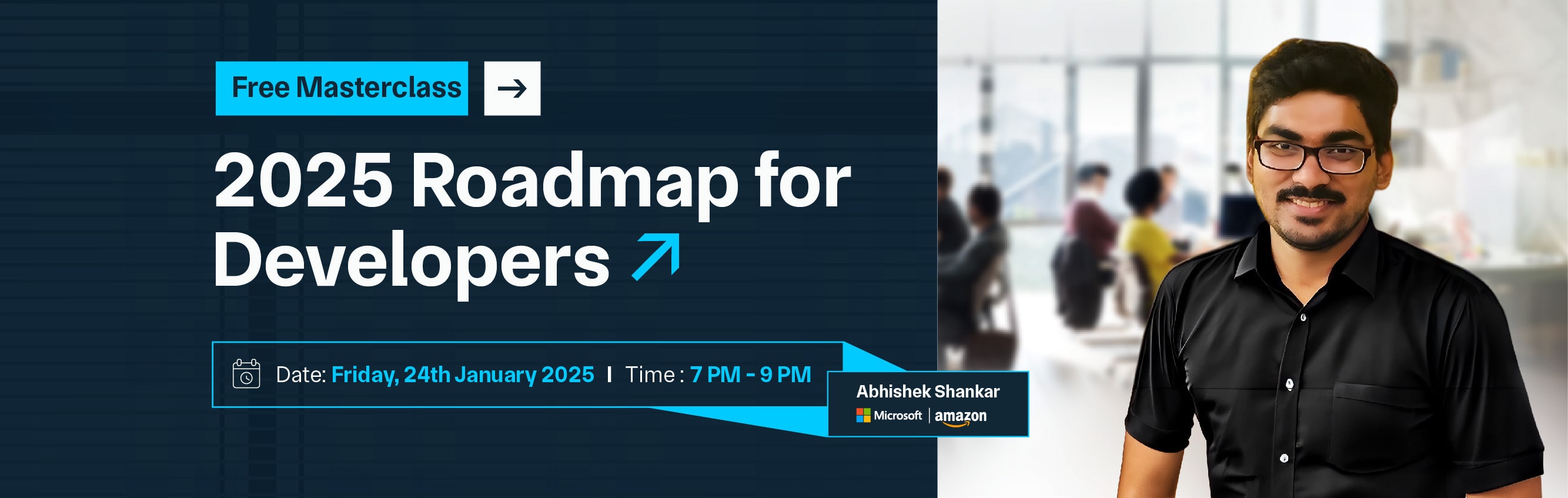

Data Science and Artificial Intelligence (AI) are two rapidly evolving fields that are shaping the modern digital world. While they often overlap in their goals and applications, they serve distinct purposes and require unique skill sets. Data Science focuses on extracting insights and knowledge from structured and unstructured data through statistical analysis, machine learning, and visualization techniques. It revolves around understanding patterns and trends in data to aid decision-making in various industries.
On the other hand, Artificial Intelligence is about creating intelligent systems capable of mimicking human thought processes and behaviors. AI encompasses technologies like machine learning, natural language processing, and robotics. The primary aim of AI is to enable machines to perform tasks that usually require human intelligence, such as problem-solving, decision-making, and language comprehension. AI systems are often built using the outputs of data science, making the two fields interdependent.
Despite their similarities, the key difference lies in their objectives. Data Science focuses on analyzing and interpreting data to generate actionable insights, while AI emphasizes creating systems that can act autonomously. Understanding these distinctions is crucial for businesses and professionals as they leverage these technologies to drive innovation and growth in various domains, including healthcare, finance, and education.
Data Science is a multidisciplinary field focused on analyzing and interpreting complex data to derive valuable insights. It involves the use of statistics, mathematics, programming, and machine learning to identify patterns and trends in structured and unstructured data. Data scientists work with large datasets, leveraging tools and algorithms to clean, process, and visualize information, enabling businesses to make data-driven decisions.
This field is widely applied across industries like finance, healthcare, and marketing to optimize strategies and solve real-world problems. The core of Data Science lies in its ability to uncover hidden insights that drive innovation and efficiency.
