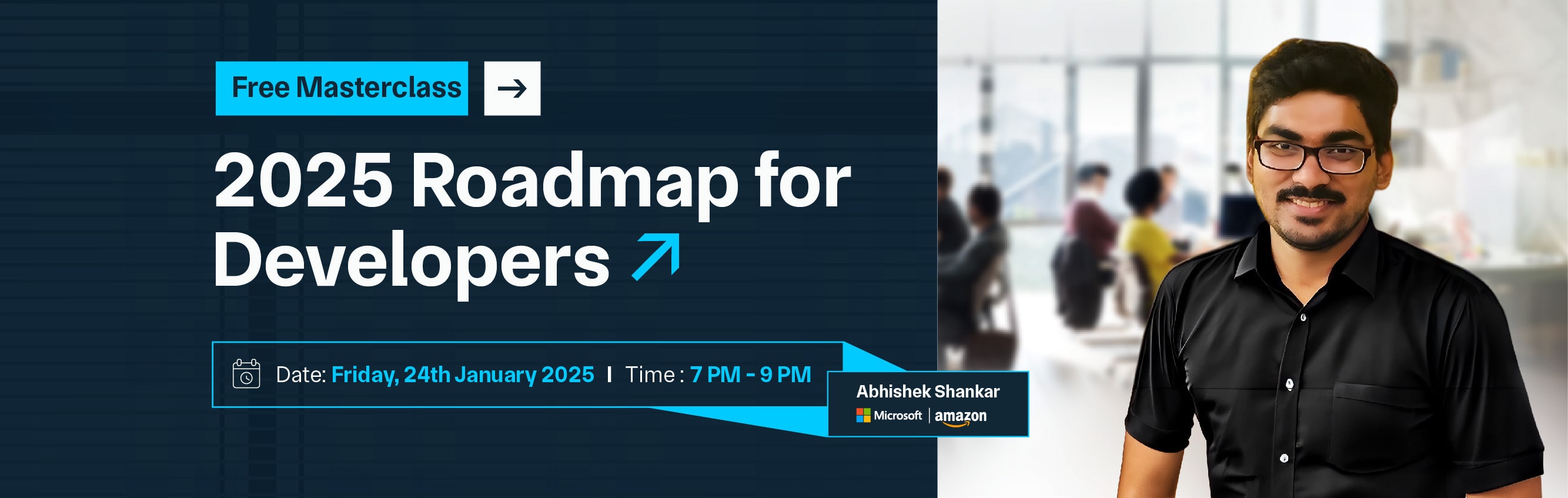

In statistics, data refers to a collection of facts, figures, or information that can be systematically analyzed to uncover insights and inform decision-making. It serves as the foundation for statistical analysis and comes in two primary types: quantitative data and qualitative data. Quantitative data consists of numerical values that can be measured and analyzed mathematically; it can be further categorized into discrete data, which includes countable items (like the number of students in a classroom), and continuous data, which encompasses measurable quantities that can take on any value within a range (such as height or temperature).
On the other hand, qualitative data describes characteristics or qualities that cannot be quantified.This type of data can include nominal categories, which have no intrinsic order (such as types of fruits), and ordinal categories, which carry a meaningful sequence but lack fixed intervals (like satisfaction ratings). Data is crucial in statistics as it enables researchers and analysts to collect, organize, and interpret information.
By employing various statistical methods, including descriptive and inferential statistics, professionals can identify trends, make predictions, and validate hypotheses, ultimately supporting evidence-based decision-making across various domains such as business, healthcare, and social sciences.
Data refers to raw facts, figures, or information collected for analysis or reference. It can take many forms, including numbers, text, images, or audio, and serves as the foundation for knowledge and decision-making. Data is categorized into two main types:
Data is essential for analysis in various fields, including business, science, healthcare, and social research. By organizing and interpreting data through statistical methods, organizations can identify trends, make predictions, and derive insights that guide decision-making and strategy development. Ultimately, data transforms into meaningful information that drives action and understanding.
In statistics, data is categorized into several types based on its characteristics and the way it can be measured or analyzed. Understanding these types is essential for choosing the appropriate statistical methods and accurately interpreting results. The main types of data in statistics are:
Quantitative data consists of numerical values that can be measured and analyzed mathematically. It is further divided into two subcategories:
Qualitative data, also known as categorical data, describes characteristics or qualities that cannot be expressed numerically. This type of data is further categorized into:
Interval data is a type of quantitative data that has both order and equal intervals between values, but it lacks a true zero point. This means that while you can measure the differences between values, you cannot make statements about how many times greater one value is compared to another. A common example is temperature measured in Celsius or Fahrenheit, where the difference between degrees is meaningful, but zero degrees does not indicate the absence of temperature.
