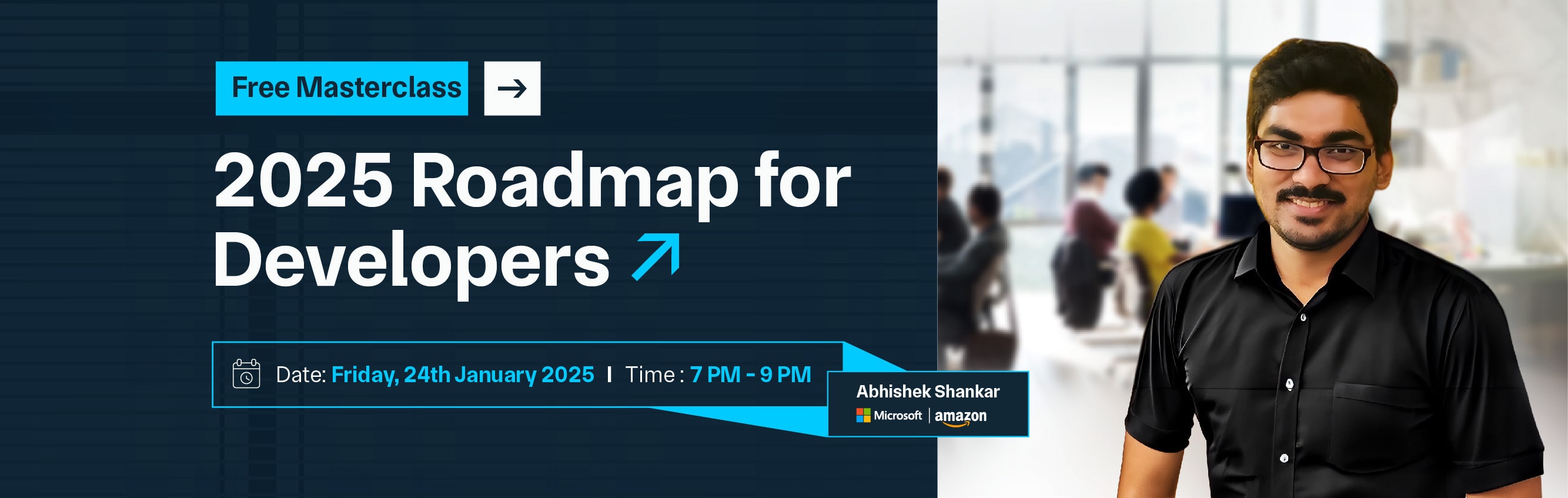

Edge Computing and Cloud Computing are two transformative technologies shaping the modern digital landscape, but they serve distinct purposes. Cloud Computing centralizes data processing in large data centers, allowing for extensive computational power and storage, making it ideal for handling massive data loads and complex analytics. Businesses use cloud services to scale up quickly and access vast resources without maintaining on-site hardware, which can be costly and time-consuming.
In contrast, Edge Computing decentralizes processing by bringing it closer to the data source, often at the “edge” of the network. This reduces latency and speeds up real-time data processing, as information doesn't have to travel to a central data center for every computation. Edge Computing is particularly beneficial in scenarios requiring immediate responses, such as IoT applications, autonomous vehicles, and remote monitoring systems. The choice between Edge and Cloud Computing depends on specific needs.
For tasks needing swift, localized processing, like video surveillance or real-time analytics, Edge Computing offers a faster, more efficient solution. Meanwhile, Cloud Computing suits applications with heavy processing demands or those needing remote access, such as online data backups and complex machine learning tasks. Each has unique strengths, and organizations are increasingly combining both approaches to create a hybrid model that balances performance, scalability, and flexibility.
Edge Computing is a distributed computing model that brings data processing closer to the source of data generation, such as IoT devices, sensors, or local servers. Instead of sending all the data to a centralized cloud server for processing, Edge Computing performs computations on-site or near the data source.
This proximity to data collection minimizes latency, enabling faster response times, which is crucial for applications that require real-time processing, such as autonomous vehicles, industrial automation, and smart cities. By processing data at or near the edge of the network, Edge Computing reduces the bandwidth requirements and alleviates the load on centralized data centers.