By combining domain knowledge with technical expertise, data scientists can predict outcomes, automate processes, and improve performance. Its scope extends beyond traditional analytics, as it integrates advanced techniques like deep learning and predictive modeling to solve complex challenges in today’s data-centric world.
Data Science is an interdisciplinary field that combines expertise in statistics, programming, and domain knowledge to process and analyze vast amounts of data. It aims to uncover hidden patterns, generate insights, and drive informed decision-making.
From collecting raw data to implementing actionable solutions, Data Science plays a crucial role in solving real-world problems and optimizing operations across industries.
Its applications extend from predictive analytics to AI and machine learning, making it a cornerstone for innovation and strategic growth. Below are the key components that define and contribute to the success of Data Science:
Data Science has become a transformative force in today’s data-driven world, enabling organizations to harness the power of vast datasets to make strategic decisions. By employing statistical techniques, machine learning, and domain expertise, Data Science uncovers patterns, trends, and actionable insights that drive business growth and innovation.
Its applications span diverse industries such as healthcare, finance, retail, and law enforcement, each benefiting from its ability to solve complex challenges and predict outcomes with precision. From optimizing supply chains to enhancing customer personalization, Data Science has a profound impact on improving efficiency, reducing costs, and ensuring competitive advantages.
By transforming raw data into actionable insights, it empowers industries to make informed decisions, innovate solutions, and address global challenges. Below are ten major applications of Data Science, explained in detail:
Data Science empowers businesses to analyze large customer datasets, enabling effective segmentation and the creation of highly targeted marketing strategies. By applying techniques like clustering and predictive analytics, organizations can group customers based on demographics, behaviors, and preferences. This segmentation helps companies craft personalized marketing campaigns that drive engagement, increase customer loyalty, and improve overall satisfaction.
Personalization extends to recommendations powered by advanced algorithms, which help businesses enhance user experience across various platforms, such as e-commerce and entertainment services. Furthermore, customer segmentation provides valuable insights into emerging market trends, consumer preferences, and purchasing behaviors.
This allows businesses to refine their product offerings, ensuring that they meet customer expectations more effectively. By focusing on high-value customers and their specific needs, companies can allocate resources more efficiently, ensuring better returns on marketing investments and optimizing customer acquisition strategies. This approach not only enhances customer experiences but also drives long-term business growth and profitability.
Predictive analytics is revolutionizing healthcare by enabling professionals to identify risks and make informed decisions about patient care. By analyzing vast amounts of clinical data, genetic information, and patient histories, Data Science models can predict the likelihood of individuals developing certain diseases. This predictive capability allows healthcare providers to recommend preventive measures and early interventions, improving health outcomes and reducing the overall cost of care.
Additionally, predictive models enhance treatment planning by forecasting how patients will respond to specific therapies, leading to more effective and personalized healthcare solutions. Data Science also plays a significant role in advancing medical research, such as drug discovery and clinical trials. Machine learning algorithms can analyze massive datasets to identify patterns that accelerate the development of new treatments and improve diagnostic accuracy.
By applying these techniques, healthcare professionals can streamline patient monitoring, optimize resource allocation, and enhance the overall quality of care. As a result, Data Science is helping to make healthcare more efficient, affordable, and accessible, while also driving innovation in medical practices.
Data Science is pivotal in detecting and preventing fraudulent activities, particularly within the financial sector. Financial institutions use advanced machine learning algorithms to analyze transaction data in real time, identifying unusual patterns and flagging potential fraudulent actions. These systems continuously learn from new data, adapting to emerging fraud techniques and enhancing the effectiveness of detection.
By spotting anomalies quickly, financial institutions can mitigate risks, protect customers from losses, and maintain operational security. Beyond real-time detection, Data Science also supports the development of proactive fraud prevention strategies. By analyzing historical transaction data, institutions can establish thresholds and enhance verification processes, preventing fraud before it occurs.
These predictive models help reduce financial losses and bolster trust between businesses and their customers. With Data Science driving improvements in fraud detection, financial systems can maintain a higher level of security, ensuring customer confidence and reducing the risk of future incidents.
