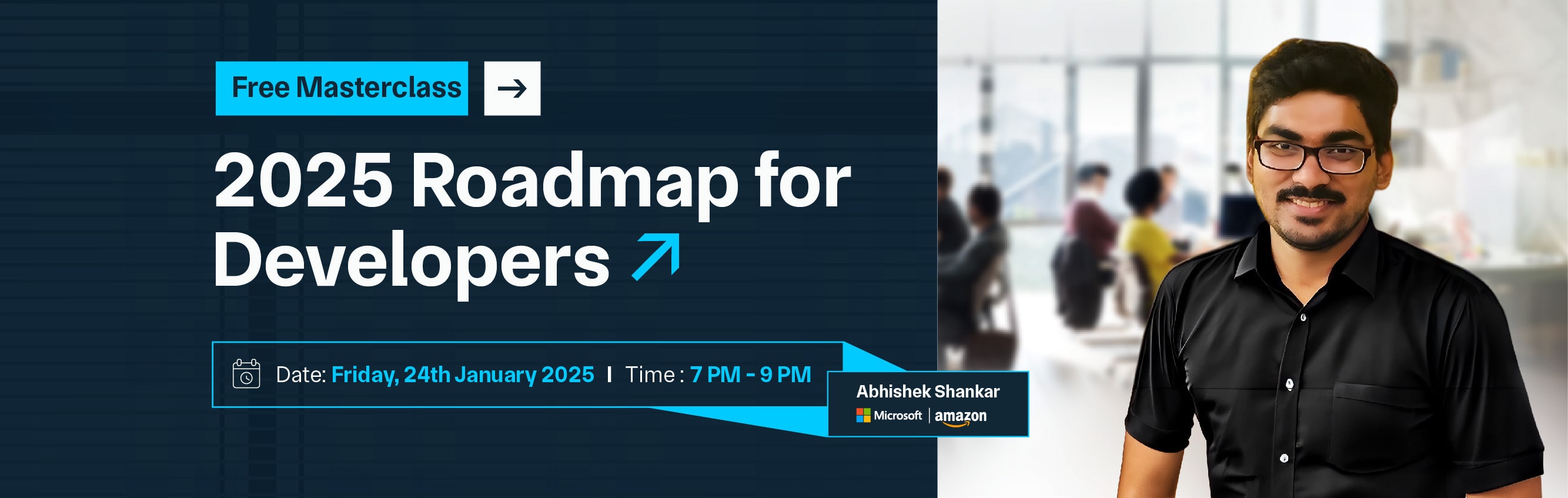

The career path of a data engineer is both dynamic and rewarding, offering a vital role in the ever-growing field of data-driven decision-making. Data engineers are responsible for designing, building, and maintaining systems that allow organizations to manage vast amounts of data efficiently. These professionals play a key role in ensuring data accessibility, reliability, and security, which serves as the backbone of analytics and business intelligence. With businesses increasingly relying on data, the demand for skilled data engineers continues to rise.
A career in data engineering typically begins with foundational knowledge in computer science, programming, and database management. Entry-level positions may include roles such as Junior Data Engineer or Data Analyst, which provide opportunities to develop expertise in data pipelines, ETL (Extract, Transform, Load) processes, and cloud technologies. As professionals gain experience, they can transition to senior roles, working on more complex data architectures, managing big data technologies, and optimizing system performance.
With further specialization, data engineers can advance into leadership roles such as Data Engineering Manager or transition into related fields like data science or machine learning engineering. Continuous learning is essential in this field, as emerging tools and techniques constantly shape the industry. A data engineering career offers excellent growth prospects, competitive salaries, and the satisfaction of making a tangible impact on organizational success.
A data engineer is a specialized professional who designs, builds, and maintains the infrastructure needed to store, process, and analyze large volumes of data. Their primary role is to create robust data pipelines that enable seamless movement of data from various sources to destinations where it can be utilized effectively.
Data engineers work with diverse tools and technologies, including databases, cloud platforms, and big data frameworks like Apache, Hadoop, and Spark. Their focus lies in ensuring that the data is clean, organized, and ready for use by data analysts, scientists, and other stakeholders. In addition to technical expertise, data engineers excel in problem-solving and system optimization.
They are responsible for addressing challenges related to data scalability, reliability, and security. Their work often involves collaborating with cross-functional teams to understand business needs and tailor data solutions accordingly. As organizations increasingly rely on data to drive decisions, the role of a data engineer has become critical in enabling insights that lead to innovation and efficiency. This career path offers a unique blend of creativity and technical mastery, making data engineers indispensable in today’s data-centric world.
A data engineer is responsible for building and maintaining the systems that enable organizations to manage and analyze data effectively. They handle the end-to-end flow of data, ensuring that information is accessible, clean, and usable for business insights.
