
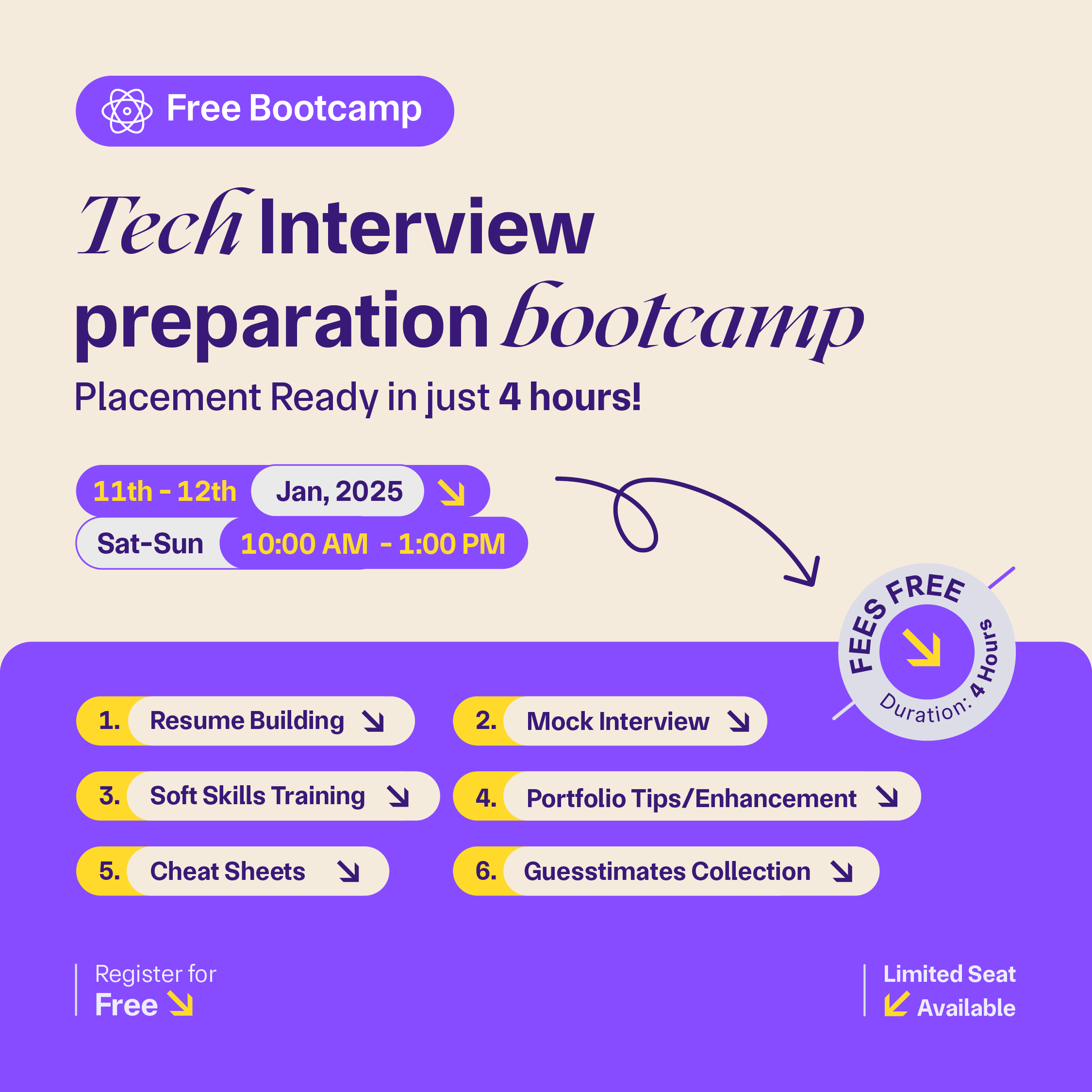
The future of data analytics is poised for significant transformation as advancements in technology continue to reshape how organizations harness and interpret data. Emerging technologies such as artificial intelligence (AI) and machine learning (ML) are driving the evolution of data analytics, enabling more sophisticated data processing, predictive insights, and automation. These technologies allow businesses to analyze vast amounts of data in real-time, uncovering patterns and trends that were previously inaccessible. The integration of AI and ML will enhance decision-making processes, making analytics more predictive and prescriptive.