Supply chain optimization is a key area where Data Science is making a significant impact. By leveraging predictive analytics, businesses can enhance demand forecasting, improve inventory management, and streamline logistics. Analyzing historical data allows companies to predict customer demand more accurately, ensuring that products are available when needed while minimizing overstock and waste.
This data-driven approach improves efficiency, reduces costs, and increases the ability to respond quickly to market changes. Data Science also supports route optimization, a critical component of supply chain management. By analyzing various factors, such as traffic patterns, weather conditions, and delivery timeframes, companies can optimize delivery routes, reducing fuel consumption and improving delivery times.
These insights help businesses make informed decisions, ensuring that resources are used efficiently, costs are minimized, and customer satisfaction is maximized. As supply chains become increasingly complex, Data Science will continue to be a vital tool for optimizing operations and ensuring business success.
Social media platforms generate an overwhelming amount of data daily, and Data Science is key to transforming this data into actionable insights for businesses. By analyzing user engagement, sentiment, and trending topics, organizations can design marketing campaigns that resonate with their target audience. Social media analytics also helps businesses identify influencers, track brand mentions, and analyze competitor strategies.
This allows companies to refine their marketing approach, improve customer engagement, and enhance brand visibility across social platforms. Beyond marketing, social media analytics provides valuable information about customer preferences and market trends. By studying patterns in user behavior and sentiment, businesses can gain deeper insights into consumer needs and adjust their strategies accordingly.
This data-driven approach allows companies to stay competitive, ensuring that they not only meet customer expectations but also anticipate shifts in market dynamics. As social media continues to play a central role in modern business, Data Science will remain essential for leveraging this vast source of information.
Data Science plays a crucial role in product development by providing valuable insights into market trends, consumer preferences, and competitors' offerings. By analyzing customer feedback, purchasing behavior, and industry trends, companies can identify gaps in the market and create products that meet customer demands more effectively.
Data-driven product development ensures that businesses stay aligned with consumer expectations, reducing the risk of product failure and enhancing the chances of market success. Additionally, Data Science aids in the innovation process by predicting future trends and consumer needs. Through advanced modeling techniques, businesses can forecast shifts in market dynamics, enabling them to develop products that are ahead of the curve.
By testing prototypes and analyzing customer responses, companies can refine their offerings and ensure they align with user preferences. This data-centric approach supports continuous innovation, helping businesses maintain a competitive edge in a rapidly evolving market.
Data Science is transforming the way financial institutions assess and manage risk. By analyzing historical financial data, market trends, and economic indicators, predictive models can assess the likelihood of defaults, identify high-risk investments, and forecast market volatility.
These insights allow businesses to make informed decisions regarding investments, loans, and portfolio management, minimizing financial risks and maximizing returns. Data Science also supports regulatory compliance by helping institutions monitor transactions, detect anomalies, and ensure that all financial activities align with legal requirements.
By utilizing predictive analytics, businesses can anticipate potential risks and take preventive measures to protect their assets and reputation. With Data Science providing deeper insights into risk factors, financial institutions can enhance decision-making processes, ensuring long-term stability and minimizing exposure to financial losses.
Data Science is revolutionizing energy consumption management by providing valuable insights into consumption patterns and demand fluctuations. By analyzing data from IoT devices, smart grids, and energy meters, companies can predict energy demand more accurately, ensuring optimal energy distribution and minimizing waste.
This data-driven approach enables energy providers to optimize their operations, reducing operational costs while improving service efficiency. Moreover, Data Science supports renewable energy initiatives by identifying the most efficient ways to integrate green energy sources into existing energy grids. By analyzing factors such as weather patterns, energy generation capacity, and grid stability, businesses can ensure that renewable energy is used effectively, contributing to sustainability goals.
This optimization process helps reduce reliance on non-renewable sources, fostering a more eco-friendly energy landscape and supporting long-term cost savings for both providers and consumers.
In the e-commerce industry, recommendation engines powered by Data Science significantly enhance the customer shopping experience. By analyzing customer behavior, purchase history, and preferences, these systems suggest personalized products, increasing customer satisfaction and driving sales. Recommendation algorithms ensure that shoppers find relevant products quickly, improving the overall shopping experience and increasing the likelihood of repeat purchases.