This approach is also more resilient to network disruptions because local processing can continue independently if a connection to the central cloud is temporarily lost. Edge Computing offers a more efficient, responsive, and reliable solution for scenarios where quick decision-making and reduced data transfer are essential.
Cloud Computing is a model that centralizes data storage and processing within large, remote data centers accessible over the Internet. It allows users to access and manage resources, such as computing power, storage, and software applications, from virtually anywhere. This scalability and flexibility have made Cloud Computing popular among businesses for handling extensive data processing, hosting applications, and managing complex analytics.
By using cloud services, companies can scale resources up or down as needed, eliminating the costs and logistics of maintaining physical hardware on-site. With Cloud Computing, organizations can offload their IT infrastructure to third-party providers, enabling remote collaboration, data storage, and disaster recovery solutions.
The cloud is ideal for applications requiring heavy computational resources, like big data analytics, artificial intelligence, and backup services. However, unlike Edge Computing, Cloud Computing relies on consistent network connectivity, and it may introduce latency due to data transmission to and from centralized data centers, making it less suitable for time-sensitive applications.
Edge Computing is rapidly transforming industries by offering local data processing, which reduces latency, enhances security, and improves efficiency. By bringing computing power closer to the data source, this model minimizes the need for constant communication with centralized cloud servers, resulting in faster response times and lower bandwidth usage.
These benefits make Edge Computing ideal for real-time applications and industries requiring quick, reliable data handling. Below are some key advantages of Edge Computing:
While Edge Computing offers many benefits, it also comes with notable challenges and limitations. Implementing a decentralized computing model requires substantial investment in infrastructure, as each device needs the capacity to handle processing and storage.
Additionally, managing security and reliability across a distributed network can be complex, and scalability may present difficulties. These disadvantages make Edge Computing less suitable for some applications. Below are some of the primary disadvantages of Edge Computing:
Cloud Computing has transformed how businesses operate by providing scalable, on-demand access to computing resources, including storage, processing power, and applications.
This model enables organizations to access sophisticated infrastructure without needing to invest in or maintain physical hardware, drastically reducing overhead costs and allowing for flexible, remote access.
By leveraging cloud services, companies can adapt to changing demands, scale operations seamlessly, and enhance collaboration. With robust security protocols and redundancy measures, Cloud Computing offers reliable data protection and disaster recovery capabilities. Below are the key advantages of Cloud Computing:
Despite its many advantages, Cloud Computing also has some notable drawbacks. Since it relies on internet connectivity, users may experience latency issues or downtime if there are network disruptions.
Additionally, while cloud providers offer robust security, entrusting sensitive data to an external provider involves potential security and compliance risks, especially in industries with strict data regulations.
Managing costs can also be challenging, as cloud expenses can increase significantly with extended usage or high resource demands. Below are some disadvantages of Cloud Computing:
Edge Computing and Cloud Computing are two distinct approaches to data processing that serve different purposes in the digital landscape. Cloud Computing centralizes processing and storage in remote servers, enabling organizations to access powerful resources and scale operations without investing in extensive infrastructure.
It is ideal for applications requiring extensive data analysis, long-term storage, and wide accessibility. Edge Computing, however, processes data closer to its source often on local devices or nearby servers enabling real-time responses and reducing latency. This approach is suited for scenarios where immediate processing is essential, such as IoT devices, autonomous vehicles, or industrial automation.
Both models have unique strengths and limitations, and understanding these differences helps businesses choose the best option based on their specific requirements. The table below outlines the key distinctions between Edge Computing and Cloud Computing:
Edge Computing and Cloud Computing are two fundamental models that handle data processing differently, addressing distinct needs in the tech landscape. Cloud Computing centralizes data in remote servers, which is ideal for large-scale data storage, analysis, and applications that don't require immediate processing.
Edge Computing, by contrast, processes data closer to its source, often on local devices, which minimizes latency and enhances real-time capabilities. This difference makes Edge Computing preferable for applications like IoT, autonomous vehicles, and AR/VR, where immediate responses are critical.