Another key trend in the future of data analytics is the rise of real-time and streaming analytics. With the increasing volume of data generated from IoT devices, social media, and other sources, organizations are shifting towards real-time data processing to gain immediate insights. This capability allows businesses to respond more swiftly to changing conditions, optimize operations, and deliver personalized experiences to customers. Real-time analytics will become essential for staying competitive in a rapidly evolving marketplace.
Additionally, the focus on data privacy and security will intensify as regulations become more stringent and concerns about data breaches grow. Organizations will need to implement robust data governance frameworks and leverage advanced security measures to protect sensitive information. The future of data analytics will involve balancing innovation with ethical considerations, ensuring that data usage aligns with privacy standards, and fostering trust among stakeholders.
Data analytics involves the systematic computational analysis of data to uncover patterns, correlations, and insights that inform decision-making and drive strategic actions. It encompasses a variety of techniques and tools used to examine raw data with the aim of extracting meaningful information. The process often includes data collection, cleaning, transformation, and modeling to reveal trends and predict future outcomes.
Data analytics is applied across various domains, such as business, healthcare, finance, and technology, to enhance efficiency, optimize processes, and solve complex problems. At its core, data analytics relies on statistical methods and algorithms to interpret large volumes of data, converting it into actionable insights.
This process can be descriptive, focusing on historical data to describe what has happened; diagnostic, identifying reasons behind past events; predictive, forecasting future trends based on historical data; or prescriptive, recommending actions to achieve desired outcomes. By leveraging data analytics, organizations can make informed decisions, improve performance, and gain a competitive edge in their respective industries.
Data analytics plays a crucial role in modern decision-making by transforming raw data into valuable insights. It helps organizations understand trends, patterns, and relationships within their data, leading to more informed and strategic decisions.
By leveraging data analytics, businesses can optimize operations, enhance customer experiences, and gain a competitive edge. The ability to analyze data effectively supports improved efficiency, innovation, and risk management across various sectors.
The role of a data analyst is continually evolving as organizations increasingly rely on data to drive decisions and strategy. Traditionally focused on data collection, cleaning, and reporting, modern data analysts now engage in more complex activities, including predictive modeling, data visualization, and advanced analytics.
As technology advances and data volumes grow, data analysts are expected to integrate new tools and methodologies to extract actionable insights. This evolution is driven by the need for more sophisticated data analysis to address dynamic business challenges and support strategic decision-making.
Data analysts are leveraging predictive modeling techniques to forecast future trends based on historical data. This involves applying statistical methods and machine learning algorithms to analyze past data and predict future outcomes.
Predictive analytics helps organizations anticipate changes in market conditions, customer behavior, and operational performance, allowing for more proactive decision-making and strategic planning. By integrating predictive insights into their strategies, businesses can better prepare for potential challenges and opportunities.
Creating interactive and insightful visualizations has become a critical responsibility for data analysts. Effective visualizations transform complex data sets into understandable and actionable insights.
This involves using tools and techniques to create charts, graphs, and dashboards that highlight key trends and patterns. By presenting data in a visually appealing and understandable manner, data analysts enable stakeholders to grasp critical information and make informed decisions quickly.
Data analysts are increasingly involved in advanced analytics, which includes data mining and pattern recognition. These techniques go beyond basic reporting to uncover deeper insights from data.
Advanced analytics involves exploring large datasets to identify hidden patterns, correlations, and anomalies. This level of analysis supports more strategic decision-making by providing a deeper understanding of underlying factors and trends that influence business performance.
Modern data analysts work closely with business leaders to ensure that data analysis aligns with organizational goals. This requires a thorough understanding of business needs and the ability to translate data insights into actionable strategies.
By aligning their analysis with business objectives, data analysts help organizations make data-driven decisions that support overall strategic goals and enhance organizational performance.
Ensuring data quality and compliance with data governance standards is a critical responsibility for data analysts. This involves maintaining data integrity, security, and accuracy while adhering to best practices in data management.
Data analysts must implement data governance frameworks to ensure that data is reliable and used appropriately, minimizing risks related to data breaches and inaccuracies.
The implementation of automation tools to streamline data processes is becoming increasingly important. Data analysts are responsible for automating repetitive tasks, such as data cleaning and reporting, to improve efficiency and reduce manual effort.