Data Science also helps e-commerce businesses improve inventory management by providing insights into product demand. By predicting which items are likely to be popular, companies can better plan their stock levels, reducing overstock and stockouts.
Additionally, customer data enables businesses to design targeted marketing campaigns, ensuring that promotions and advertisements reach the right audience at the right time. With these data-driven insights, e-commerce platforms can optimize their operations and improve both customer engagement and revenue.
Data Science is playing a pivotal role in law enforcement by predicting crime hotspots and enabling proactive resource allocation. By analyzing historical crime data, demographic information, and socio-economic factors, predictive models can identify areas with a high likelihood of criminal activity.
This allows law enforcement agencies to deploy resources more effectively, preventing crime before it occurs and enhancing public safety. Additionally, Data Science supports criminal investigations by analyzing evidence, tracking suspects, and identifying patterns in criminal behavior.
These advanced analytics help law enforcement agencies solve cases faster, improving case closure rates and ensuring that justice is served. The use of Data Science in law enforcement not only strengthens crime prevention efforts but also builds trust between communities and the police, ensuring a safer and more secure environment for all.
Artificial Intelligence (AI) is a branch of computer science dedicated to creating intelligent systems capable of performing tasks that typically require human intelligence. It encompasses technologies like machine learning, natural language processing, and computer vision to enable machines to learn from data, make decisions, and interact in human-like ways.
AI applications range from virtual assistants and chatbots to autonomous vehicles and predictive analytics. The primary goal of AI is to replicate cognitive functions, allowing machines to understand, reason, and respond intelligently. Unlike traditional software, AI systems improve over time through learning from experiences and adapting to new information.
This transformative technology is reshaping industries by automating repetitive tasks, enhancing customer experiences, and enabling innovations that were once considered science fiction.
Artificial Intelligence (AI) is a revolutionary field focused on creating systems capable of mimicking human intelligence and performing tasks such as learning, reasoning, and decision-making. It integrates cutting-edge technologies like machine learning, neural networks, and robotics to enable machines to operate autonomously.
AI has become a transformative force in industries from healthcare and finance to autonomous vehicles and entertainment. Below are the critical components that define AI and its far-reaching capabilities:
Artificial Intelligence (AI) has emerged as a transformative technology with vast potential across multiple industries. Its applications span from healthcare and finance to transportation and entertainment, revolutionizing how businesses operate and enhancing user experiences. AI leverages machine learning, natural language processing, and neural networks to analyze complex data, predict outcomes, and automate tasks, making processes faster, more efficient, and highly accurate.
With the ability to adapt and learn from data, AI systems can optimize decision-making and solve problems that were once challenging or time-consuming for humans. In the modern world, AI is no longer just a futuristic concept but an integral part of everyday life. From virtual assistants like Siri and Alexa to self-driving cars and predictive analytics in healthcare, AI is reshaping industries by offering innovative solutions.
Businesses are utilizing AI for customer service, personalized marketing, fraud detection, and process automation, driving productivity and profitability. As AI continues to evolve, its potential to transform industries and improve lives becomes more apparent, making it an essential technology for the future.
AI is a core component in the development of autonomous vehicles, where it empowers self-driving cars to navigate roads safely. By processing real-time data from various sensors, cameras, and radars, AI systems detect objects, recognize traffic signs, and map out the vehicle's surroundings. Through machine learning algorithms, these vehicles can make critical decisions like braking, accelerating, or turning without human intervention.
By continuously analyzing driving patterns and learning from the data, AI enables autonomous vehicles to improve their performance and safety over time. The integration of AI into autonomous vehicles enhances their ability to predict traffic flow and optimize routes. This minimizes delays, reduces fuel consumption, and ensures a smoother, more efficient journey for passengers.
Companies like Tesla, Waymo, and Uber are already using AI to develop autonomous vehicle technologies. As AI continues to evolve, these self-driving cars will become safer, more affordable, and widely accessible, revolutionizing the way people travel and reducing human-related traffic accidents.
AI has revolutionized healthcare diagnostics by offering advanced solutions for detecting diseases early and accurately. By leveraging machine learning algorithms, AI systems analyze medical images like X-rays, MRIs, and CT scans, identifying abnormalities such as tumors or fractures with impressive precision. These algorithms can learn to recognize patterns in large datasets of medical images, making them a powerful tool for radiologists and doctors.
