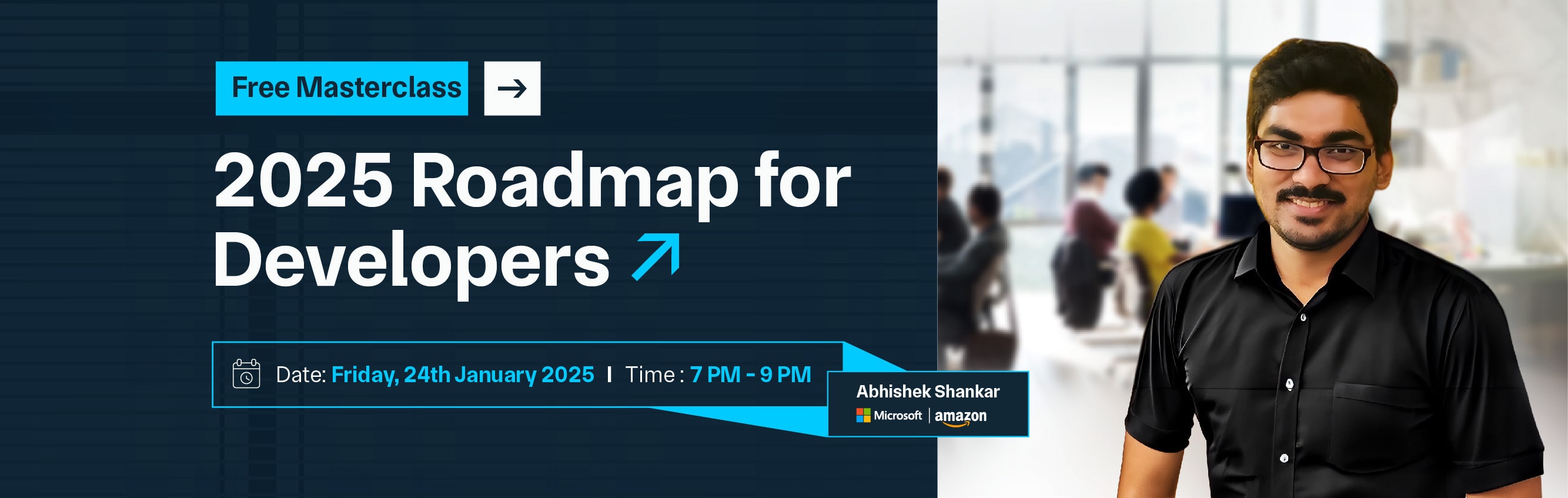

Exploratory Data Analysis (EDA) is a crucial initial step in any data science project, where the main goal is to understand the data before diving into more complex modelling. It involves a variety of techniques to summarise the main characteristics of the data, often using visual methods such as histograms, box plots, and scatter plots. During EDA, the primary objectives include identifying patterns, uncovering underlying relationships, and detecting outliers or anomalies that may require special attention.
By examining distributions and summary statistics, data scientists can make informed decisions about data preprocessing steps such as normalisation or handling missing values. Furthermore, EDA helps in selecting appropriate models and defining feature engineering strategies based on insights gained about the relationships between variables. It also plays a crucial role in communicating findings to stakeholders, as visual representations and concise summaries derived from EDA can clarify complex data structures.
In essence, EDA serves as the foundation for building accurate and reliable predictive models in data science by ensuring that data quality is adequate for subsequent analysis and modelling phases. It is not merely a preliminary task but a critical process that shapes the entire data science workflow, guiding decisions at every stage, from preprocessing to model interpretation.
Exploratory Data Analysis (EDA) is a fundamental approach in data science that involves exploring and summarising data sets to understand their main characteristics. It aims to uncover patterns, relationships, anomalies, and key insights that can guide further analysis and decision-making in the data science workflow.
EDA typically begins with a descriptive overview of the data using summary statistics such as mean, median, standard deviation, and quartiles. Visualisation plays a crucial role in EDA, where graphs like histograms, box plots, scatter plots, and heatmaps help in understanding the distribution, spread, and correlations between variables.