By using scripts and automation tools, analysts can handle large volumes of data more effectively, allowing them to focus on higher-value analysis and decision support.
Data analysts often collaborate with various departments, including IT, marketing, and finance, to ensure that data insights are relevant and actionable across the organization.
This collaborative approach enhances the impact of data analysis by integrating diverse perspectives and expertise. Effective communication and teamwork enable data analysts to provide insights that address cross-departmental needs and support overall business objectives.
Big Data Analytics is a rapidly evolving field that continues to shape how businesses operate and make decisions. With the increasing availability of data from various sources, organizations are leveraging advanced analytics to gain insights, improve efficiency, and drive innovation. The future of Big Data Analytics promises even more transformative changes with the integration of artificial intelligence, real-time analytics, and more sophisticated tools.
These advancements are expected to enhance predictive capabilities, enable personalized experiences, and foster new opportunities across industries. Here are some key trends and developments that will define the future of Big Data Analytics:
Artificial Intelligence (AI) and Machine Learning (ML) are transforming Big Data Analytics by automating complex data processing tasks and generating more accurate insights. The future will see a deeper integration of AI and ML, allowing for real-time analysis and decision-making.
As these technologies advance, they will enhance predictive analytics, enable the discovery of hidden patterns, and drive automated responses. This integration will also lead to more personalized user experiences as systems learn from user behavior and adapt to individual preferences.
The demand for real-time data processing is growing as businesses seek to respond quickly to changing market conditions and customer needs. Future developments in Big Data Analytics will focus on reducing latency and increasing processing speed, enabling organizations to analyze and act on data as it is generated.
This shift will empower businesses to make more informed decisions, improve operational efficiency, and offer more timely and relevant customer interactions. Real-time analytics will be crucial in industries like finance, healthcare, and e-commerce, where timely information is vital.
As data becomes more valuable, the need for robust security and privacy measures grows. The future of Big Data Analytics will involve more sophisticated techniques for protecting sensitive information and ensuring compliance with regulations such as GDPR.
Innovations in encryption, anonymization, and access controls will be key to safeguarding data and maintaining customer trust. Additionally, organizations will need to focus on ethical data usage, balancing the benefits of analytics with the rights and privacy of individuals.
Edge computing is set to revolutionize Big Data Analytics by bringing data processing closer to the source. This approach reduces latency and bandwidth usage, enabling faster and more efficient data analysis.
The future will see increased adoption of edge computing, particularly in IoT applications where real-time insights are crucial. This shift will allow businesses to analyze data at the edge of the network, leading to quicker decision-making and enhanced performance in areas such as manufacturing, transportation, and smart cities.
Predictive and prescriptive analytics will play a more significant role in decision-making as organizations look to anticipate future trends and optimize outcomes. Advances in algorithms and computing power will enhance the accuracy and reliability of these analytics, allowing businesses to forecast trends and prescribe actions more effectively.
This expansion will enable organizations to proactively address challenges, seize opportunities, and improve strategic planning. Industries like finance, healthcare, and retail will particularly benefit from these advanced analytics capabilities.
Data-as-a-Service (DaaS) is becoming increasingly popular as organizations seek flexible and scalable solutions for data management and analysis. The future will see further growth in DaaS offerings, providing businesses with on-demand access to data and analytics tools.
This model allows companies to leverage external data sources and analytics capabilities without the need for extensive in-house infrastructure. DaaS will enable organizations to focus on their core competencies while benefiting from specialized data insights and services.
The democratization of data analytics refers to making analytics tools and insights accessible to a broader range of users within an organization. The future will see more user-friendly interfaces and self-service analytics platforms that empower employees at all levels to leverage data insights.
This democratization will foster a data-driven culture, encouraging collaboration and innovation across departments. By enabling more employees to make data-informed decisions, organizations can enhance productivity and drive better business outcomes.
Data visualization is a critical aspect of Big Data Analytics, enabling users to understand complex data through intuitive visuals. The future will bring more advanced visualization techniques, incorporating interactive elements and immersive experiences like virtual and augmented reality.
These innovations will make it easier for users to explore data, identify patterns, and communicate insights effectively. Enhanced data visualization will support decision-making and storytelling, allowing organizations to convey complex information in more engaging and impactful ways.