AI’s ability to quickly process and interpret medical data results in faster and more accurate diagnoses, enabling healthcare professionals to intervene at earlier stages of disease progression. Furthermore, AI also contributes to personalized healthcare by analyzing patient data, including genetic information, to recommend tailored treatment options.
AI models predict the likelihood of disease development based on historical data, allowing healthcare providers to address potential health risks proactively. As AI continues to enhance healthcare delivery, it is transforming the field into a more efficient, data-driven system that provides better outcomes for patients and reduces overall healthcare costs.
AI-driven chatbots and virtual assistants have become integral to customer service, transforming the way businesses engage with their customers. These systems use natural language processing (NLP) to understand and respond to customer queries in real time, making them highly effective in handling routine tasks. By providing instant responses, chatbots can resolve inquiries, track orders, and assist with account management, enhancing the customer experience.
They learn from interactions, improving their responses over time to offer more relevant solutions, making them indispensable for customer support teams. Moreover, virtual assistants like Siri, Alexa, and Google Assistant go beyond customer service applications by helping users with personal tasks, such as setting reminders, controlling smart home devices, or getting weather updates.
These systems are continuously improving through machine learning and data analysis, making them more intuitive and responsive to user needs. As AI-powered assistants become more sophisticated, businesses will increasingly rely on them to automate interactions, reduce operational costs, and provide a seamless experience for users.
Fraud detection systems powered by AI are critical in protecting businesses and consumers from financial fraud. These AI algorithms analyze vast amounts of transaction data in real time, identifying patterns and anomalies that may indicate fraudulent behavior. By monitoring user activity and transaction histories, AI systems can detect suspicious behavior, such as sudden large withdrawals or uncharacteristic purchasing patterns, and trigger alerts for further investigation.
The adaptability of machine learning models ensures that these systems evolve, staying ahead of emerging fraud tactics and ensuring ongoing protection. In addition to real-time detection, AI helps financial institutions proactively prevent fraud by analyzing historical data to identify high-risk areas and potential vulnerabilities. Predictive models assess the likelihood of fraud and suggest preventative measures, such as adjusting transaction limits or increasing verification requirements.
These systems not only improve security but also reduce false positives, minimizing disruptions for legitimate customers. With AI’s help, businesses can better safeguard sensitive information, ensuring customer trust and enhancing security in financial operations.
Smart home technology powered by AI is transforming the way we live by making homes more efficient, secure, and convenient. Through devices such as smart thermostats, lighting systems, and security cameras, AI learns user preferences and adapts to daily routines. For instance, AI-powered thermostats adjust the temperature based on occupancy, ensuring comfort while saving energy.
Similarly, security cameras use facial recognition and movement detection to identify familiar faces and alert homeowners to unusual activity, enhancing home security. These AI-driven devices continuously improve their functionality by learning from user interactions, making them more effective over time. The integration of AI into home automation also supports energy efficiency by optimizing power usage.
Smart devices communicate with each other, ensuring that lights, appliances, and heating systems operate only when necessary, significantly reducing wastage. With AI, homes can become more energy-efficient, sustainable, and secure. As these technologies evolve, the potential for smart homes to improve quality of life while contributing to a more sustainable environment will continue to grow, providing increased comfort and convenience to users.
AI has become an essential tool in the retail and e-commerce industries, driving personalized shopping experiences and operational efficiencies. AI algorithms analyze customer data, including browsing history and purchase patterns, to provide tailored recommendations. This helps businesses create personalized shopping experiences that enhance customer satisfaction and drive higher conversion rates.
AI-powered recommendation engines, like those used by Amazon and Netflix, suggest products based on individual preferences, increasing the chances of a purchase and improving overall customer retention. AI also plays a crucial role in optimizing supply chain management, where predictive analytics help retailers forecast demand, manage inventory, and reduce stockouts or overstocking.