While Cloud Computing offers high scalability, it depends on reliable internet connectivity and can have higher latency. Here, we explore each model in detail to understand the unique benefits, limitations, and ideal use cases for each.
In Cloud Computing, data processing takes place in centralized data centers that are often located far from the data sources. These data centers are typically managed by large cloud providers such as AWS, Microsoft Azure, or Google Cloud. While this setup allows organizations to take advantage of powerful, scalable computational resources, the distance between the data source and the data center can introduce significant delays. This is particularly noticeable in situations where large volumes of data need to be processed in real time or when applications require fast feedback from the server.
In contrast, Edge Computing processes data locally, often at the source of the data or close to the edge of the network, such as on IoT devices or local edge servers. By processing data locally, Edge Computing minimizes the time it takes to analyze and act on the data, which is crucial for real-time decision-making. This local data processing eliminates the need for long-distance data transfers, reducing latency and providing faster responses. Edge Computing is ideal for applications like autonomous vehicles, industrial automation, and smart cities, where immediate action based on real-time data is critical.
In Cloud Computing, latency is typically higher because data must travel from the device to the central cloud server, which could be located thousands of miles away. The data then undergoes processing at the cloud server before being sent back to the device. This round-trip journey introduces a delay, which can be problematic for applications that demand instant or near-instant responses. For example, in virtual reality (VR), online gaming, or real-time monitoring systems, even minor delays can drastically degrade the user experience or compromise the efficiency of operations.
Edge Computing, on the other hand, significantly reduces latency by processing data as close to the source as possible, either on edge devices or local servers. With data being analyzed and acted upon at the edge, there is no need for long-distance communication between devices and distant cloud servers. As a result, Edge Computing provides near-instantaneous responses, making it well-suited for time-sensitive applications. Real-time applications such as autonomous driving, industrial robots, and medical monitoring systems benefit from the low latency offered by Edge Computing, as every millisecond of delay can have significant consequences.
Cloud Computing is ideally suited for applications that require large-scale computational power, extensive storage, and flexible scalability. For instance, businesses that need to process huge datasets, perform machine learning tasks, or manage enterprise software platforms benefit from the cloud's centralized resources. Cloud-based services like data warehousing, backup solutions, and customer relationship management (CRM) tools are common examples. The cloud’s ability to scale quickly and cost-effectively makes it perfect for organizations that need on-demand resources without the burden of maintaining expensive hardware.
Edge Computing, in contrast, is more suited for scenarios that require processing data in real-time or in remote locations where cloud connectivity might be unreliable. IoT devices, autonomous vehicles, industrial automation, and smart healthcare applications are prime examples where Edge Computing excels. These use cases require low latency, high reliability, and the ability to process data quickly on-site without relying on a central cloud server. Edge Computing is also useful in remote locations where the internet connection is sparse or unavailable, ensuring continuous operation even without consistent cloud connectivity.
In Cloud Computing, scalability is one of the biggest advantages. Cloud services allow businesses to quickly adjust their computational and storage resources based on demand without the need to invest in physical infrastructure. Cloud platforms like Amazon Web Services (AWS), Google Cloud, and Microsoft Azure offer easy-to-use scaling options, enabling businesses to increase or decrease their usage according to the fluctuating needs of their operations. The elasticity of Cloud Computing allows organizations to grow their infrastructure quickly as their demands change, making it a go-to solution for companies with unpredictable or seasonal workloads.
On the other hand, Edge Computing faces challenges when it comes to scalability. Since Edge Computing relies on local devices, such as edge servers or IoT devices, expanding the network requires adding more physical devices in various locations, which can be more complex and expensive. This decentralized nature can make it difficult to manage and scale the system effectively compared to the centralized model of the cloud. However, while scaling an Edge Computing solution can be challenging, it is highly effective for real-time applications in specific geographic regions, where localized processing is required to maintain low latency and reliability.