As AI and Big Data Analytics become more prevalent, there will be a growing emphasis on ethical AI and data use. The future will involve developing frameworks and guidelines to ensure transparency, fairness, and accountability in AI systems and data practices.
Organizations will need to address biases in algorithms, ensure data integrity, and prioritize ethical considerations in their analytics initiatives. By focusing on ethical AI and data use, businesses can build trust with stakeholders and contribute to a more equitable digital future.
The future of Big Data Analytics will see increased collaboration across industries, driving innovation and unlocking new opportunities. By sharing data and insights, organizations can tackle complex challenges and develop solutions that benefit multiple sectors. Cross-industry collaboration will foster innovation in areas such as healthcare, transportation, and environmental sustainability.
As industries come together to leverage Big Data Analytics, they can address global issues and create value that extends beyond individual organizations. These trends and developments highlight the transformative potential of Big Data Analytics and its ability to shape the future of business and society.
A career as a Data Analyst offers an exciting opportunity to work at the intersection of technology and business. Data Analysts play a crucial role in helping organizations make informed decisions by collecting, processing, and analyzing data. They transform raw data into meaningful insights that drive strategic planning and operational improvements.
As data becomes increasingly central to business success, the demand for skilled Data Analysts continues to grow across various industries such as finance, healthcare, retail, and technology. This career path not only offers competitive salaries and growth opportunities but also allows individuals to impact decision-making processes significantly.
Data Analysts are responsible for collecting and organizing data from various sources, cleaning and preprocessing it to ensure accuracy, and analyzing it to extract meaningful insights. They use statistical tools and techniques to interpret complex datasets and present their findings through visualizations and reports to aid in decision-making.
Key skills for Data Analysts include proficiency in data analysis tools like Excel, SQL, Python, and R. Strong analytical and problem-solving skills, attention to detail, and the ability to communicate findings effectively are essential. Familiarity with data visualization tools such as Tableau or Power BI is also beneficial.
Most Data Analysts hold a bachelor's degree in fields like data science, statistics, mathematics, computer science, or business. Advanced degrees or certifications in data analytics can enhance job prospects and open up more senior positions.
Data Analysts are in high demand across various industries, including finance, healthcare, marketing, e-commerce, and government sectors. Businesses rely on data-driven insights to improve operations, optimize marketing strategies, and enhance customer experiences.
A Data Analyst career offers numerous opportunities for advancement. With experience, analysts can progress to roles such as Senior Data Analyst, Data Scientist, Data Engineer, or Business Intelligence Analyst. Continuous learning and keeping up with industry trends can accelerate career growth.
Data Analysts play a vital role in shaping business strategies by providing insights that drive informed decisions. Their analyses can lead to cost savings, improved efficiency, and better customer experiences, ultimately contributing to an organization's success.
Data Analysts use various tools and technologies to analyze data, such as SQL for database querying, Excel for data manipulation, Python and R for statistical analysis, and Tableau or Power BI for data visualization. Staying updated with the latest tools enhances effectiveness.
Salaries for Data Analysts vary based on experience, location, and industry. Entry-level analysts can expect competitive salaries, with the potential for significant increases as they gain experience and expertise in the field.
Data analytics encompasses various methodologies, each serving distinct purposes to extract insights from data. Understanding the different types of analytics helps organizations address specific business needs and make informed decisions. The main types of data analytics include descriptive, diagnostic, predictive, and prescriptive analytics.
Each type plays a crucial role in interpreting data, understanding past events, forecasting future outcomes, and recommending actions. By employing these analytic techniques, businesses can gain comprehensive insights, optimize performance, and strategize effectively.
Data analytics tools are essential for processing, analyzing, and visualizing data to derive meaningful insights. These tools enable organizations to handle vast amounts of data efficiently, apply complex analytical techniques, and present results in an understandable format.
They support various functions such as data collection, data cleaning, statistical analysis, and data visualization. By leveraging advanced analytics tools, businesses can enhance their decision-making processes, uncover trends, and drive strategic initiatives based on data-driven insights.
Data analytics is continuously evolving, driven by advancements in technology and the growing demand for actionable insights. The latest trends in data analytics reflect the industry's focus on improving data accuracy, enhancing decision-making processes, and leveraging innovative tools and techniques.