AI models predict customer demand based on past sales, seasonal trends, and external factors, allowing businesses to adjust their inventory levels accordingly. In addition, AI is used for dynamic pricing, adjusting prices based on real-time market conditions and customer behavior. This enables retailers to maximize revenue and stay competitive in an increasingly digital marketplace.
AI-driven language translation tools are breaking down language barriers by offering real-time, accurate translations of text and speech. Tools like Google Translate use advanced natural language processing (NLP) algorithms to convert text and speech from one language to another. These systems are trained on vast amounts of multilingual data, allowing them to detect nuances, idiomatic expressions, and context, resulting in higher-quality translations.
They are increasingly being used by businesses and individuals to communicate across language barriers, making global interactions more seamless. These AI-based translation tools are not only beneficial for international business communication but also for travelers, students, and researchers. They enable real-time conversations in foreign languages, improving the accessibility of information and enhancing cross-cultural understanding.
AI also helps in breaking down regional dialects and translating uncommon languages, ensuring that more people worldwide can access global knowledge. As machine translation improves, it will further facilitate communication and collaboration across cultures and industries.
AI is revolutionizing the education sector by personalizing learning experiences and automating administrative tasks. Adaptive learning platforms powered by AI assess students’ progress and adjust course materials according to individual learning speeds and preferences. This ensures that each student receives tailored content that meets their specific needs, improving learning outcomes and engagement.
AI-driven tutoring systems also provide extra support by offering real-time assistance on complex subjects, enabling students to learn at their own pace and reinforcing their understanding of key concepts. Beyond student learning, AI is transforming the way schools and universities operate. AI tools automate tasks such as grading, scheduling, and student progress tracking, freeing up educators’ time to focus on teaching.
AI can also assist in monitoring students’ emotional well-being and academic performance, identifying at-risk students early, and providing timely interventions. As AI technologies continue to evolve, education will become more efficient, accessible, and tailored to the needs of both students and educators.
AI is revolutionizing industries like manufacturing and aviation through predictive maintenance. By analyzing sensor data, historical maintenance records, and environmental factors, AI systems can predict when machines or equipment are likely to fail, allowing businesses to address potential issues before they result in costly breakdowns.
These predictive models identify early warning signs, such as unusual vibrations, temperature changes, or wear and tear, and provide recommendations for maintenance or replacement. This proactive approach extends the lifespan of equipment and ensures minimal downtime, significantly reducing operational costs. Predictive maintenance powered by AI is also transforming the safety standards within industries.
By preventing unexpected failures, it reduces the risk of accidents or hazards caused by malfunctioning equipment. The integration of AI in maintenance systems allows organizations to optimize their maintenance schedules, reducing unnecessary interventions while ensuring critical systems are always functioning at peak performance. This shift toward predictive maintenance is enabling industries to achieve higher operational efficiency, cut costs, and improve safety standards across the board.
AI is increasingly shaping the entertainment industry by enhancing content discovery, production, and user experiences. Streaming services like Netflix, Spotify, and YouTube utilize AI-powered recommendation algorithms to suggest personalized content based on user preferences and viewing history. This not only helps users find content they enjoy but also increases engagement and keeps audiences hooked to platforms for longer periods.
AI’s ability to analyze massive amounts of data ensures that these recommendations are accurate and tailored to individual tastes. AI is also revolutionizing the creative aspects of entertainment, particularly in the areas of movie production and video games.
Machine learning algorithms are used to enhance CGI effects, creating more realistic visual effects and animation. AI-driven tools are also assisting content creators in the editing process by automating tedious tasks such as color correction and sound design. As AI continues to evolve, it will further enhance the quality of entertainment, allowing for more immersive and interactive experiences.
Data Science and Artificial Intelligence (AI) are closely related fields, but they differ in scope, goals, and methods. Data Science involves the use of scientific methods, algorithms, and systems to analyze and interpret large amounts of data. It focuses on gathering, processing, and extracting actionable insights from data to help businesses make informed decisions.
Data Science is often used to uncover patterns, trends, and correlations within datasets, which can lead to predictions, visualizations, and valuable business intelligence. On the other hand, Artificial Intelligence refers to the creation of machines or systems that can simulate human intelligence. AI systems are designed to perform tasks such as learning, reasoning, problem-solving, and decision-making without human intervention.
