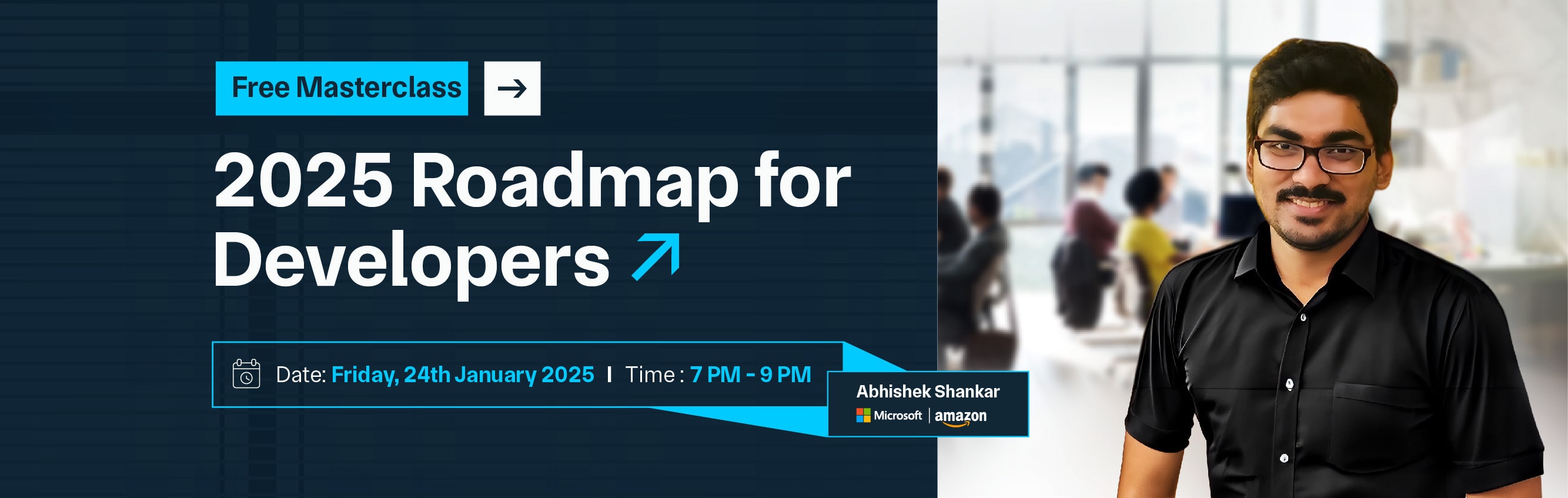

The integration of data science into agriculture is revolutionizing traditional farming methods, driving precision, efficiency, and sustainability. With the growing demand for food due to population increase and climate change challenges, the agricultural sector is adopting advanced technologies to optimize resources and enhance productivity. Data science enables farmers to make data-driven decisions by analyzing patterns and trends, helping them manage crops, water, and soil more effectively. Through predictive analytics and machine learning, data science facilitates better crop management by forecasting weather conditions, pest infestations, and yield outcomes.
These insights empower farmers to take proactive measures, reducing risks and ensuring optimal growth conditions. Satellite imagery, drones, and IoT devices further complement data science applications, providing real-time data on field conditions, irrigation needs, and disease detection. Sustainability is a core focus of data science in agriculture, addressing the pressing need for eco-friendly practices. By optimizing fertilizer use, reducing water wastage, and minimizing environmental impact, data-driven techniques contribute to greener farming.
Additionally, the fusion of blockchain and data science ensures transparency and traceability in the food supply chain. As the agricultural landscape evolves, data science stands as a pivotal tool to meet global food security goals while promoting innovative and sustainable farming practices. This convergence is shaping a smarter, greener future for agriculture worldwide.
Data science in agriculture is the application of advanced analytical tools and techniques to improve farming practices and outcomes. By harnessing data from multiple sources, including sensors, satellites, and historical records, data science helps farmers make informed decisions that enhance crop yield, reduce costs, and ensure resource efficiency. It involves using technologies like machine learning, artificial intelligence, and big data analytics to process and interpret large datasets, enabling precision agriculture.
This empowers farmers to address specific challenges, such as weather variability, pest outbreaks, and soil health, with targeted solutions. Beyond immediate agricultural productivity, data science plays a critical role in shaping the sustainability and resilience of farming systems. Analyzing environmental factors and resource usage aids in reducing wastage, optimizing irrigation, and promoting eco-friendly farming practices.
Furthermore, data science supports supply chain management by improving the tracking, forecasting, and distribution of agricultural products. This integration ensures not only higher profitability for farmers but also the availability of safe and nutritious food for consumers. In a rapidly changing world, data science in agriculture stands as a transformative approach to meeting global food demands while fostering innovation and environmental stewardship.
Data science is redefining sustainable farming by introducing precision and efficiency into agricultural practices. By analyzing large datasets from IoT devices, weather sensors, and satellite imagery, farmers can make more informed decisions that minimize waste and optimize resource use. Techniques such as predictive analytics enable the anticipation of environmental changes, helping farmers adapt their practices to maintain soil fertility and conserve water.
This data-driven approach not only increases crop yield but also reduces the ecological footprint of farming by promoting the judicious use of fertilizers and pesticides. Moreover, data science facilitates the integration of sustainability into the entire agricultural value chain. From monitoring greenhouse gas emissions to ensuring biodiversity-friendly farming methods, data analytics helps achieve long-term ecological balance.