Ratio data possesses all the characteristics of interval data, but it includes a true zero point, allowing for meaningful comparisons and calculations of ratios. Examples include weight, height, age, and income. In ratio data, you can say that one value is twice as much as another because zero represents the absence of the quantity being measured.
Qualitative data refers to non-numerical information that captures descriptive characteristics, qualities, or attributes, making it essential for understanding complex phenomena in fields such as social sciences, market research, and healthcare. Unlike quantitative data, which focuses on measurable quantities, qualitative data emphasizes the richness of human experience and perception. It is categorized into two main types: nominal data, which consists of categories without any inherent order such as types of fruits (e.g., apples, bananas) or colors (e.g., red, blue) and ordinal data, which involves ordered categories but lacks consistent intervals between them, such as satisfaction ratings (e.g., very dissatisfied to very satisfied).
Qualitative data is typically collected through methods like interviews, focus groups, surveys with open-ended questions, observations, and content analysis, allowing researchers to gather in-depth insights and explore participants' thoughts and feelings. Analyzing qualitative data often involves techniques such as thematic analysis, where recurring themes are identified; coding, which organizes responses into categories; and narrative analysis, which examines personal stories for deeper understanding.
The significance of qualitative data lies in its ability to provide context and meaning behind numbers, revealing motivations, behaviors, and social dynamics that are crucial for informed decision-making and effective strategies in various domains. By capturing the nuances of human experience, qualitative data enriches our understanding of the world, enabling researchers and organizations to address complex issues more holistically.
Quantitative data refers to numerical information that can be measured, counted, and expressed mathematically, making it essential for statistical analysis and comparison across various fields, such as science, economics, psychology, and healthcare. This type of data is categorized into two main types: discrete data, which consists of countable, distinct values, such as the number of students in a classroom or the number of cars in a parking lot, and continuous data, which can take any value within a range and can be measured to any level of precision, such as height, weight, or temperature.
Quantitative data is typically collected through structured methods like surveys with closed-ended questions, controlled experiments, observational studies, and existing records. Analyzing this data involves techniques such as descriptive statistics, which summarize key measures like mean and standard deviation, and inferential statistics, which allow researchers to make predictions or inferences about a population based on sample data.
The strength of quantitative data lies in its objectivity and measurability, providing reliable insights that facilitate evidence-based decision-making. By quantifying complex phenomena, it reveals patterns and trends, enabling researchers and organizations to understand behaviors, evaluate performance, and drive innovation effectively.
Quantitative and qualitative data are two fundamental types of information used in research and analysis, each serving distinct purposes and providing unique insights. Quantitative data focuses on numerical values that can be measured and analyzed statistically, making it ideal for identifying patterns and testing hypotheses.
In contrast, qualitative data captures non-numerical descriptions, emphasizing depth and context to explore underlying motivations and experiences. Understanding the differences between these two data types is crucial for researchers and decision-makers, as both play important roles in gathering comprehensive insights and informing strategies across various fields.
Discrete data involves countable, distinct values, while continuous data encompasses measurable values that can take on any number within a range. Understanding these differences is crucial for selecting appropriate statistical methods and accurately interpreting results in various fields.
understanding the distinction between discrete and continuous data is essential for effective data analysis and interpretation. Discrete data, characterized by countable, distinct values, is ideal for scenarios involving specific quantities, such as the number of people or items. In contrast, continuous data allows for a broader range of values and is crucial for measurements that require precision, such as weight or height.
Recognizing these differences enables researchers and analysts to select appropriate statistical methods and accurately represent their findings. Ultimately, both types of data play significant roles in quantitative research, contributing to informed decision-making and a deeper understanding of various phenomena.
Copy and paste below code to page Head section
Discrete data consists of countable, distinct values, typically whole numbers, while continuous data can take any value within a range and includes fractions and decimals.
Sure! Examples of discrete data include the number of students in a class, the number of cars in a parking lot, and the number of votes in an election.
Examples of continuous data include height, weight, temperature, and time, where values can vary infinitely within a certain range.
Discrete data is often collected through counting (e.g., surveys with specific options), while continuous data is typically gathered through measurement (e.g., scales, rulers).
Common methods for analyzing discrete data include frequency distributions, chi-square tests, and bar graphs.
Continuous data analysis often involves techniques such as regression analysis, t-tests, and histograms to assess distributions and relationships.