumptions about a population based on sample data. It begins with formulating two competing hypotheses: the null hypothesis (H₀), which posits no effect or difference, and the alternative hypothesis (H₁), which suggests otherwise.
Researchers then choose a significance level (α), typically set at 0.05, to determine the threshold for statistical significance. After collecting relevant sample data, they select an appropriate statistical test—such as a t-test or ANOVA—based on the data type and research question. The test yields a test statistic and a p-value, the latter indicating the probability of observing the data under H₀.
If the p-value is less than α, the null hypothesis is rejected; otherwise, it is not. Finally, researchers interpret the results within the context of their study, considering practical significance and communicating their findings clearly while also addressing any limitations in their analysis. This structured approach ensures that conclusions drawn are evidence-based and methodologically sound.
This table highlights the key differences between hypothesis generation and hypothesis testing, illustrating their distinct roles in the research process.
Here are some real-life examples of hypothesis testing across various fields:
In medical research, hypothesis testing is often employed to evaluate the effectiveness of new treatments. For example, researchers might hypothesize that a new drug is more effective than a standard treatment in reducing blood pressure. To test this, they would conduct a randomized controlled trial where one group of participants receives the new drug while another group receives the standard treatment.
After a specified period, researchers measure the blood pressure levels in both groups. They would use a t-test to analyze the results and determine if the observed differences in blood pressure reduction are statistically significant. This process helps validate the drug's effectiveness, informing clinical decisions and potential approvals.
In the field of education, hypothesis testing can be used to assess the impact of interventions such as tutoring programs. For instance, a hypothesis might state that students who participate in after-school tutoring will achieve higher math scores than those who do not. To evaluate this, an educator collects test scores from both groups and conducts an analysis, such as ANOVA, to compare the mean scores.
By examining whether the difference in scores is statistically significant, educators can determine if the tutoring program effectively enhances student performance, thereby guiding future educational strategies and resource allocation.
In marketing, businesses often test new advertising strategies to gauge their effectiveness. For instance, a company might hypothesize that a new advertising campaign will increase sales compared to a previous campaign. To test this hypothesis, the company might implement the new campaign in one region while maintaining the old campaign in another region.
By analyzing sales data from both areas using a chi-squared test, the company can determine if there is a statistically significant difference in sales figures. This information helps the company decide whether to adopt the new campaign more broadly or revise its marketing strategy.
Hypothesis testing is also prevalent in agricultural research, where it helps assess the effectiveness of various farming practices. For example, a farmer might hypothesize that a specific fertilizer leads to higher crop yields than using no fertilizer at all. To test this, they would set up an experiment with two groups of plants: one group receives the fertilizer while the other does not.
At harvest time, the farmer measures the crop yields and uses a t-test to analyze the data for significant differences. The results guide the farmer’s decisions on fertilizer use, ultimately impacting crop production and profitability.
In psychology, hypothesis testing is crucial for evaluating the effectiveness of therapeutic interventions. For instance, researchers might hypothesize that cognitive behavioral therapy (CBT) reduces anxiety more effectively than standard counseling. To test this, participants with diagnosed anxiety disorders are randomly assigned to receive either CBT or standard counseling.
After the treatment, their anxiety levels are measured, and a paired t-test is conducted to assess differences between the two groups. The results provide valuable insights into the most effective therapeutic approaches, informing practitioners and improving patient outcomes.
In economics, hypothesis testing helps analyze the impact of policy changes on economic indicators. For instance, economists might hypothesize that an increase in the minimum wage does not affect employment levels. To investigate this, they would analyze employment data before and after the wage increase across various regions. By employing regression analysis, they can determine if there is a statistically significant relationship between the wage change and employment rates.
The findings from such studies inform policymakers and stakeholders about the potential consequences of wage adjustments, guiding economic decisions, and labor policies. These examples illustrate the diverse applications of hypothesis testing across various fields, highlighting its importance in making informed, evidence-based decisions.
Making decisions based on hypothesis testing involves interpreting statistical results in the context of the research question, weighing evidence, and considering practical implications. Here’s how the decision-making process typically unfolds:
Once the hypothesis testing process is complete, researchers analyze the results, focusing primarily on the p-value obtained from the statistical test. The p-value indicates the probability of observing the data if the null hypothesis (H₀) is true. If the p-value is less than the predetermined significance level (commonly set at 0.05), researchers reject the null hypothesis in favor of the alternative hypothesis (H₁).
This step suggests that there is statistically significant evidence to support the alternative hypothesis. However, if the p-value is greater than 0.05, researchers fail to reject H₀, indicating insufficient evidence to support the alternative.
Interpreting results also requires a consideration of the study’s context. Researchers should evaluate whether the statistical significance translates into practical significance. For instance, a drug may show statistically significant improvements in patient outcomes, but if the improvement is minimal, it may not be clinically meaningful.
Context includes factors such as sample size, effect size, and the relevance of findings to the real world. Researchers should also take into account any limitations of the study, including biases, confounding variables, or issues with data quality.