Cloud Computing typically requires large amounts of bandwidth, especially when processing or transmitting large datasets to and from the cloud. The data must travel over the internet to the cloud servers, where it is processed before being sent back to the device. This data transfer can lead to high bandwidth usage, especially in applications that require constant data streaming, such as video conferencing, cloud storage, or media distribution. Organizations that rely heavily on cloud services for continuous data transmission may face higher operational costs due to the increased demand for bandwidth, especially in areas with limited internet access or high bandwidth costs.
Edge Computing, by contrast, reduces bandwidth consumption by processing data locally at the edge of the network. Instead of sending raw data to the cloud for processing, only relevant or aggregated data is sent back, greatly minimizing the need for large-scale data transfers. This local processing eliminates the burden on internet bandwidth, making Edge Computing ideal for situations where large amounts of data need to be handled in real time. Still, full-scale cloud processing would need to be more efficient and practical. Edge Computing is particularly advantageous for remote environments, where internet connectivity may be limited or expensive.
Cloud Computing generally offers high reliability, as cloud providers invest heavily in infrastructure, redundancy, and disaster recovery systems. Many cloud services guarantee uptime percentages (e.g., 99.9% or higher), and providers often have multiple data centers spread across different regions to ensure that services remain available even if one data center fails. However, Cloud Computing depends entirely on internet connectivity, and if an internet connection fails or becomes unreliable, accessing cloud resources becomes impossible, which can impact business continuity.
Edge Computing provides higher reliability in situations where continuous operation is critical, even without a constant internet connection. Since data is processed locally on edge devices, the system can continue to function without relying on the cloud or external servers. In remote environments or areas with unreliable internet, Edge Computing ensures that data is processed and analyzed on-site without interruptions. This makes Edge Computing a better choice for industries like manufacturing, healthcare, and transportation, where operational downtime can lead to significant consequences.
Cloud Computing offers robust security features, such as encryption, firewalls, and regular security updates provided by cloud service providers. However, storing sensitive data off-site in remote data centers can raise concerns about data privacy and the potential for cyber-attacks. Cloud services are frequent targets of hackers due to the large volume of valuable data they store. Although cloud providers often adhere to strict security standards and protocols, customers must rely on them to ensure data protection. The privacy of data in Cloud Computing may also be affected by geographic regulations and legal concerns, especially when data is stored in different countries.
Edge Computing, with its localized data processing, offers enhanced security for certain applications. Since data is processed at or near the source, there is less risk of sensitive data being intercepted during transmission over the Internet. Additionally, Edge Computing allows businesses to maintain direct control over their data, which may be particularly important in regulated industries like healthcare, finance, or government. However, the decentralized nature of Edge Computing means that each edge device must be individually secured, making it more challenging to ensure consistent security across all devices in the network.
Cloud Computing is typically more cost-effective for businesses that require large-scale resources without the upfront investment in physical infrastructure. The pay-as-you-go model enables businesses to only pay for the resources they use, whether it's storage, computing power, or bandwidth. This makes Cloud Computing an attractive option for startups or businesses that experience fluctuating demand. However, as the amount of data increases or as more storage and computational power are needed, the costs can rise significantly, especially when there are high data transfer volumes or additional storage requirements.
Edge Computing, on the other hand, can incur higher upfront costs due to the need for physical devices, such as edge servers, IoT sensors, and local storage solutions. Organizations must invest in the hardware, maintenance, and management of these devices, which can make the initial investment relatively expensive. However, Edge Computing can offer long-term savings by reducing the need for large-scale data transfers to the cloud, as well as minimizing bandwidth consumption. For time-sensitive applications that require local data processing, the cost benefits of Edge Computing become more apparent as it reduces operational expenses related to cloud services.
Cloud Computing plays a critical role in enhancing the capabilities and efficiency of Edge AI as Edge AI involves processing data locally on devices like IoT sensors, cameras, or edge servers. Cloud Computing steps in to provide advanced computational power, scalability, and extensive storage when needed.
While Edge AI focuses on real-time decision-making with minimal latency, Cloud Computing supports it by offering heavy data processing, complex machine learning models, and large-scale data storage. Together, they create a seamless ecosystem for developing intelligent, autonomous systems.