Blockchain technology, combined with data science, also enhances traceability in the food supply chain, ensuring ethical and sustainable sourcing. As climate change pressures intensify, the synergy between data science and sustainable farming offers a practical path to address global food security while preserving natural ecosystems. This intersection exemplifies how technology can harmonize productivity and sustainability, paving the way for a resilient agricultural future.
Data science is playing a transformative role in modern agriculture by leveraging technology and analytics to address challenges in farming. It enables farmers to make data-driven decisions, optimize resource use, and improve overall productivity. With the integration of IoT devices, satellite imagery, and predictive models, data science helps monitor and enhance every stage of the agricultural process.
From soil analysis to crop forecasting, its applications ensure sustainable practices and higher yields while minimizing environmental impact. Furthermore, data science fosters innovation by providing actionable insights into pest management, weather patterns, and supply chain efficiency. This approach not only supports individual farmers but also strengthens the global agricultural infrastructure.
By combining advanced technologies like machine learning and artificial intelligence, data science is empowering the agricultural sector to meet growing food demands sustainably. Let’s explore the key roles of data science in transforming agriculture, offering farmers the tools to build a more efficient and environmentally conscious future.
Data science is revolutionizing agriculture by making farming practices more efficient, precise, and sustainable. The integration of advanced analytics, machine learning, and data-driven decision-making enables farmers to optimize resource usage, increase crop yields, and enhance overall farm productivity. Data science helps tackle challenges like climate change, pest outbreaks, and supply chain inefficiencies, making it an essential tool for modern agriculture.
With the help of big data, cloud computing, and AI, farmers are equipped to meet the growing demand for food while minimizing environmental impact. From precision farming to livestock management, data science offers a range of applications that benefit both large-scale operations and smallholder farms.
The insights derived from data-driven methods allow farmers to make smarter decisions that save time, reduce costs, and improve long-term sustainability. Below are ten key applications of data science in agriculture that highlight how technology is transforming the industry.
Data science is revolutionizing how farmers detect and prevent crop diseases. Through the integration of sensors, satellite imagery, and machine learning models, farmers can identify potential disease outbreaks before they spread across crops. By analyzing environmental conditions such as humidity, temperature, and soil health, data-driven systems predict the likelihood of diseases like blight or mildew. This allows farmers to take preventative measures, such as applying pesticides or altering crop rotation schedules before the problem escalates.
As a result, farmers can reduce the use of chemicals, minimize crop loss, and enhance overall crop health. With data science, farmers are empowered to make proactive decisions that lead to healthier, more resilient crops, thereby improving yield and sustainability. The ability to predict and prevent disease before it spreads is vital for ensuring the longevity of both crops and farming operations.
Data science is integral to the development of autonomous farming equipment, which is reshaping agriculture by reducing labor costs and increasing precision. Self-driving tractors, harvesters, and drones are becoming increasingly common on farms, using advanced algorithms to operate without human intervention. These machines gather vast amounts of data, from soil conditions to crop health, and adjust their operations in real time. For example, autonomous tractors use GPS and data from soil sensors to ensure that seeds are planted at the right depth and spacing, maximizing crop yield.
Similarly, autonomous harvesters can identify the optimal time for harvesting based on crop maturity, improving efficiency. This technology not only saves labor but also reduces resource waste, as equipment can be optimized for each task. Through the integration of machine learning and AI, autonomous farming equipment improves productivity while minimizing the environmental impact, making agriculture more sustainable and cost-effective.
Pest management has traditionally been a reactive process, but with data science, it is becoming predictive. By using data from multiple sources, including field sensors, weather stations, and satellite images, predictive analytics can forecast pest outbreaks. Machine learning algorithms analyze patterns and environmental factors to predict the likelihood of pest invasions in specific areas. For example, if weather conditions are favorable for certain pests, predictive models can alert farmers to take preventive actions, such as using targeted pesticides or applying organic treatments.
This proactive approach not only reduces the amount of pesticides needed but also minimizes the environmental impact and enhances biodiversity. Data science enables farmers to manage pests more effectively, ensuring healthier crops and higher yields. In addition, pest management strategies can be tailored to individual farms, improving their overall efficiency and sustainability while minimizing the risks of overuse of chemicals in agriculture.
Precision fertilization, supported by data science, has revolutionized how farmers manage soil health and nutrient application. Traditional fertilization techniques often lead to overuse or misuse of fertilizers, which can result in environmental pollution and higher costs. Data science addresses this challenge by providing detailed insights into soil conditions, moisture levels, and nutrient deficiencies, allowing farmers to apply fertilizers precisely where they are needed. Using tools like soil sensors, drone imagery, and weather data, farmers can optimize the amount and timing of fertilizer application, improving crop yield and minimizing waste.
Machine learning models further refine this process by predicting which crops will need more nutrients at different growth stages. As a result, farmers can reduce their environmental impact, lower input costs, and boost the sustainability of their farming operations. The data-driven approach ensures that every input is maximized for productivity, contributing to more efficient and sustainable farming practices.
Climate change poses significant challenges to agriculture, including unpredictable weather patterns, extreme temperatures, and droughts. Data science plays a vital role in helping farmers adapt to these changing conditions by providing predictive models and climate forecasting tools. By analyzing historical weather data, soil moisture levels, and global climate models, data scientists can help farmers prepare for and mitigate the impacts of extreme weather events. For example, predictive models can forecast the likelihood of drought in a region, allowing farmers to adjust irrigation schedules or switch to more drought-resistant crops.