Based on the interpretation of the results, researchers make informed decisions about the implications of their findings. This might involve recommending further research, changing practices or policies, or implementing new interventions.
For example, if a study finds that a new teaching method significantly improves student performance, educators may choose to adopt this method in their curricula. Conversely, if results do not support the hypothesis, researchers may explore alternative explanations or revise their approaches.
Effectively communicating the results and their implications is essential for decision-making. Researchers should present their findings clearly, often using visual aids like graphs or tables, and provide context for their conclusions.
This communication is vital for stakeholders—such as policymakers, educators, or healthcare professionals—who rely on the research to inform their decisions. Transparency about the study's limitations and uncertainties also fosters trust and facilitates informed decision-making.
Finally, reflecting on the hypothesis testing process and the decision-making outcomes is essential for continuous improvement in research practices. Researchers should consider what worked well, what challenges arose, and how findings can inform future studies.
This reflection not only enhances individual research projects but also contributes to the broader scientific community by promoting better methodologies and practices in hypothesis testing.
Performing a hypothesis test is a systematic process that helps researchers evaluate assumptions about a population using sample data. This process involves defining hypotheses, selecting a significance level, collecting data, and applying appropriate statistical tests to conclude. Here are the key steps involved:
This structured approach ensures that conclusions drawn from hypothesis testing are reliable and meaningful.
Collecting data is a crucial step in the hypothesis testing process, as the quality and relevance of the data directly impact the validity of the results. This phase involves designing a data collection plan, selecting appropriate methods, and ensuring that the data gathered is representative of the population being studied. Here are the key considerations and steps involved in collecting data:
1. Define the Population: Clearly identify the population of interest, which is the larger group you want to make inferences about.
2. Choose a Sampling Method: Select an appropriate sampling method to ensure that the sample is representative. Common methods include:
3. Determine Sample Size: Calculate an adequate sample size to ensure statistical power, which helps detect meaningful effects. This often involves considering the expected effect size, significance level, and desired power of the test.
4. Select Data Collection Methods: Choose the appropriate methods for collecting data, such as:
5. Develop Data Collection Instruments: Create or select tools for data collection, ensuring they are reliable and valid. This might include designing survey questions or preparing measurement protocols.
6. Pilot Testing: Conduct a pilot study to test the data collection process and instruments, allowing for adjustments based on feedback and initial findings.
7. Implement Data Collection: Execute the data collection plan, ensuring adherence to ethical standards, including informed consent and confidentiality.
8. Monitor Data Quality: Continuously check for data accuracy and integrity during the collection process, addressing any issues that arise promptly.
9. Organize and Store Data: Once collected, organize the data systematically for analysis. This may involve data entry, cleaning, and ensuring that the data is stored securely.
Collecting high-quality data is essential for reliable hypothesis testing and ensures that subsequent analyses yield valid and meaningful conclusions.
While hypothesis testing is a powerful tool for statistical analysis, it has several limitations that researchers must consider. Understanding these limitations is crucial for interpreting results accurately and making informed decisions. Here are some key limitations:
Understanding these limitations is essential for researchers to interpret their findings accurately and to communicate the results responsibly. By acknowledging these constraints, researchers can approach hypothesis testing with a critical mindset and enhance the quality of their conclusions.
Hypothesis testing is a vital component of statistical analysis that enables researchers to draw meaningful conclusions from data. By systematically evaluating assumptions about populations based on sample data, hypothesis testing provides a structured approach to decision-making in various fields, including medicine, education, marketing, and more.
The process involves defining null and alternative hypotheses, selecting appropriate significance levels, collecting data, and applying statistical tests to assess the validity of the hypotheses.
Copy and paste below code to page Head section
Hypothesis testing is a statistical method used to evaluate assumptions or claims about a population based on sample data. It involves formulating a null hypothesis (H₀) and an alternative hypothesis (H₁), collecting data, and using statistical tests to determine if there is enough evidence to reject H₀.
Hypothesis testing is crucial for making data-driven decisions in various fields. It allows researchers to assess the validity of their assumptions, determine the significance of their findings, and draw conclusions that can inform practices, policies, or further research.
A p-value is a statistical measure that indicates the probability of observing the data or something more extreme if the null hypothesis is true. A low p-value (typically ≤ 0.05) suggests that the observed effect is statistically significant and provides evidence against the null hypothesis.
Choosing the appropriate statistical test depends on the research question, the type of data, and the number of groups being compared. Common tests include t-tests for comparing means, ANOVA for comparing multiple groups, and chi-squared tests for categorical data.
The significance level (α) is the threshold set by the researcher to determine whether to reject the null hypothesis. A common choice is 0.05, meaning there is a 5% risk of making a Type I error.
While hypothesis testing is a valuable tool, results should be interpreted cautiously. Factors such as sample size, study design, and potential biases can influence outcomes. It’s essential to consider the context and limitations of the study.