Student Performance
Objective: Analyze factors influencing student grades.
1. Data Loading and Understanding:
2. Summary Statistics:
3. Visualization:
4. Data Cleaning:
5. Insights:
6. Next Steps:
In this example, EDA helps understand the dataset's characteristics and relationships between variables, laying the groundwork for further analysis and modeling in the data science process.
EDA is not just a preliminary step but an iterative process that informs subsequent steps in the data science pipeline, such as model selection, validation, and interpretation of results.
It ensures that data scientists have a solid understanding of the data's structure and behavior, enabling them to build more accurate and effective models for prediction or decision-making purposes.
Exploratory Data Analysis (EDA) is a preliminary step in data analysis that involves summarising the main characteristics of a dataset. It includes techniques to understand data distributions, identify patterns, detect anomalies, and explore relationships between variables. EDA plays a crucial role in uncovering insights, validating assumptions, and guiding further data exploration and modeling decisions in data science projects.
Overall, EDA serves as a critical foundation in data science projects, ensuring data integrity, informing modeling decisions, and empowering stakeholders with actionable insights derived from data exploration.
Exploratory Data Analysis (EDA) is a critical initial step in data analysis that involves examining and visualizing data sets to summarize their main characteristics. It helps analysts understand data distributions, detect patterns, identify outliers, and explore relationships between variables.
EDA techniques include visual methods like histograms and scatter plots, as well as statistical measures such as mean, median, and correlation coefficients. By uncovering insights and understanding the structure of the data, EDA guides further analysis and decision-making in data science and machine learning projects.
This type focuses on analyzing a single variable at a time to understand its distribution and characteristics. It helps in identifying outliers and understanding central tendency (mean, median, mode), spread (variance, range), and shape of the data distribution. Visual tools like histograms and box plots are commonly used to visualize univariate data, providing insights into data quality and initial patterns.
Bivariate analysis explores relationships between two variables simultaneously. It helps in understanding how one variable behaves with respect to another. Common techniques include scatter plots to visualize correlations, correlation coefficients (e.g., Pearson's correlation) to quantify relationships, and contingency tables for categorical variables. Bivariate analysis is essential for identifying dependencies and potential interactions between variables in a dataset.
This type examines relationships between multiple variables at once. Techniques like principal component analysis (PCA), factor analysis, and multidimensional scaling help in reducing the dimensionality of data while preserving important information.
Multivariate analysis is crucial for uncovering complex patterns and understanding interactions among several variables simultaneously, facilitating deeper insights into data structure and variability.
Graphical representations such as histograms, scatter plots, heatmaps, and bar charts are fundamental in EDA. They provide intuitive visual summaries of data distributions, relationships, and trends.
Visualization techniques aid in identifying outliers, patterns, clusters, and correlations within datasets, making complex data more accessible and interpretable for stakeholders and decision-makers.
Summary statistics encompass numerical measures that summarize the central tendency, dispersion, and shape of data distributions. Key statistics include mean, median, mode, standard deviation, skewness, and kurtosis.
These measures provide quantitative insights into data characteristics, helping to detect anomalies, assess data quality, and make informed decisions about further analysis or preprocessing steps in data science projects.
This technique involves reducing the number of variables in a dataset while retaining essential information. Methods like PCA and t-SNE transform high-dimensional data into lower-dimensional representations, facilitating easier visualization and analysis.
Dimensionality reduction is useful for simplifying complex datasets, identifying key features for modeling, and improving computational efficiency without losing critical patterns or relationships present in the data.
Outlier detection identifies data points that significantly differ from the majority of the dataset. Techniques such as z-score, IQR (Interquartile Range), and clustering-based methods help in detecting and handling outliers.
Addressing outliers is crucial for ensuring data quality, preventing biased analysis or model performance, and understanding potential anomalies or errors in the dataset.
Feature engineering involves creating new features or transforming existing ones based on insights gained from EDA. It aims to improve predictive model performance by enhancing the relevance, representation, or interpretability of features.
Techniques include encoding categorical variables, scaling numerical features, creating interaction terms, or deriving new features from existing ones. Effective feature engineering leverages EDA findings to optimize feature selection and preprocessing steps, leading to more accurate and robust machine learning models.