Similarly, data models can predict seasonal temperature shifts, helping farmers plan planting and harvesting times to avoid crop loss. This adaptability helps farmers reduce the risks associated with climate change, making agriculture more resilient and sustainable. Through data science, farmers gain insights that allow them to manage and adapt to environmental uncertainties proactively, improving long-term farm productivity.
Remote sensing technology, powered by data science, has transformed how farmers monitor crop health and conditions. Using satellite imagery, drones, and sensors, farmers can gather real-time data on factors such as crop stress, soil moisture, and plant growth. Machine learning algorithms process this data to identify patterns and detect early signs of issues like nutrient deficiencies, disease, or water stress. This continuous monitoring helps farmers take immediate corrective actions, such as adjusting irrigation or applying fertilizers, leading to healthier crops and better yields.
Real-time crop monitoring also reduces the need for manual inspections, saving time and labor costs. The ability to monitor crops remotely enables farmers to cover large areas more efficiently, making it possible to manage vast farms with greater precision. Additionally, remote sensing can be used to track environmental conditions, helping farmers make data-driven decisions for sustainable and efficient farming practices.
Data science is powering the rise of smart greenhouses and controlled environment agriculture (CEA), where farmers can grow crops in optimized, controlled conditions. These systems rely on sensors and IoT devices to continuously monitor variables like temperature, humidity, light levels, and CO2 concentrations. Machine learning algorithms process this data to adjust environmental factors in real time, creating the ideal conditions for plant growth. Smart greenhouses also use automated systems to control irrigation, fertilization, and pest management, minimizing resource waste while maximizing crop yields.
The integration of AI and robotics further enhances these systems, allowing for fully automated farming. CEA can increase food production in urban areas where traditional farming is not feasible, and it is particularly useful in regions with harsh climates. By reducing the need for external inputs such as pesticides and fertilizers, smart greenhouses contribute to sustainable farming practices while also ensuring consistent and high-quality food production.
Data science helps optimize the agricultural supply chain by analyzing vast amounts of data related to production, logistics, and market demand. Predictive analytics can forecast demand for specific crops, allowing farmers to adjust production schedules accordingly. Big data tools track inventory levels, weather patterns, and market trends to optimize the timing and route for transportation, reducing waste and improving efficiency. By improving logistics, data science helps farmers get their products to market faster, ensuring better pricing and reducing food spoilage.
Additionally, data science can enhance transparency and traceability in the supply chain, enabling consumers to know where their food comes from and ensuring ethical sourcing practices. With real-time monitoring and analytics, farmers and distributors can collaborate more effectively, leading to a more resilient and profitable supply chain. The insights derived from big data help streamline the entire process from farm to table, benefiting both farmers and consumers alike.
Automated crop harvesting, driven by data science, is changing the way farmers approach the labor-intensive task of harvesting. Robotics, powered by machine learning and computer vision, can detect the optimal time for harvest and perform the task with precision. Data from sensors and cameras allows automated harvesters to distinguish ripe crops from unripe ones, ensuring that only the best-quality produce is collected. This reduces labor costs, minimizes damage to crops, and improves harvesting efficiency.
By leveraging predictive analytics, these systems can anticipate crop readiness based on environmental conditions and growth patterns, allowing farmers to schedule harvests more effectively. Automated harvesting systems also reduce food waste by harvesting crops at the peak of ripeness, ensuring that produce reaches the market in the best condition. With advancements in robotics and data science, automated harvesting is expected to become more widespread, contributing to greater efficiency and sustainability in agriculture.
Blockchain technology is increasingly being used in agriculture to improve transparency, traceability, and trust in the food supply chain. By recording every step of the agricultural process on an immutable ledger, blockchain ensures that consumers can trace the origin of their food and verify its sustainability credentials. Data science tools analyze blockchain data to track production, processing, and transportation, providing detailed insights into food provenance. This transparency benefits both consumers and farmers, as it enables consumers to make informed purchasing decisions and incentivizes farmers to adopt sustainable practices.
Additionally, blockchain helps reduce fraud, foodborne illnesses, and waste by providing an unalterable record of transactions. The integration of blockchain and data science in agriculture ensures that food systems are more transparent, accountable, and efficient while also promoting sustainability and ethical sourcing practices across the supply chain.
Data science is making a significant impact on agriculture worldwide, transforming traditional farming methods and increasing efficiency. By utilizing machine learning, AI, and big data analytics, farmers and agribusinesses are optimizing crop yields, reducing resource usage, and tackling global challenges like climate change and food security.
From smart irrigation systems to precision agriculture tools, real-world applications of data science are driving innovation in the agricultural sector. Below are eight key examples of how data science is being applied to modern farming practices, each showing tangible results and advancements that highlight the power of technology in agriculture.
John Deere has been at the forefront of precision agriculture, integrating data science into its farming equipment to optimize operations. The company’s tractors, harvesters, and planters are equipped with GPS and sensor-based technology that collects real-time data on soil conditions, crop health, and environmental factors. This data helps farmers make data-driven decisions on the best times for planting, fertilizing, and harvesting crops. By utilizing machine learning algorithms, these tools allow for precise application of water, pesticides, and fertilizers, minimizing waste and improving efficiency.
The implementation of these precision agriculture technologies by John Deere has shown remarkable results, with farmers reporting up to a 15% increase in crop yields and a reduction in input costs by 10-15%. The application of this technology allows farmers to become more sustainable, not only by reducing their environmental impact but also by enhancing farm productivity. Additionally, John Deere’s integration of artificial intelligence further enables farmers to anticipate challenges like soil compaction or moisture levels, optimizing their operations even more efficiently.