These trends are shaping how businesses collect, process, and analyze data, allowing them to stay competitive and meet the changing needs of their customers. From the rise of artificial intelligence to the increasing importance of data privacy, these developments are transforming the landscape of data analytics.
Data mesh architecture is emerging as a decentralized approach to managing data. Unlike traditional centralized data storage and processing, data mesh distributes data responsibilities across various teams or domains within an organization.
This approach enhances data ownership, reduces bottlenecks, and promotes scalability. By decentralizing data management, organizations can achieve more efficient and flexible data handling, fostering innovation and responsiveness to business needs.
Blockchain technology is gaining attention for its potential to ensure data integrity and security. By creating a decentralized, immutable ledger, blockchain technology helps verify and secure data transactions, protecting against tampering and unauthorized access.
This is particularly valuable in sectors requiring high levels of data trust and transparency, such as finance, supply chain management, and healthcare, where maintaining data accuracy and authenticity is crucial.
Synthetic data generation involves creating artificial datasets that simulate real-world data for testing and training purposes. This method addresses issues related to data privacy, availability, and quality by providing a controlled environment for experimentation and model development.
Synthetic data is especially useful for enhancing machine learning models and conducting simulations, enabling organizations to explore new scenarios without compromising sensitive or limited real-world data.
Quantum computing represents a groundbreaking advancement in data processing technology. Although still in its nascent stages, quantum computing holds the potential to solve complex data problems that classical computers struggle with.
By leveraging quantum bits (qubits) and quantum algorithms, this technology could revolutionize data analytics, offering unprecedented processing speeds and the ability to tackle more sophisticated modeling and analytical challenges.
Fostering a data-driven culture is becoming increasingly essential for organizations aiming to maximize the value of their data. This trend focuses on embedding data into decision-making processes across all levels of an organization.
It involves promoting data literacy among employees, encouraging data-centric thinking, and facilitating collaboration around data insights. A strong data-driven culture empowers teams to make informed decisions and drive business growth.
Explainable AI (XAI) is crucial for making AI and machine learning models more transparent and understandable. As AI systems become more intricate, there is a growing need to explain how models reach their conclusions.
XAI aims to provide clear explanations of model behavior and decision-making processes, enhancing trust in AI systems and ensuring that their outputs are accountable and interpretable by users.
Advancements in data visualization are transforming how insights are presented and interpreted. Innovations such as augmented reality (AR) and virtual reality (VR) are offering new ways to interact with and explore data.
These immersive visualization tools help users engage with data in more intuitive and meaningful ways, leading to deeper insights and a better understanding of complex datasets.
Augmented data analytics combines advanced analytics capabilities with automation to enhance data analysis processes. This trend leverages artificial intelligence to automate routine tasks, such as data cleaning and report generation, making the analytics process more efficient.
Augmented analytics tools help users derive insights faster and more accurately, reducing the manual effort required and accelerating decision-making.
A career as a Data Analyst offers numerous opportunities for professional growth and advancement. By continuously developing skills and expanding expertise, Data Analysts can position themselves for higher-level roles and increased responsibilities.
The field of data analytics is dynamic, with emerging technologies and evolving business needs creating pathways for career enhancement. Leveraging these opportunities effectively can lead to significant career boosts, offering both personal and professional satisfaction. Here’s how Data Analysts can advance their careers and capitalize on new opportunities:
A successful career in data analytics requires a combination of technical expertise, analytical thinking, and strong communication skills. Data Analysts must be adept at handling complex datasets, using various tools and techniques to derive meaningful insights.
As businesses increasingly rely on data-driven decision-making, possessing a well-rounded skill set is crucial for effectively analyzing data and driving strategic outcomes. Here’s a look at the key skills needed for a career in data analytics:
Data analytics has become a critical component for businesses striving to stay competitive and make informed decisions. By leveraging data analytics, organizations can transform raw data into actionable insights, driving strategic initiatives and improving operational efficiency.
The benefits of data analytics extend across various facets of business operations, from enhancing decision-making to optimizing processes and gaining a deeper understanding of customer behavior.