From designing data pipelines to implementing storage solutions, their work involves a deep understanding of databases, cloud platforms, and big data technologies. In addition to technical implementation, data engineers also focus on optimizing performance and ensuring data security.
Their efforts ensure that organizations can handle growing amounts of data efficiently. Working closely with analysts, scientists, and developers, they enable accurate and timely decision-making by delivering high-quality data. Below are the key responsibilities of a data engineer:
Pursuing a career in data engineering offers an exciting opportunity to be at the forefront of the data revolution. In today’s data-driven world, organizations rely on data engineers to build the infrastructure that powers analytics, machine learning, and decision-making.
This career combines technical expertise, creativity, and problem-solving, providing a dynamic and fulfilling work environment. The demand for skilled data engineers continues to grow as businesses generate and utilize increasing amounts of data.
This field not only provides job security but also offers lucrative salaries and opportunities for career advancement. Additionally, data engineers play a crucial role in shaping how companies operate and innovate, making their work highly impactful. Below are key reasons to pursue this career:
Data engineering is a lucrative field, with salaries reflecting the high demand for skilled professionals. On average, entry-level data engineers in the United States can earn between $70,000 and $90,000 annually, while mid-level professionals typically make $100,000 to $120,000 per year. Experienced data engineers and specialists in advanced technologies, such as big data or cloud platforms, often command salaries exceeding $140,000.
These figures may vary based on location, industry, and the complexity of the role, with cities like San Francisco, New York, and Seattle offering some of the highest pay scales. Globally, the demand for data engineers ensures competitive pay across various markets. For instance, data engineers in countries like the UK and Germany earn between £50,000–£80,000 and €60,000–€90,000 annually, respectively.
In India, salaries for skilled data engineers range from ₹6 LPA to ₹25 LPA, depending on experience and expertise. Furthermore, professionals with certifications in tools like Apache Spark, AWS, or Snowflake often enjoy higher salaries due to their specialized skills. These earnings highlight the potential for financial growth and job security in this ever-evolving career.
The career path of a data engineer offers many growth opportunities, thanks to the increasing demand for skilled professionals in the data field. Starting from entry-level positions, data engineers build experience in managing and processing vast amounts of data, designing data pipelines, and ensuring data quality.
With time, they advance into more specialized and senior roles, where they manage complex data systems, lead teams, and drive innovation in data infrastructure. As organizations increasingly rely on data for decision-making, data engineers are crucial in supporting the entire data lifecycle, from collection to analysis.
The progression from junior roles to senior leadership positions can be achieved by continuously developing technical expertise and leadership abilities, making the data engineering field both rewarding and dynamic.
Becoming a data engineer requires a blend of education, technical skills, and hands-on experience. Data engineers are responsible for building and maintaining data systems, ensuring the flow of data across various platforms, and preparing it for analysis. As organizations continue to rely heavily on data for decision-making, the demand for skilled data engineers has grown significantly.
To pursue a career in data engineering, it is essential to acquire a strong foundation in computer science, programming, and database management. Additionally, gaining proficiency in big data tools, cloud platforms, and ETL (Extract, Transform, Load) processes is crucial for success.
Hands-on experience through internships, projects, or contributions to open-source initiatives plays a key role in developing practical skills. The following steps outline how to become a data engineer, focusing on education, technical expertise, and practical experience that will help you build the necessary skills for this rapidly growing field.
A strong educational foundation is the first step to becoming a data engineer. Most data engineers pursue a degree in computer science, information technology, or related fields to gain a deep understanding of algorithms, data structures, and systems design. The coursework typically covers topics like databases, programming languages, and statistics, which are critical for a successful career in data engineering. A bachelor's degree is generally the minimum requirement, though additional qualifications like a master's degree or certifications can enhance your skills.
In addition to formal education, gaining knowledge of specialized data engineering topics is vital. Topics like distributed computing, data architecture, and cloud services are essential for working with big data systems. By combining educational qualifications with hands-on experience, aspiring data engineers are better prepared to take on complex technical challenges and contribute to the field’s advancements.
Proficiency in programming languages is critical to excelling in data engineering. Learning languages such as Python, Java, or Scala equips data engineers with the ability to manipulate large datasets, automate tasks, and build scalable solutions. Python is particularly popular in data engineering due to its simplicity and vast library of data processing tools. Java and Scala, on the other hand, are essential for working with big data frameworks like Apache Hadoop and Apache Spark, enabling engineers to handle massive datasets efficiently.
Mastering the syntax, libraries, and frameworks of these languages allows data engineers to build and maintain robust data pipelines. Understanding how to integrate different programming languages with data systems helps engineers optimize performance, reduce redundancy, and solve problems more effectively. As data engineering often involves building custom solutions, mastering these languages is a cornerstone for success in this field.