The FieldView platform, developed by The Climate Corporation, is a leading example of how data science can be applied to precision farming. FieldView integrates satellite imagery, weather forecasts, and data analytics to provide farmers with actionable insights on crop health, soil conditions, and weather impacts. By tracking real-time data from the field, the platform offers recommendations for optimizing irrigation, pest control, and fertilization, ensuring crops receive exactly what they need at the right time. Farmers using the platform have reported an average increase of 5-10% in crop yields, demonstrating the power of data-driven decision-making.
The platform also tracks historical data, enabling farmers to compare past and present field conditions and adjust practices accordingly. Additionally, FieldView helps farmers mitigate risk by forecasting potential adverse weather conditions and adjusting operations to avoid losses. With over 100 million acres of farmland in the U.S. alone using this technology, Climate Corporation’s data-driven approach is revolutionizing the agricultural industry by enabling farmers to maximize efficiency while minimizing costs.
IBM’s Watson Decision Platform for Agriculture leverages the power of AI, machine learning, and data analytics to support farmers in optimizing crop production and reducing risks associated with climate change. The platform integrates data from various sources, including weather stations, sensors, satellite images, and local farming data. By combining this data with predictive analytics and real-time decision-making tools, farmers can make informed decisions about irrigation schedules, crop rotations, and pest management. This helps them maximize productivity while reducing resource consumption and waste.
In collaboration with other agribusinesses, IBM has seen farmers achieve significant improvements, such as a 12% increase in crop yields and a 20-30% reduction in costs. The platform’s ability to monitor conditions continuously and adjust inputs based on data-driven insights ensures that each stage of the farming process is optimized. Additionally, Watson’s machine learning models continually improve based on the feedback and data collected, further refining predictions and enhancing the decision-making process for farmers.
Farmers Edge is a Canadian agritech company that specializes in providing farmers with data-driven solutions through a combination of cloud-based software, smart sensors, and analytics tools. Their platform collects data from a wide array of sources, including weather stations, satellite imagery, and IoT devices embedded in the field. By analyzing this data, Farmers Edge helps farmers make real-time decisions on planting, fertilization, irrigation, and pest control. This precision farming approach not only improves crop yields but also reduces waste and input costs.
In fact, farmers using Farmers Edge’s platform have reported up to a 7% increase in yields and a 15-20% reduction in operating costs. By using predictive analytics, the platform also helps farmers prepare for adverse weather conditions, such as droughts or storms, allowing them to adjust their operations to mitigate potential risks. The data-driven recommendations provided by Farmers Edge enable farmers to optimize their resources more effectively, leading to a more sustainable and profitable agricultural operation.
Syngenta, a global leader in agribusiness, has implemented a cutting-edge pest and disease management system that uses data science to predict and prevent crop damage. This system analyzes a combination of satellite images, weather data, and field sensor readings to monitor the conditions that are conducive to pest and disease outbreaks. By applying machine learning algorithms to this data, Syngenta can predict when and where pest problems are likely to occur. With this predictive information, farmers can apply targeted treatments only when necessary, minimizing pesticide use while maintaining effective pest control.
This data-driven approach has been shown to reduce pesticide use by up to 40% while still achieving effective pest management. In some regions, Syngenta’s crop protection system has led to a 10-20% increase in crop yield, as it enables farmers to address pest issues before they cause significant damage proactively. Furthermore, the system has a lower environmental impact compared to traditional pest management methods, making it a more sustainable option for modern farming.
PrecisionHawk, a drone technology company, has integrated data science into its crop monitoring services to revolutionize how farmers track crop health and productivity. Using drones equipped with high-resolution cameras and sensors, PrecisionHawk collects detailed data on soil conditions, crop stress, and irrigation efficiency. This data is processed using machine learning algorithms that identify areas in need of attention, such as underperforming crops or areas experiencing water stress. By providing farmers with real-time insights, the technology allows for more efficient use of water, fertilizers, and pesticides.
In a large-scale trial conducted in the U.S., drone technology helped farmers reduce water usage by 25% while increasing crop yields by 15%. This precision approach enables farmers to maximize their yields with fewer resources, improving sustainability in the process. Drones also help improve the speed and accuracy of crop monitoring, enabling farmers to cover larger areas in less time compared to traditional field inspections. PrecisionHawk’s innovative use of data science and drones is driving more efficient and sustainable agricultural practices.
AeroFarms, a pioneer in vertical farming, uses data science to optimize crop production in a controlled indoor environment. Their aeroponic farming system is powered by a network of sensors that monitor conditions such as temperature, humidity, CO2 levels, and light intensity. These sensors feed data into AI algorithms that automatically adjust these variables to create the ideal environment for plant growth. This data-driven approach allows AeroFarms to achieve up to 30 times the crop yield per square foot compared to traditional farming methods.
The company has also reduced water usage by 95% compared to conventional farming, highlighting the sustainability benefits of this technology. AeroFarms uses machine learning to continuously optimize its operations, refining the farming process and further improving crop production. The company’s innovative use of data science has not only improved efficiency and sustainability but also addressed food security concerns by enabling food production in urban environments where traditional farming may not be feasible.
Rabobank, a global leader in agricultural finance, uses big data to optimize food supply chains around the world. The company analyzes vast amounts of data from farmers, distributors, and retailers to forecast demand, predict price trends, and optimize transportation logistics. This helps streamline the entire food supply chain, reducing food waste and ensuring that products are delivered efficiently to the right markets at the right time. Rabobank’s big data-driven approach has been credited with improving the profit margins of agricultural businesses by 10-20% and reducing food waste by 15%.