Embracing data analytics enables businesses to make data-driven decisions, which can lead to significant improvements in performance, profitability, and overall success. Here’s a closer look at the key benefits of data analytics:
Data analytics provides businesses with valuable insights derived from extensive data analysis, allowing for more informed and evidence-based decision-making. By examining trends, patterns, and anomalies in data, organizations can make strategic decisions that are supported by empirical evidence rather than relying on intuition alone.
This leads to better outcomes and helps mitigate the risks associated with decision-making. For example, sales data analysis can inform product launch strategies, while customer behavior analysis can guide marketing efforts.
Data analytics helps organizations identify inefficiencies and bottlenecks within their operations. By analyzing performance metrics and process data, businesses can pinpoint areas that require improvement, streamline workflows, and optimize resource allocation.
This leads to significant cost savings and enhanced productivity. For instance, analyzing supply chain data can reveal opportunities to reduce delays, improve inventory management, and lower operational costs.
Through data analytics, businesses can gain a deeper understanding of customer behavior, preferences, and needs. By analyzing customer data, such as purchase history and interaction patterns, organizations can tailor their products, services, and marketing strategies to meet customer expectations better.
This results in increased customer satisfaction and loyalty. For example, personalized marketing campaigns based on customer data can drive higher engagement and conversion rates.
Data analytics enables organizations to identify opportunities for revenue growth and improved profitability. By examining sales data, market trends, and customer preferences, businesses can develop targeted strategies to optimize pricing models, discover new revenue streams, and enhance sales performance.
This leads to increased sales and higher profit margins. For instance, analyzing sales trends can help identify profitable product lines and optimize pricing strategies to maximize revenue.
Utilizing data analytics provides a competitive edge by allowing businesses to stay ahead of industry trends and market shifts. By analyzing competitive data and market dynamics, organizations can make proactive decisions, adapt to changes, and seize opportunities before their competitors.
This strategic advantage helps businesses maintain their market position and drive growth. For example, predictive analytics can forecast market trends, allowing companies to adapt their strategies accordingly.
Data analytics plays a crucial role in identifying and mitigating risks by providing insights into potential threats and vulnerabilities. By analyzing historical data and modeling various scenarios, businesses can develop strategies to manage risks, prevent issues, and ensure business continuity.
This proactive approach helps organizations avoid costly disruptions and safeguard their operations. For instance, financial risk analysis can help identify potential vulnerabilities and implement measures to mitigate financial risks.
Data analytics aids in the development and refinement of products by providing insights into customer preferences, market demands, and product performance. By analyzing feedback, usage data, and market trends, businesses can create products that align more closely with customer needs and market expectations.
This leads to better product offerings and increased market success. For example, analyzing user feedback can guide product improvements and feature enhancements.
By leveraging data analytics, businesses can develop more effective marketing strategies tailored to their target audience. Analyzing customer data, campaign performance, and market trends enables organizations to create targeted marketing campaigns, optimize advertising spend, and improve customer engagement.
This leads to more efficient marketing efforts and higher return on investment. For instance, data-driven insights can help personalize marketing messages and identify the most effective channels for reaching customers.
Yes, data analysts are in high demand across a wide range of industries. As organizations increasingly recognize the value of data-driven decision-making, the need for professionals who can interpret complex datasets and provide actionable insights continues to grow. According to the U.S. Bureau of Labor Statistics, employment for data analysts is expected to grow by 25% from 2020 to 2030, which is significantly faster than the average for all occupations.
This surge in demand reflects the growing reliance on data across sectors such as finance, healthcare, e-commerce, and technology. In addition to strong job growth, data analysts are experiencing competitive salaries. The median annual salary for a data analyst in the United States was approximately $77,000 in 2023, with potential increases based on experience, location, and industry.
For example, data analysts working in major tech hubs like San Francisco or New York can earn significantly higher salaries. The expanding role of data analytics in driving business strategy, coupled with a shortage of skilled professionals, ensures that data analysts will remain highly sought after in the job market.
A career in data analytics often starts with roles such as Data Analyst or Junior Data Analyst, where professionals focus on data collection, cleaning, and initial interpretation. These entry-level positions typically involve using tools like Excel and SQL to generate reports and basic visualizations. As individuals gain experience, they can advance to roles like Senior Data Analyst or Data Scientist, where responsibilities grow to include advanced statistical analyses, predictive modeling, and using sophisticated tools like Python and R.