Database management is at the heart of data engineering. A data engineer must be proficient in managing both relational and NoSQL databases, such as SQL, MySQL, MongoDB, or Cassandra. Understanding how to design, maintain, and optimize databases ensures that data is stored efficiently and can be accessed quickly. SQL is critical for managing relational data, while NoSQL databases are used for handling unstructured data or large-scale distributed systems.
A deep knowledge of database design principles, such as indexing, normalization, and query optimization, is essential. A data engineer must also ensure data consistency and integrity across various systems. Understanding the intricacies of data modeling and database scaling allows engineers to create robust solutions that handle large volumes of data while maintaining accessibility and speed.
Data engineering relies heavily on a suite of tools and frameworks for processing and managing large datasets. Big data tools like Apache Hadoop and Apache Spark are widely used to process massive amounts of data in distributed computing environments. Hadoop is designed for batch processing, while Spark handles both batch and real-time processing, making it highly flexible for various data engineering tasks.
Mastering these tools, along with other technologies such as Apache Kafka for stream processing and Apache Airflow for workflow automation, is vital. Data engineers use these frameworks to build scalable data pipelines, manage data workflows, and ensure smooth data processing operations. Familiarity with these tools enables engineers to meet the challenges of modern data engineering, such as working with large-scale, high-velocity data systems.
Cloud platforms are increasingly becoming the backbone of modern data infrastructure, and data engineers must be adept at using them. Leading cloud platforms like AWS, Google Cloud, and Microsoft Azure offer an array of services tailored for data storage, processing, and analytics. Data engineers need to be familiar with cloud data warehouses like Amazon Redshift, Google BigQuery, or Azure Synapse Analytics, which help manage and analyze data at scale.
Cloud computing offers the flexibility to build cost-effective, scalable data architectures. By understanding serverless computing, cloud-native data pipelines, and cloud storage options, data engineers can design systems that are efficient, resilient, and scalable. Mastery of cloud platforms ensures data engineers are well-equipped to handle the ever-growing volume and complexity of data in today’s cloud-first world.
Data pipelines are the backbone of a data engineering workflow, as they allow for the seamless movement of data between systems. ETL (Extract, Transform, Load) processes are central to this. Data engineers design and maintain pipelines that extract data from various sources, transform it into the necessary format, and load it into databases or data warehouses. These processes ensure data is clean, organized, and ready for analysis.
A data engineer must be skilled in optimizing these workflows, ensuring data flows reliably and without delays. Tools like Apache NiFi, Talend, or custom scripts are often used to automate these processes. Building efficient data pipelines minimizes errors and enhances the overall speed and performance of data systems. Streamlining these processes is essential for ensuring that data is consistently available for real-time or batch-processing tasks.
As technology evolves, so does the field of data engineering. Emerging trends such as machine learning, artificial intelligence, and real-time data processing continue to reshape how data is handled and processed. To remain competitive, data engineers must stay informed about new tools, frameworks, and techniques that can improve the efficiency and scalability of data systems.
Engaging in continuous learning through conferences, industry blogs, online courses, and certifications is crucial. By staying up to date with advancements in the field, data engineers ensure that their skills remain relevant and they can adapt to new challenges. Whether it’s exploring the potential of quantum computing in data processing or learning the latest in cloud-native data solutions, being proactive about learning helps data engineers maintain their edge in an ever-changing field.
Practical experience is essential for mastering the skills needed to be a successful data engineer. Internships, freelance work, or personal projects provide hands-on opportunities to work with real data and develop problem-solving skills. Data engineers who engage in practical projects can build a strong portfolio that showcases their expertise and technical proficiency.
Working on personal projects, such as building data pipelines, optimizing databases, or implementing real-time data solutions, allows you to apply theoretical knowledge in real-world scenarios. Contributing to open-source projects, collaborating with other data engineers, or gaining experience through internships can also help build confidence and improve your ability to work in a team environment, preparing you for the challenges of a full-time data engineering role.
A data engineer plays a crucial role in managing and optimizing the flow of data within an organization. They are responsible for designing, building, and maintaining the infrastructure that allows data to be collected, processed, and stored efficiently. Data engineers ensure that data is clean, accessible, and ready for analysis by data scientists and business analysts.
Their work involves building scalable data pipelines, managing data warehousing solutions, and implementing efficient data storage strategies. Data engineers also focus on optimizing system performance and ensuring data security. They work closely with data scientists, analysts, and IT teams to align technical infrastructure with organizational needs and business goals.
The role requires a strong understanding of programming, databases, cloud computing, and data integration tools. Data engineers provide the foundational systems that empower organizations to make data-driven decisions, improve processes, and unlock the full potential of their data assets.
A successful data engineer must possess a diverse set of technical and analytical skills. As data engineering plays a critical role in ensuring data is properly stored, processed, and made available for analysis, having the right skills is essential. Data engineers are responsible for building robust data pipelines, working with databases, and integrating various data sources.