In addition to improving logistics, the company’s data analytics tools also help farmers predict market trends, enabling them to adjust production strategies to meet demand. Rabobank’s role in integrating data science into the agricultural supply chain enhances efficiency, transparency, and profitability for all stakeholders, from farmers to consumers. By leveraging data analytics, Rabobank is contributing to a more sustainable and resilient global food system.
Sustainable agriculture focuses on meeting current food production needs without compromising future generations' ability to do the same. To achieve this, technology and innovative tools are crucial in optimizing resources, minimizing waste, and improving productivity. Advances in data science, automation, and environmental monitoring have made it easier for farmers to adopt eco-friendly practices while increasing efficiency.
From precision farming tools to AI-driven analytics, these technologies play a significant role in shaping the future of agriculture. By integrating cutting-edge technologies, sustainable agriculture becomes more accessible, helping farmers to enhance yields, conserve resources, and protect ecosystems.
Tools such as drones, IoT devices, and machine learning models are central to the revolution in farming practices. These technologies enable data-driven decision-making, improved crop management, and reduced environmental impact. Below are some key tools and technologies transforming sustainable agriculture.
Data science has become an indispensable tool in modern agriculture, revolutionizing how farming practices are carried out. By leveraging big data, machine learning, and predictive analytics, it helps farmers make informed decisions that enhance productivity, reduce costs, and promote sustainability. From real-time crop monitoring to precise irrigation techniques, data science ensures that resources are used efficiently, benefiting both the environment and the economy.
Additionally, data science empowers farmers to address challenges like climate variability, pest outbreaks, and soil degradation with advanced solutions. It also strengthens the agricultural supply chain by optimizing logistics, reducing waste, and increasing transparency.
As the demand for food grows and environmental concerns mount, data science is proving crucial in shaping a resilient and sustainable agricultural future. Below are some key benefits of data science in agriculture, highlighting its transformative impact on the industry.
Agricultural data science has the potential to revolutionize farming, but it is not without its challenges. While the integration of advanced technologies and data analytics offers immense opportunities, various obstacles limit its widespread adoption. These challenges stem from the complexity of agricultural systems, the diversity of data sources, and the technical expertise required to analyze and interpret the data.
Additionally, resource constraints in rural areas often hinder the implementation of data-driven solutions. Issues such as data quality, accessibility, and privacy concerns further complicate the effective use of data science in agriculture. Farmers, especially smallholders, may struggle with the financial and technical demands of adopting these technologies.
Overcoming these barriers requires collaborative efforts among policymakers, technologists, and agricultural experts. Below are the key challenges faced in agricultural data science and the implications they have for the future of farming innovation.
As we move towards 2025, data science is poised to revolutionize agriculture through advancements in artificial intelligence, machine learning, and the Internet of Things (IoT). One of the key trends is the continued growth of precision agriculture, where data-driven insights are used to make farming practices more efficient and sustainable. For instance, AI-powered algorithms are expected to improve crop yield predictions by 20-30% by analyzing a combination of weather, soil health, and crop data. Additionally, the rise of drone technology and satellite imagery will enable real-time monitoring of fields, allowing for early detection of pests, diseases, and nutrient deficiencies.
The integration of autonomous machinery, including tractors and harvesters, with AI systems will also become more prevalent, reducing labor costs and increasing operational efficiency. These trends are expected to make agriculture more data-centric, with farmers increasingly relying on sophisticated models to optimize resource usage, reduce waste, and maximize production. By 2025, the use of big data in agriculture is predicted to expand significantly, with the global agricultural data market reaching over $15 billion. This growth will be driven by the increasing availability of data from sensors, weather stations, and IoT devices, as well as the development of more advanced data analytics tools.
The future of agricultural data science will also see greater integration of sustainability metrics, with data scientists playing a pivotal role in ensuring that farming practices contribute to environmental conservation and climate change mitigation. With climate change impacting agricultural productivity, there will be an increased focus on using data to optimize water management, reduce carbon footprints, and increase resilience to extreme weather events. These developments will not only improve food security but will also help the agricultural sector meet the global sustainability goals set for 2030.
Data plays a pivotal role in modern agriculture, enabling farmers to make informed decisions and optimize their operations. With advancements in technology, various types of agricultural data are collected, analyzed, and applied to enhance productivity, sustainability, and efficiency.
These datasets encompass environmental, biological, and operational aspects of farming, offering insights into soil health, weather conditions, crop performance, and market trends. The diversity of agricultural data ensures that farmers can address challenges specific to their regions and practices.
From precision farming to supply chain management, the application of data science relies heavily on accurate and comprehensive data. Understanding the types of data used in agriculture is essential to leveraging their potential effectively. Below are the key types of data that are shaping the future of farming.
A data scientist plays a transformative role in modern agriculture, bridging the gap between technology and traditional farming practices. Their expertise lies in collecting, analyzing, and interpreting vast amounts of data to derive actionable insights. In agriculture, data scientists enable farmers to optimize resource use, predict outcomes, and make informed decisions that enhance productivity and sustainability.
They work across diverse areas, including crop management, weather analysis, and supply chain optimization. The role extends beyond data crunching; data scientists also develop predictive models and machine learning algorithms tailored to agricultural needs. They collaborate with agronomists, environmental scientists, and tech developers to create integrated solutions for challenges like climate change, pest infestations, and resource scarcity.
By introducing precision and efficiency, data scientists empower the agricultural sector to adapt to global demands and environmental challenges. Below are some unique contributions of data scientists in agriculture.