These roles offer opportunities to contribute to strategic decision-making and influence business outcomes. With mid-career experience, professionals may transition to specialized positions such as Data Engineer or Machine Learning Engineer. Data Engineers design and build data pipelines and manage data infrastructure, while Machine Learning Engineers develop algorithms and predictive models. Both roles require advanced technical skills and offer the chance to work on high-impact projects that leverage data for business innovation.
In the future, emerging roles such as Chief Data Officer (CDO) and Analytics Consultant will become more prominent. These positions focus on overseeing data strategy governance and providing expert consulting on data-driven initiatives. Additionally, the rise of AI and big data will lead to roles like AI Analyst and Big Data Specialist, which will demand a blend of technical expertise and strategic insight to drive business growth.
The future of data analytics in business looks exceptionally promising as organizations increasingly rely on data to drive strategic decisions and optimize operations. Advancements in technology, such as artificial intelligence (AI), machine learning (ML), and big data platforms, are expected to enhance the capabilities of data analytics further.
Businesses will increasingly adopt real-time analytics, enabling them to make more timely and informed decisions. Predictive analytics will become more sophisticated, allowing companies to anticipate market trends and customer behavior with greater accuracy.
Additionally, the integration of advanced data visualization tools will facilitate more intuitive and interactive data exploration. As data analytics evolves, it will continue to play a pivotal role in shaping business strategies, improving operational efficiency, and fostering innovation.
Data analytics has become the cornerstone of modern business practices due to its profound ability to convert vast amounts of raw data into actionable insights. As organizations face increasingly complex market dynamics and customer expectations, data analytics provides a clear advantage by enabling evidence-based decision-making.
By harnessing the power of data, businesses can enhance their competitive edge, optimize strategies, and drive operational improvements. The integration of data analytics into everyday business operations has proven to be essential for adapting to market changes, understanding customer behavior, and fostering innovation.
Data analytics is increasingly recognized for its ability to drive innovation and efficiency across a wide range of sectors. Each industry harnesses the power of data to tackle specific challenges, optimize operations, and make strategic decisions.
By applying analytical techniques tailored to their unique needs, sectors can unlock valuable insights, improve performance, and stay competitive in a rapidly evolving landscape. Understanding how data analytics impacts various fields underscores its essential role in modern business practices and its transformative potential across different domains.
In the finance sector, data analytics plays a pivotal role in enhancing risk management, detecting fraudulent activities, and optimizing investment strategies. Financial institutions analyze vast amounts of data to identify patterns and trends that inform investment decisions and risk assessments. Advanced analytics is used to develop predictive models that forecast market movements and evaluate credit risks.
By leveraging data, banks and investment firms can offer personalized financial products, manage portfolios more effectively, and improve regulatory compliance. Data-driven insights help in detecting anomalies and fraud, ensuring the integrity and security of financial transactions.
Data analytics in healthcare is revolutionizing patient care, operational efficiency, and medical research. By analyzing patient records, medical history, and treatment outcomes, healthcare providers can identify trends, predict disease outbreaks, and personalize treatment plans. Predictive analytics helps in early diagnosis and management of chronic conditions, while data-driven insights support clinical decision-making and improve patient outcomes.
Data analytics also aids in optimizing hospital operations, managing resources, and reducing costs. Moreover, it supports research and development efforts by uncovering insights into disease patterns and treatment effectiveness, contributing to advancements in medical science.
In the marketing sector, data analytics is essential for understanding consumer behavior, optimizing marketing campaigns, and measuring return on investment (ROI). By analyzing customer data, marketers can segment audiences, track campaign performance, and tailor messages to meet specific needs.
Advanced analytics provides insights into customer preferences, purchasing patterns, and engagement levels. This enables marketers to design targeted campaigns, personalize content, and enhance customer experiences. Data-driven insights also help in evaluating the effectiveness of marketing strategies, refining tactics, and improving overall marketing ROI.
Retailers leverage data analytics to enhance inventory management, optimize supply chains, and deliver personalized shopping experiences. By analyzing sales data, customer behavior, and market trends, retailers can forecast demand, manage stock levels, and implement effective pricing strategies. Data analytics helps in identifying high-performing products, optimizing promotional efforts, and improving customer loyalty.