While Data Science provides the foundational insights and data for AI systems to function, AI goes a step further by automating tasks, making autonomous decisions, and learning from new data. The key distinction is that Data Science is focused on data analysis, while AI aims to mimic intelligent behavior and automate processes.
Data Science and Artificial Intelligence (AI) are two interconnected fields that have significantly impacted industries and business operations worldwide. Despite their differences, they share several similarities in their objectives, methodologies, and applications. Both fields rely heavily on data to derive insights and enhance decision-making processes.
While Data Science focuses on collecting, analyzing, and interpreting data to provide actionable insights, AI goes a step further by developing systems capable of making decisions and predictions autonomously based on the data. Both disciplines rely on machine learning and statistical techniques to model data and uncover hidden patterns. They share a common goal of optimizing business processes, improving operational efficiency, and delivering personalized experiences.
Additionally, both Data Science and AI are increasingly used together in various domains such as healthcare, finance, e-commerce, and manufacturing, where data-driven decision-making and automation can lead to innovative solutions and competitive advantages. Below is a detailed comparison of the similarities between Data Science and Artificial Intelligence.
Data Science has become one of the most sought-after fields in the tech industry due to its ability to drive business decisions and strategies through data insights. As companies across various industries recognize the value of data-driven decision-making, the demand for skilled Data Science professionals has surged. These roles encompass a wide range of responsibilities, from analyzing complex datasets to building predictive models and data visualizations that inform business strategies.
Data Scientists often work closely with other teams, leveraging their expertise in statistical analysis, programming, and machine learning to uncover patterns and trends that improve performance and efficiency. The career opportunities in Data Science are diverse, with job roles ranging from entry-level positions to senior data leadership roles.
Along with ample job opportunities, professionals in Data Science are rewarded with competitive salaries, which increase as individuals gain more experience and expertise. Below is an overview of some key Data Science roles and their corresponding salary ranges.
Artificial Intelligence (AI) has become one of the most sought-after fields due to its transformative impact on various industries, ranging from healthcare and finance to automotive and entertainment. As companies increasingly adopt AI technologies, the demand for skilled professionals in AI-related roles continues to rise. These roles span a wide range of expertise, including machine learning, deep learning, natural language processing, robotics, and AI research.
The salary ranges for AI-related jobs are significantly higher compared to many other tech roles, reflecting the specialized skills required in the field. Professionals in AI not only benefit from competitive salaries but also from opportunities for career growth and innovation.
The roles in AI require a deep understanding of algorithms, programming, data science, and problem-solving, which makes them highly valuable in today’s tech-driven economy. Here is a detailed look at some of the top AI job roles and their corresponding salary ranges.
Artificial Intelligence (AI) is a rapidly growing field of computer science dedicated to creating intelligent machines capable of mimicking human cognition. These machines can learn, reason, solve problems, and make decisions with minimal human intervention. AI relies on advanced algorithms and computational power to process vast amounts of data and identify complex patterns.
From chatbots to self-driving cars, AI has transformed industries by enhancing efficiency and driving innovation. AI and Data Science are deeply intertwined, with Data Science serving as the backbone for AI's success. Data Science involves collecting, cleaning, and analyzing data, which AI utilizes to train models, automate processes, and improve decision-making.
Together, they empower organizations to predict trends, optimize operations, and create intelligent solutions. The synergy between AI and Data Science drives groundbreaking advancements in technology and redefines the way businesses and societies operate. Below are the key connections between AI and Data Science:
Data science is rapidly transforming industries by enabling businesses to extract actionable insights from vast datasets. It involves programming, statistics, and data analysis to help organizations make informed decisions.
A solid academic foundation in fields like computer science, mathematics, and engineering is critical for entering the data science field. With a combination of technical expertise and practical experience, professionals can pursue careers in tech, healthcare, finance, and more.
Degrees such as a bachelor’s or master’s, coupled with certifications, provide individuals with specialized skills in areas such as machine learning, big data analytics, and business decision-making. The demand for data scientists continues to grow, making it an exciting and rewarding career path for those who have the necessary skills and knowledge.