Becoming a data scientist in agriculture involves a unique blend of technical expertise and domain-specific knowledge to address the challenges faced by the agricultural industry. The role requires proficiency in data analysis, machine learning, and statistical modeling while also having an understanding of agricultural practices and processes.
With the increasing integration of technology in farming, data scientists are now playing a critical role in transforming the way agricultural decisions are made, from crop yield predictions to optimizing irrigation systems.
To succeed in this field, a data scientist must possess strong programming skills, familiarity with big data technologies, and an understanding of agricultural principles like crop cycles, pest management, and resource optimization. Additionally, they must be skilled in communicating complex data insights to non-technical stakeholders, such as farmers or agronomists.
A data scientist in agriculture must be proficient in programming languages such as Python, R, and SQL. Python is particularly useful due to its extensive libraries (like Pandas, NumPy, and TensorFlow) that aid in data analysis, machine learning, and statistical modeling. R is ideal for statistical analysis, while SQL is essential for querying large agricultural datasets stored in databases. These programming languages are the foundation of data manipulation, cleaning, and analysis, allowing data scientists to work efficiently with agricultural data.
Being able to develop algorithms, automate data processes, and create predictive models is crucial in making data-driven decisions for farm management. In agriculture, these skills are often applied in analyzing crop growth patterns, weather trends, and soil health, ultimately aiding in more precise resource allocation. Proficiency in these languages is essential to ensure that data science solutions are implemented accurately and effectively across different agricultural platforms.
Machine learning is a key tool in modern agricultural data science, enabling data scientists to develop models that predict crop yields, detect plant diseases, and optimize irrigation. A data scientist in agriculture must have in-depth knowledge of various machine learning algorithms, including supervised learning, unsupervised learning, and reinforcement learning. These algorithms help identify patterns in large datasets, such as soil conditions, weather patterns, and historical crop performance. For instance, supervised learning models can predict the optimal time for planting or harvesting, while unsupervised learning can identify emerging trends in pest control.
Data scientists should be adept at implementing algorithms using tools like TensorFlow, Keras, and Scikit-learn. In addition, understanding how to fine-tune model parameters, evaluate model performance, and apply cross-validation techniques is essential for producing reliable results. Machine learning algorithms are indispensable in solving complex problems like crop disease detection and yield forecasting, making them a fundamental skill for aspiring agricultural data scientists.
Statistical analysis is an essential skill for data scientists in agriculture, as it helps in drawing meaningful insights from complex datasets. Understanding various statistical techniques such as regression analysis, hypothesis testing, and time-series analysis is crucial when working with agricultural data. This skill allows data scientists to analyze patterns and relationships in data, such as the impact of weather conditions on crop yield or the effectiveness of irrigation systems. Additionally, data interpretation skills are necessary to translate complex data outputs into actionable insights that farmers and agricultural professionals can use.
Data scientists must be able to identify trends, correlations, and anomalies in large datasets, and they should be able to communicate these insights clearly to stakeholders through data visualization tools. Proficiency in statistical software like SAS, SPSS, or R is often required. By applying statistical analysis and interpreting the results, data scientists can provide farmers with vital information to enhance decision-making and improve agricultural outcomes.
In addition to technical skills, a data scientist working in agriculture must have a solid understanding of agricultural processes, such as crop production, irrigation, pest management, and soil health. Familiarity with farming practices and how data can be applied to improve them is crucial. For instance, knowledge of crop growth cycles, the impact of weather patterns on yields, and the specifics of irrigation methods can help data scientists design more effective predictive models. Moreover, understanding the challenges faced by farmers, such as water scarcity, pest outbreaks, and soil degradation, is necessary for developing data-driven solutions that address these issues.
Domain expertise also aids in communicating effectively with agricultural professionals and translating their needs into technical requirements. Collaboration with agronomists and farmers is key to ensuring that the solutions developed are practical and relevant to the agricultural sector. Data scientists who combine their technical expertise with agricultural knowledge can create more accurate models and provide better insights for sustainable farming practices.
Data visualization is a crucial skill for data scientists in agriculture because it allows complex data to be presented in a way that is easily understandable by farmers and stakeholders. Proficiency in tools like Tableau, Power BI, and Matplotlib is essential for creating clear and actionable visual representations of agricultural data. These tools enable data scientists to present findings, such as crop yield predictions or soil health trends, in a manner that farmers can easily interpret and act upon. Effective visualizations can also help in identifying patterns, anomalies, or correlations in large datasets that may not be immediately apparent through raw data alone.
For example, a data scientist might use geographic information system (GIS) tools to map soil quality or moisture levels across different areas of a farm. The ability to communicate insights through compelling visual stories is essential in ensuring that data-driven decisions are made and that stakeholders can easily grasp the information presented to them.
Agricultural data scientists often work with large, complex datasets, so familiarity with big data technologies is essential. Tools such as Apache Hadoop, Apache Spark, and cloud platforms like AWS or Google Cloud are widely used to store, process, and analyze agricultural data. These tools allow data scientists to handle massive amounts of information, such as satellite imagery, sensor data from fields, or real-time weather data, at scale. Big data technologies enable the processing of data in real-time, which is crucial for tasks such as monitoring crop conditions, managing irrigation schedules, or predicting pest outbreaks.
Additionally, experience in managing and working with databases like MongoDB or NoSQL is often required, as agricultural data can be unstructured or semi-structured. Big data technologies empower data scientists to analyze trends and generate predictions on a large scale, enabling more efficient and sustainable farming practices. Knowledge of these technologies ensures that data science applications in agriculture are scalable and capable of handling the vast amounts of data generated by modern farming systems.