Retailers use insights to personalize offers, improve store layouts, and enhance online shopping experiences. This data-driven approach supports operational efficiency and boosts profitability by aligning inventory with customer demand and market conditions.
In manufacturing, data analytics focuses on improving production efficiency, reducing equipment downtime, and ensuring quality control. By analyzing data from machinery and production processes, manufacturers can predict equipment failures, optimize maintenance schedules, and streamline operations.
Predictive analytics helps in identifying potential issues before they affect production, reducing unplanned downtime and improving overall equipment effectiveness (OEE). Data-driven insights also support supply chain management, demand forecasting, and process optimization, leading to cost savings and enhanced product quality.
E-commerce platforms use data analytics to drive sales, enhance user experiences, and optimize online strategies. By analyzing user behavior, transaction data, and website performance, e-commerce businesses can personalize product recommendations, improve site navigation, and manage inventory more effectively.
Data analytics helps in understanding customer preferences, identifying purchasing trends, and optimizing marketing efforts. Insights gained from data analysis enable e-commerce companies to design targeted promotions, improve customer engagement, and enhance the overall shopping experience, leading to increased conversion rates and customer satisfaction.
In the telecommunications sector, data analytics is crucial for managing network performance, optimizing customer service, and predicting customer churn. Telecom companies analyze network data to ensure reliable service, optimize bandwidth usage, and identify areas for network expansion. Predictive analytics helps in anticipating customer churn, allowing companies to implement retention strategies and improve customer satisfaction.
Data-driven insights support customer service operations by identifying common issues, streamlining support processes, and enhancing service delivery. Analytics also aids in designing personalized plans and offers, improving customer experience and loyalty.
Data analytics in education helps institutions enhance student learning outcomes, improve administrative efficiency, and optimize resource allocation. By analyzing student performance data, educators can identify areas where students may need additional support, tailor instructional methods, and track academic progress.
Data-driven insights support curriculum development, student engagement, and personalized learning experiences. Analytics also helps in optimizing institutional planning, managing resources, and improving administrative processes. By leveraging data, educational institutions can make informed decisions that contribute to student success and institutional effectiveness.
The future of data analytics is poised for transformative growth, driven by rapid advancements in technology and increasing data volumes. As organizations continue to recognize the value of data, the demand for sophisticated analytical tools and skilled professionals will escalate. Emerging technologies such as artificial intelligence, machine learning, and big data analytics are set to redefine how data is processed, analyzed, and utilized, enabling more accurate predictions and innovative solutions. The role of data analytics is expanding beyond traditional reporting to include advanced capabilities like predictive and prescriptive analytics, which provide deeper insights and actionable recommendations.
This shift will enhance decision-making processes, improve operational efficiency, and foster a competitive edge in various industries. As the field evolves, professionals will need to adapt by acquiring new skills and staying updated with the latest trends and tools. The continuous development in data analytics will not only open new career opportunities but also drive significant advancements in how organizations leverage data for strategic advantage, ensuring that data remains a central pillar of modern business success.
Copy and paste below code to page Head section
Data analytics involves the process of examining raw data with the purpose of concluding that information. It utilizes various techniques and tools to uncover patterns, trends, and insights that can inform decision-making and improve business performance.
A career in data analytics typically requires skills in data manipulation and analysis, proficiency with analytical tools and programming languages (like Excel, SQL, Python, and R), strong statistical knowledge, and the ability to communicate findings effectively. Familiarity with data visualization tools such as Tableau or Power BI is also beneficial.
The main types of data analytics are descriptive (summarizing past data), diagnostic (identifying the causes behind past events), predictive (forecasting future trends), and prescriptive (providing recommendations for actions). Each type serves a distinct purpose in understanding and leveraging data.
Businesses use data analytics to make informed decisions, optimize operations, enhance customer experiences, and drive strategic growth. It helps in identifying market trends, understanding customer behavior, improving efficiency, and measuring performance across various functions.
Data analytics offers numerous benefits, including improved decision-making, increased efficiency, cost savings, enhanced customer experiences, and competitive advantage. It enables organizations to make data-driven decisions, optimize processes, and uncover new opportunities for growth.
Data analytics is valuable across many industries, including finance, healthcare, marketing, retail, manufacturing, e-commerce, telecommunications, and education. Each sector leverages data analytics to address specific challenges and enhance performance in their respective fields.