Choosing between Edge Computing and Cloud Computing depends on various factors, including the need for real-time data processing, connectivity constraints, and the scale of data management.
Edge Computing is ideal when processing data locally at the device or sensor level is required, especially when latency is a concern. Cloud Computing is better suited for large-scale data storage and processing that doesn’t require immediate decision-making.
Each has its strengths and weaknesses, and understanding these differences can help businesses make more informed decisions about which technology to employ for specific use cases. The table below highlights key aspects to consider when deciding between Edge and Cloud Computing.
Edge Computing refers to the practice of processing data closer to its source rather than relying on a centralized data center. By placing computational resources at the "edge" of the network near devices like sensors, cameras, and other IoT equipment, Edge Computing enables quicker decision-making and faster response times. This is particularly important for applications requiring low-latency, real-time data processing, such as autonomous vehicles, industrial automation, and remote monitoring systems.
The proximity to data sources allows these systems to perform analysis and decision-making locally, reducing the need for constant data transmission to centralized servers and minimizing the time it takes to act on data insights. One of the key benefits of Edge Computing is its ability to function in environments with limited or intermittent connectivity.
Since processing can occur locally, Edge devices can operate independently without relying on continuous internet access, making it ideal for remote locations or situations with unreliable networks. Furthermore, Edge Computing reduces bandwidth consumption by processing only essential data locally and sending back the necessary insights to the cloud or central systems. This improves system efficiency, reduces operational costs, and minimizes the dependency on centralized infrastructure.
Cloud Computing provides centralized computing power and resources, allowing organizations to store vast amounts of data, run complex applications, and perform heavy computational tasks remotely. With cloud platforms like Amazon Web Services (AWS), Microsoft Azure, and Google Cloud, users gain access to virtually limitless storage and processing capabilities without the need for on-premises infrastructure.
Cloud computing excels in handling large-scale data processing, machine learning model training, and enterprise-level data analytics, making it a versatile solution for businesses with substantial data and computational needs. Unlike Edge Computing, which focuses on local processing, Cloud Computing relies on powerful remote data centers that can scale dynamically based on demand. One of the major advantages of Cloud Computing is its scalability. Businesses can quickly adjust their resources to meet changing demands without worrying about hardware limitations.
This makes Cloud Computing ideal for industries that require flexible, on-demand resources, such as big data analytics, backup and disaster recovery, and web hosting. Additionally, Cloud Computing enables easy collaboration and access to data from anywhere in the world, provided there is an internet connection. However, while it offers powerful capabilities, it can sometimes introduce latency issues for real-time applications and requires continuous internet connectivity, making it less suitable for scenarios where low-latency processing is a priority.
The future of the IT sector is set to be shaped by rapid advancements in technology, transforming industries and everyday life. Innovations such as Artificial Intelligence (AI), Machine Learning (ML), Edge Computing, and 5G are already playing a significant role in revolutionizing business operations and customer experiences.
As we look to the future, IT professionals will face new challenges and opportunities as these technologies evolve. The increasing demand for cybersecurity, data privacy, and the integration of emerging technologies will further define the direction of the industry. Here are some key trends that are likely to shape the future of IT:
As both Edge Computing and Cloud Computing become increasingly prevalent, each comes with its own set of challenges that need to be addressed for their successful implementation. While Edge Computing brings data processing closer to the source, ensuring faster responses and lower latency, it faces hurdles related to infrastructure, management, and scalability.
On the other hand, Cloud Computing offers vast computational power and storage but presents challenges concerning data security, connectivity, and ongoing costs. Understanding these challenges is essential for organizations to leverage the best of both technologies while mitigating potential risks. Below, we explore the key challenges associated with both.
Challenges of Edge Computing:
Challenges of Cloud Computing:
"Labor at the edge" refers to the work done in Edge Computing environments where data is processed and analyzed close to the data source rather than being sent to a centralized cloud server or data center. This approach is becoming increasingly important as the demand for real-time data processing grows, particularly in industries like manufacturing, healthcare, automotive, and retail.