Along with general data science tools, a data scientist in agriculture should also be familiar with domain-specific software and tools used in the agricultural industry. These may include farm management software like Ag Leader or Trimble, which are designed to collect and analyze data specific to farming activities. Familiarity with precision agriculture tools and remote sensing equipment, such as drones or soil sensors, is also crucial. Data scientists must know how to integrate these devices and platforms into their models to ensure seamless data flow and accurate results.
For example, knowing how to incorporate satellite imagery or weather data into agricultural models can be pivotal for predictive analytics related to crop yield forecasts or irrigation needs. Being well-versed in these tools ensures that data scientists can build effective and comprehensive solutions tailored to the unique challenges of agriculture, making them more valuable to agribusinesses and farmers.
Lastly, effective communication and collaboration are critical skills for data scientists working in agriculture. These professionals must be able to explain complex technical concepts to non-technical stakeholders, such as farmers, agronomists, or business managers. Clear communication is essential when presenting data insights, interpreting model outputs, or discussing recommendations for improving agricultural practices. Moreover, since data scientists often collaborate with a diverse team of experts, including agronomists, engineers, and software developers, the ability to work well in cross-functional teams is necessary.
Data scientists should be able to translate the needs of farmers into technical requirements, ensuring that data-driven solutions align with agricultural goals. In addition, data scientists must be adept at writing technical reports or creating presentations that clearly convey their findings to stakeholders. Strong communication and collaboration skills ensure that data science solutions are not only technically sound but also practical and beneficial to the agricultural community.
Becoming a data scientist in agriculture requires a combination of technical, analytical, and agricultural knowledge. As the agricultural industry becomes increasingly reliant on data-driven decisions, the demand for data scientists who can analyze and interpret vast amounts of data continues to grow.
These professionals are responsible for creating models that predict crop yields, optimize resource usage, and improve farming practices. To succeed in this field, one must acquire a solid foundation in data science principles and gain expertise in agricultural practices.
The journey involves acquiring the right educational background, gaining practical experience, and developing a deep understanding of agricultural processes. Below are the key steps to becoming a data scientist in agriculture, outlining the education, skills, and experiences necessary to thrive in this field.
The first step to becoming a data scientist in agriculture is obtaining a strong foundation in data science. This typically involves earning a bachelor’s degree in computer science, statistics, mathematics, or a related field. Some aspiring data scientists may also pursue advanced degrees, such as a Master’s or Ph.D. in data science or agricultural technology. During these programs, students gain proficiency in programming languages such as Python, R, and SQL, as well as statistical modeling, machine learning, and data visualization techniques.
Knowledge of these core concepts is essential for building the algorithms and models that will be used in agricultural applications, such as predicting crop performance, managing water resources, or analyzing soil health. Some universities and institutions offer specialized programs in agricultural data science or precision agriculture, providing students with the opportunity to gain both technical and domain-specific expertise. Additionally, completing internships or working on related projects during their education can give students a practical understanding of how data science is applied in agriculture.
While technical skills are critical, gaining practical experience in agriculture is equally important. Understanding agricultural processes, such as crop cycles, irrigation methods, soil conditions, and pest management, is crucial for applying data science to real-world farming problems. Aspiring data scientists should gain hands-on experience by working on farms, participating in agricultural internships, or collaborating with agricultural professionals. By doing so, they can develop a deeper understanding of the challenges faced by farmers and how data can be used to address these issues.
This practical experience helps bridge the gap between technical knowledge and its application in the agricultural sector. Additionally, working alongside agronomists, farmers, and other industry professionals provides valuable insights into the types of data collected in agriculture, such as weather patterns, soil moisture levels, and crop health, and how these datasets can be used to develop effective solutions. Real-world exposure will also help data scientists understand the context in which data is collected, ensuring that the models they build are relevant and practical for the agricultural industry.
To become an effective data scientist in agriculture, it is essential to understand the types of data used in the industry. Agricultural data is often diverse and includes variables like weather data, soil conditions, crop performance, and sensor data collected from farm equipment or IoT devices. Learning how to collect, clean, and analyze this data is crucial for building reliable models. Data scientists should also familiarize themselves with the specific challenges associated with agricultural data, such as its often unstructured or incomplete nature. Understanding the nuances of this data enables data scientists to create accurate models and predictions.
Moreover, it is important to stay informed about emerging technologies in agriculture, such as drones, remote sensing, and satellite imagery, which provide valuable data for analysis. By gaining a deep understanding of the agricultural data landscape, data scientists can identify trends, patterns, and insights that help improve farming practices, optimize resource usage, and drive sustainability in the agricultural sector.
A crucial aspect of becoming a data scientist in agriculture is mastering the tools and technologies used to process and analyze data. Proficiency in programming languages like Python, R, and SQL is essential, as they are used to manipulate, clean, and analyze data. In addition, data scientists must be familiar with machine learning frameworks like TensorFlow, Keras, and Scikit-learn for developing predictive models. Knowledge of big data technologies, such as Apache Hadoop and Spark, is also important, as agriculture generates vast amounts of data that require scalable processing capabilities.
Furthermore, tools like Tableau and Power BI are valuable for creating visualizations that help communicate insights to non-technical stakeholders, such as farmers and agronomists. Additionally, proficiency in cloud platforms such as AWS, Google Cloud, or Microsoft Azure can enable data scientists to store and process large datasets efficiently. By mastering these tools, data scientists in agriculture can ensure that they are equipped to handle the complexities of agricultural data and provide meaningful insights that drive improvements in farming practices.