A specialized technique within Exploratory Data Analysis (EDA) is Principal Component Analysis (PCA). PCA is used to reduce the dimensionality of a dataset while retaining as much variability as possible. Here’s a brief overview:
PCA transforms a dataset into a set of orthogonal components that are linear combinations of the original variables. These components, called principal components, are ordered by the amount of variance they explain in the data. By reducing the number of dimensions, PCA simplifies the dataset and facilitates visualization and analysis.
PCA is a specialized EDA technique that plays a crucial role in data preprocessing and exploration, especially for datasets with high dimensionality or complex relationships between variables.
Exploratory Data Analysis (EDA) involves using various techniques and tools to explore and understand datasets initially. It focuses on summarizing main characteristics, detecting patterns, identifying outliers, and exploring relationships between variables.
EDA plays a crucial role in data science by providing insights that guide further analysis, preprocessing, and modeling decisions. It uses methods like statistical summaries, visualizations (e.g., histograms, scatter plots), and software tools (e.g., Python's Pandas, R's ggplot2) to uncover hidden patterns and ensure data quality before diving into more complex analyses or modeling tasks.
There are several tools commonly used for performing Exploratory Data Analysis (EDA) in data science projects. Here are some popular ones.
These tools provide a range of capabilities for data exploration, visualization, statistical analysis, and machine learning, catering to different needs and preferences in the data science community.
Exploratory Data Analysis (EDA) is a critical initial step in any data science project. It involves systematically analyzing and visualizing data to uncover patterns, spot anomalies, and extract meaningful insights. By understanding the dataset's structure and relationships between variables, EDA helps data scientists make informed decisions about data preprocessing, feature engineering, and modelling strategies.
Through techniques like statistical summaries, visualizations, and hypothesis testing, EDA ensures data quality and lays a solid foundation for more advanced analyses. Ultimately, EDA plays a vital role in transforming raw data into actionable insights that drive business decisions and scientific discoveries.
You emphasize gathering data from various sources such as databases, files, and APIs, which is crucial for comprehensive analysis. Ensuring data integrity through checks for completeness, accuracy, and reliability sets a strong foundation for reliable insights.
Importance: Proper data collection ensures that the subsequent analysis is based on accurate and reliable information, laying the foundation for meaningful insights.
Addressing missing values, outliers, and inconsistencies is essential for preparing the data for analysis. Techniques like imputation and outlier detection help in maintaining data quality, reducing bias in subsequent analyses.
Importance: Clean data reduces bias and errors in subsequent analyses, improving the reliability and accuracy of insights derived.
Analyzing dataset structures, distributions, and relationships between variables provides initial insights into the data. Techniques like univariate, bivariate, and multivariate analysis help in understanding patterns and correlations within the data.
Importance: Data exploration uncovers patterns, trends, and relationships that guide further analysis and modeling decisions.
Creating visual summaries using libraries like Matplotlib, Seaborn, or Plotly enhances the clarity and effectiveness of presenting findings. Customizing visualizations to highlight key insights aids in communicating complex information to stakeholders.
Importance: Visualizations simplify complex data into intuitive formats, aiding in communication of findings to stakeholders.
Deriving new features or transforming existing ones optimizes the data for modelling purposes. Effective feature engineering contributes significantly to improving model accuracy and performance.
Importance: Effective feature engineering improves model accuracy and performance by providing better input variables.
Validating assumptions and findings through statistical tests ensures the robustness of conclusions drawn from the data. Techniques like hypothesis testing and assumption validation provide rigour and confidence in interpreting data patterns.
Importance: Statistical analysis provides rigor and confidence in interpreting data patterns and relationships.
Extracting meaningful insights and patterns from analysis results is crucial for deriving actionable recommendations.Summarizing and interpreting findings within the context of business objectives helps stakeholders make informed decisions.
Importance: Interpretation bridges data analysis with actionable recommendations, driving informed decision-making.
Documenting the entire exploratory data analysis (EDA) process, findings, and visualizations ensures transparency and reproducibility. Comprehensive reports and presentations facilitate effective communication of insight.