Instead of relying on the cloud to handle all data processing tasks, devices and sensors located at the "edge" of the network perform computation locally. By doing so, these systems can quickly analyze data, make decisions, and initiate actions without the latency associated with transferring data to remote servers. This "edge labor" enables faster responses and more efficient processing in scenarios where speed is critical, such as autonomous driving or industrial automation. The term "labor at the edge" also reflects the need for skilled professionals who can manage and maintain Edge Computing systems.
This includes deploying and optimizing edge devices, ensuring secure communication between local and remote systems, and managing the overall infrastructure; according to a recent report by MarketsandMarkets, the global Edge Computing market is expected to grow from $15.7 billion in 2023 to $50.6 billion by 2028, demonstrating the increasing reliance on edge computing across various sectors. As the need for low-latency data processing and real-time decision-making continues to rise, labor at the edge will become a key component in the future of technology and innovation.
Deciding between Edge Computing and Cloud Computing depends on the specific needs of an organization or application. Edge Computing is ideal for situations where low latency and real-time data processing are critical. By processing data at the source, Edge Computing minimizes delays and bandwidth usage, enabling immediate actions based on real-time data.
This is especially useful in applications such as autonomous vehicles, industrial automation, and remote healthcare services, where real-time decision-making is crucial. However, Edge Computing comes with challenges like the need for distributed infrastructure, increased security concerns, and limited computational resources at the edge. On the other hand, Cloud Computing excels in providing scalable storage, advanced computational power, and centralized data management.
It is well-suited for applications that require large-scale data storage, complex analytics, and the ability to scale up or down based on demand. Cloud services offer flexibility, cost-efficiency, and robust security protocols. However, Cloud Computing can face latency issues due to the distance data must travel and may not be the best option for real-time processing. Ultimately, the choice between Edge and Cloud Computing depends on the use case, with some organizations opting for a hybrid approach that combines the strengths of both technologies.
To conclude, both Edge Computing and Cloud Computing offer distinct advantages, each catering to specific needs. Edge Computing is optimal for situations requiring low-latency, real-time data processing at the source, making it ideal for applications like autonomous systems, smart cities, and healthcare monitoring. However, managing distributed infrastructure and ensuring security across remote devices can be challenging.
Cloud Computing, in contrast, is perfect for businesses that require powerful, scalable computing resources for data storage, complex analytics, and long-term processing. It provides centralized control, flexibility, and cost-effectiveness but may only be suitable for applications with flexible latency requirements. Ultimately, a hybrid approach, blending Edge and Cloud Computing, can provide the best solution, ensuring performance optimization while addressing scalability and security needs across industries.
Copy and paste below code to page Head section
Edge Computing processes data near its source, reducing latency and bandwidth usage, while Cloud Computing involves centralizing data processing in remote servers. Edge is best for real-time tasks, and Cloud offers scalability and centralized resources for large data processing, each serving distinct purposes depending on the application.
Edge Computing is preferred when low latency, real-time data processing, and local decision-making are required. Applications such as autonomous vehicles, industrial automation, and healthcare monitoring benefit from Edge, as it minimizes delays and reduces reliance on cloud servers for immediate data responses.
Cloud Computing offers scalability, flexibility, and cost-efficiency. It allows organizations to access virtually unlimited storage and computational power without managing physical infrastructure. With centralized management and advanced security protocols, Cloud services enable efficient data processing, analytics, and seamless scaling as business needs grow.
By processing data locally at the source, Edge Computing minimizes the distance data needs to travel, significantly reducing delays. This allows for faster response times in applications requiring immediate action, such as autonomous vehicles or real-time healthcare diagnostics, ensuring quicker decision-making without relying on remote servers.
Yes, a hybrid approach combining Edge Computing and Cloud Computing can be highly effective. Edge Computing can handle real-time, low-latency tasks, while Cloud Computing can provide centralized storage, analytics, and long-term data processing. This integrated model ensures optimal performance, scalability, and cost efficiency across various applications.
Edge Computing involves processing data at decentralized locations, which can increase exposure to security risks. Protecting data at the edge requires robust encryption, secure communication channels, and regular monitoring. Ensuring that devices are secure and that data privacy is maintained across multiple locations is a key challenge.