Once a data scientist has a solid foundation in data science, it is beneficial to specialize in agricultural data science or precision agriculture. Precision agriculture involves using data and technology to optimize farming practices and improve crop yields while minimizing environmental impact. Specializing in this field involves learning how to apply machine learning models, sensors, and remote sensing technologies to monitor and analyze crop health, soil conditions, and weather patterns. Data scientists can further enhance their expertise by gaining certification in precision agriculture or attending workshops and conferences related to agricultural technology.
Specialization allows data scientists to understand the unique challenges of farming and create tailored solutions that improve efficiency and sustainability. It also opens up opportunities to collaborate with agricultural companies, governments, and research institutions that are focused on advancing technology in agriculture. Becoming an expert in agricultural data science can lead to impactful work that directly benefits farmers, the environment, and the global food supply chain.
The field of data science is constantly evolving, with new techniques, tools, and technologies emerging regularly. As such, aspiring data scientists in agriculture need to engage in continuous learning to stay ahead of industry trends. This can be achieved by enrolling in online courses, attending conferences, and participating in webinars related to data science, machine learning, and agriculture. Staying up-to-date with the latest advancements in agricultural technologies, such as AI-powered drones, sensor networks, and autonomous farm equipment, is also essential.
By engaging with the agricultural data science community, professionals can learn about new tools and methods that can enhance their ability to solve real-world agricultural challenges. Furthermore, joining professional organizations and networks can provide access to valuable resources, including research papers, case studies, and job opportunities. By committing to lifelong learning, data scientists can continue to improve their skills and contribute to the advancement of agriculture through data-driven solutions.
Building a portfolio of relevant projects and case studies is an effective way to demonstrate your expertise as a data scientist in agriculture. A strong portfolio not only showcases your technical abilities but also highlights your understanding of agricultural challenges and your ability to apply data science methods to solve real-world problems. Aspiring data scientists can build portfolios by working on personal projects or collaborating with organizations in the agricultural sector. These projects include analyzing crop yield data, creating predictive models for weather forecasting, or developing a recommendation system for irrigation scheduling.
By documenting and sharing these projects, data scientists can attract potential employers or clients who are looking for skilled professionals in the agricultural sector. Having a well-organized portfolio also demonstrates a practical understanding of how data science can directly benefit farming practices. Whether through GitHub repositories or a personal website, a portfolio is a powerful tool for gaining visibility and showcasing expertise in agricultural data science.
Networking and collaboration are essential for data scientists in agriculture to stay connected with industry professionals and gain insights into the latest trends and technologies. Building relationships with farmers, agronomists, and agricultural engineers can help data scientists better understand the challenges facing the industry and tailor their solutions to address specific needs. Networking with professionals in the field also opens up opportunities for collaboration on projects, research, and even job openings. Attending industry conferences, joining online forums, and engaging with agricultural technology startups can provide valuable networking opportunities.
Additionally, collaborating with cross-disciplinary teams, such as those involving agricultural experts, environmental scientists, and data engineers, can lead to innovative solutions that address complex problems. Networking not only provides a chance to learn and grow but also helps in establishing a professional reputation in the agricultural data science community, making it easier to find career opportunities and contribute to meaningful projects.
Data science is transforming agriculture by providing innovative solutions to improve productivity, sustainability, and efficiency. With the integration of advanced technologies like AI, machine learning, and IoT, farmers can make informed decisions that enhance crop yields, optimize resource usage, and mitigate risks associated with climate change.
The continuous evolution of data-driven tools will enable the agricultural industry to tackle challenges like food security and environmental impact more effectively. As we look toward the future, data science will play a critical role in shaping the next generation of farming practices, ensuring a more sustainable and resilient agricultural system.
Copy and paste below code to page Head section
Data science in agriculture refers to the use of data analysis, machine learning, and AI technologies to optimize farming practices. By analyzing data from sensors, weather patterns, and soil conditions, farmers can make informed decisions on crop management, irrigation, and pest control, leading to improved yields and sustainable farming practices.
Data science enhances crop yield by predicting optimal planting times, monitoring soil health, and identifying pest infestations. Using advanced algorithms, farmers can analyze historical data and current conditions to make data-driven decisions that maximize crop productivity and minimize waste. This leads to more efficient use of resources and higher-quality harvests.
Challenges include data accuracy, lack of infrastructure, and resistance to technology adoption. Additionally, agricultural data can be inconsistent or incomplete, making it difficult to build reliable models. Addressing these issues requires collaboration between farmers, tech providers, and policymakers to ensure that data science solutions are accessible and effective in real-world farming environments.
Key technologies include AI, machine learning, remote sensing, IoT sensors, drones, and satellite imagery. These tools enable farmers to collect, analyze, and interpret data to monitor crop health, optimize irrigation, and manage resources efficiently. These technologies are integral to precision agriculture, which seeks to improve productivity while minimizing environmental impact.
Data science helps predict and detect pest and disease outbreaks by analyzing patterns from historical data, weather forecasts, and real-time monitoring. AI-powered models can identify the early signs of pest infestations, enabling farmers to take preventive measures, such as targeted pesticide application, which reduces chemical use and minimizes environmental impact.
Data scientists contribute to sustainable farming by optimizing resource use, improving crop management, and reducing waste. By analyzing data on soil health, water usage, and climate conditions, they help farmers make decisions that conserve water, reduce energy consumption, and lower carbon emissions. This supports environmentally friendly practices and ensures the long-term viability of farming.