Importance: Documentation ensures transparency, reproducibility, and clarity in conveying insights and recommendations to stakeholders.
Exploratory Data Analysis (EDA) plays a pivotal role in data science. It serves as the initial stage where analysts delve into data to understand its structure, uncover patterns, and identify relationships between variables. EDA helps in detecting anomalies, assessing data quality, and guiding decisions on preprocessing and feature engineering.
By visualizing and summarizing data through statistical methods and graphical tools, EDA enables data scientists to derive actionable insights that inform subsequent modeling and analysis tasks. Ultimately, EDA empowers data-driven decision-making, enhances model performance, and ensures the reliability of findings in various domains of data science.
In essence, EDA plays a foundational role in data science by facilitating thorough exploration, understanding, and preparation of data, ultimately enabling more accurate and reliable analyses and predictions.
The primary objective of Exploratory Data Analysis (EDA) is to analyze and investigate data sets to summarize their main characteristics, often employing visual methods. EDA aims to uncover underlying patterns, trends, relationships, and anomalies in the data through a combination of statistical summaries and graphical representations.
By doing so, EDA facilitates the understanding of data properties and informs subsequent steps in the data analysis process, such as data preprocessing, feature selection, and modeling. Ultimately, the goal of EDA is to extract meaningful insights and hypotheses that guide further investigation and decision-making in data science and analytics projects.
Exploratory Data Analysis (EDA) offers numerous advantages in data science by providing a foundational understanding of datasets. It helps in identifying patterns, outliers, and relationships, ensuring data quality and guiding subsequent analysis and decision-making.
EDA also facilitates hypothesis generation, feature selection, and effective communication of insights to stakeholders. By uncovering hidden trends and anomalies, EDA enhances the efficiency and reliability of data-driven projects, making it an essential step in extracting actionable insights from raw data.
Overall, EDA serves as a crucial exploratory phase that enhances understanding, improves data quality, and supports decision-making in data-driven endeavors.
Exploratory Data Analysis (EDA) serves as a critical gateway in the realm of data science, offering indispensable insights and benefits. By systematically exploring and visualizing data, EDA unveils hidden patterns, anomalies, and relationships that lay the groundwork for informed decision-making. It ensures data quality, aids in hypothesis generation, and guides feature selection and engineering processes.
Moreover, EDA facilitates effective communication of findings to stakeholders, enhancing collaboration and understanding across teams. Ultimately, EDA empowers data scientists to derive meaningful insights, optimize modeling efforts, and drive impactful outcomes in diverse fields—from business intelligence to scientific research. Thus, EDA stands as a cornerstone in leveraging data to uncover knowledge and drive innovation.
Copy and paste below code to page Head section
Exploratory Data Analysis (EDA) is a preliminary approach to analyzing data sets to summarize their main characteristics using visual methods and statistical summaries. It helps in understanding data distributions, identifying patterns, and exploring relationships between variables.
EDA is crucial in data science as it helps detect anomalies, assess data quality, and understand the structure and nature of the data. It informs data preprocessing, feature engineering, and modeling decisions, leading to more accurate and reliable analyses and predictions.
The primary objectives of EDA include: Identifying patterns, trends, and relationships in the data. Assessing data quality and detecting anomalies or outliers. Formulating hypotheses and guiding further analysis. Communicating insights effectively to stakeholders.
EDA employs various techniques such as: Univariate analysis (histograms, box plots) to analyze single variables. Bivariate analysis (scatter plots, correlation analysis) to explore relationships between pairs of variables. Multivariate analysis (PCA, clustering) to examine interactions among multiple variables. Statistical tests (hypothesis testing) to validate assumptions and findings.
EDA contributes to decision-making by providing actionable insights derived from data exploration. It helps stakeholders understand trends, make informed decisions, and prioritize strategies based on evidence-backed findings.
Popular tools for EDA include programming languages like Python (using libraries such as Pandas, Matplotlib, and Seaborn) and R (using ggplot2, dplyr), as well as interactive tools like Tableau and Power BI for visualization and exploration.