Artificial Intelligence (AI) is revolutionizing industries by automating processes and creating intelligent systems. To succeed in AI, professionals need a deep understanding of algorithms, data structures, machine learning techniques, and mathematical concepts. Degrees that provide expertise in these areas, such as computer science, electrical engineering, and robotics, are crucial for building AI systems.
With the increasing demand for AI solutions in industries like healthcare, finance, and technology, there are numerous career opportunities for individuals who pursue advanced studies in AI and related fields.
A solid foundation in the core principles of AI, combined with specialized skills in subfields like robotics or computational neuroscience, can lead to rewarding roles in research, development, and innovation.
Data science and artificial intelligence (AI) are two rapidly growing fields, each offering exciting career opportunities. Data science primarily focuses on extracting valuable insights from large datasets using statistical analysis, machine learning, and data visualization techniques. Professionals in data science work with data processing, data mining, and predictive modeling to help organizations make data-driven decisions. If you enjoy working with data, uncovering patterns, and creating actionable insights for business strategy, a career in data science may be the right path for you.
According to recent industry reports, the global data science market is expected to grow from $37.9 billion in 2023 to $104.8 billion by 2030, highlighting the increasing demand for skilled data professionals. On the other hand, AI focuses on creating intelligent systems that can mimic human cognitive functions, such as learning, reasoning, and decision-making. AI encompasses areas like machine learning, deep learning, and natural language processing to develop applications such as autonomous vehicles, virtual assistants, and personalized recommendations.
If you're interested in building systems that can perform tasks autonomously or develop algorithms that enable machines to "think," then AI may be more aligned with your interests. The AI market is predicted to reach $500 billion by 2024, driven by innovations in automation, robotics, and AI-driven analytics. Both fields have overlapping skills but differ in their end goals—data science aims at using data to inform decisions, while AI is about creating intelligent systems that can perform tasks independently. The choice between the two depends on your interests in data analysis versus developing autonomous, intelligent systems.
Both data science and AI offer dynamic career opportunities, but the choice between the two depends on your interests and career goals. Data science is more about analyzing and interpreting data to guide business decisions, leveraging statistical models, machine learning, and data visualization techniques. If you're passionate about deriving insights and improving decision-making processes, data science might be the right path.
On the other hand, AI involves developing intelligent systems that can mimic human cognition and perform tasks autonomously. If you want to explore the cutting-edge of automation and machine learning, AI would be a great choice. Both fields are essential in today's technological landscape and offer tremendous growth potential in the coming years.
Copy and paste below code to page Head section
Data Science focuses on analyzing data to derive insights and inform decision-making using statistical techniques and machine learning. Artificial Intelligence (AI) develops systems that can perform tasks autonomously by mimicking human cognitive functions, such as learning and problem-solving. While Data Science extracts value from data, AI creates intelligent systems capable of evolving.
Both Data Science and AI offer strong job prospects. However, AI is expected to see faster growth due to the increasing reliance on automation and intelligent systems across industries. Data Science also remains highly in demand, particularly in sectors such as healthcare, finance, and marketing, as businesses rely on data-driven decision-making.
A career in Data Science requires proficiency in programming languages like Python and R, as well as knowledge of machine learning algorithms, data analysis, and statistical modeling. Familiarity with tools like SQL, Tableau, and Hadoop, along with strong mathematical and analytical skills, is crucial for successfully analyzing and interpreting complex datasets.
To pursue AI, a Bachelor's degree in Computer Science, Engineering, or Mathematics is typically required. A Master's or Ph.D. in Artificial Intelligence or related fields provides in-depth knowledge of machine learning, neural networks, and deep learning. Certifications in AI tools and programming languages like Python are also highly beneficial for career advancement.
Yes, it’s possible to learn both Data Science and AI simultaneously, as they share many foundational concepts such as machine learning. However, Data Science focuses more on extracting insights from data, while AI emphasizes building intelligent systems. A good approach is first to master the basics of Data Science and then move on to AI-specific topics.
AI plays a crucial role in Data Science by enhancing the analytical process through advanced machine learning algorithms and automation. AI enables the creation of models that can make predictions and decisions without human intervention, thus improving efficiency and accuracy in data processing. AI is often used to uncover deeper insights from large datasets.