To excel in this role, professionals need a deep understanding of data management principles, strong programming capabilities, and proficiency in various data engineering tools and frameworks. Moreover, data engineers must be able to collaborate with data scientists, analysts, and business stakeholders to ensure data-driven decision-making processes are streamlined.
In this rapidly evolving field, it's also vital to stay updated on emerging technologies and industry best practices. The following key skills are essential for any aspiring data engineer to succeed and make a significant impact in the field of data engineering.
Programming forms the foundation of a data engineer's work. Proficiency in languages like Python, Java, or Scala is crucial for building and maintaining efficient data pipelines. Python, for example, is widely used for automating tasks, manipulating datasets, and integrating with data processing tools. Java and Scala are particularly important when working with big data frameworks like Hadoop and Spark.
A solid understanding of these languages enables data engineers to design scalable and optimized solutions for data management. Mastering these languages also aids in making code reusable and efficient for handling large data sets. Additionally, the ability to choose the appropriate language for the task at hand based on performance requirements is essential for optimizing workflow.
Data engineers need an in-depth knowledge of both relational (SQL) and non-relational (NoSQL) databases. SQL expertise is essential for managing structured data, writing complex queries, and optimizing performance. NoSQL databases, such as MongoDB and Cassandra, are important when handling unstructured or semi-structured data. Data engineers must be able to design, implement, and maintain databases, ensuring data is accessible, secure, and efficiently retrievable for analysis.
Mastering database management ensures smooth data storage and retrieval processes. Beyond just querying, data engineers must optimize database schemas for performance and scalability. Understanding indexing, partitioning, and data replication further enhances the ability to build efficient systems capable of handling large datasets across various platforms.
As more businesses migrate to the cloud, cloud computing platforms like AWS, Microsoft Azure, and Google Cloud are becoming integral to data engineering. A deep understanding of cloud services is vital for building scalable, cost-effective, and flexible data architectures. Data engineers need to be proficient in utilizing cloud storage, computing resources, and serverless architectures to process and store large datasets.
Cloud platforms enable seamless data integration and collaboration across teams, making them a critical skill for data engineers. Moreover, cloud-based solutions often come with security and compliance features that are essential for managing sensitive data. Cloud providers also offer specialized data tools like AWS Redshift, Google BigQuery, and Azure Data Lake, which data engineers can leverage to build sophisticated data pipelines and improve system efficiency.
Data engineers are responsible for designing and maintaining data pipelines, which include the Extract, Transform, and Load (ETL) processes. Mastery of ETL tools, such as Apache NiFi, Talend, or custom-built solutions, is essential for moving data between systems, transforming it into a usable format, and loading it into databases or data warehouses.
Data engineers must ensure that these pipelines are efficient, reliable, and scalable, ensuring smooth data flow for downstream processing or analytics. Efficient pipeline architecture allows data to be processed in real time, ensuring that teams can access up-to-date insights. Additionally, the ability to automate the ETL process and optimize its performance is crucial for meeting business demands. Being adept at troubleshooting pipeline issues and ensuring data integrity is key to maintaining smooth operations.
As data volumes continue to grow, familiarity with big data technologies like Apache Hadoop, Apache Spark, and Kafka is crucial. These frameworks help data engineers handle large-scale data processing, distributed data storage, and real-time data streaming. Hadoop and Spark are used for batch processing, while Kafka facilitates real-time data streaming. By mastering these tools, data engineers can efficiently process, store, and analyze large datasets, ensuring timely data delivery for analysis and decision-making.
Understanding how to leverage the distributed computing capabilities of Hadoop and Spark also helps engineers scale data processing capabilities. Moreover, Kafka’s ability to manage and process data streams in real time supports the growing demand for faster and more dynamic data analytics, making it an essential skill in data engineering.
A solid understanding of data modeling and data warehousing principles is essential for designing efficient storage systems. Data engineers must be proficient in designing models representing the relationships between data elements and optimizing storage systems for fast querying and analysis. Data warehousing involves integrating data from multiple sources into a central repository, enabling business intelligence tools to access consolidated data.
Data engineers ensure that data is structured in a way that facilitates quick, accurate analysis. Data modeling also involves defining data relationships and ensuring that data is normalized to prevent redundancy and ensure consistency. A well-structured data warehouse is a crucial asset for any organization, as it facilitates the ability to access and query historical data in a structured and optimized manner.
Automation plays a crucial role in data engineering, as it reduces manual workloads and enhances efficiency. Data engineers should have strong scripting skills to automate repetitive tasks such as data extraction, transformation, and loading. Automation also extends to monitoring data pipelines, managing data workflows, and scheduling tasks.
Proficiency in scripting languages like Python, Bash, or PowerShell can help data engineers streamline processes, improve reliability, and ensure data integrity throughout the pipeline lifecycle. By automating manual tasks, data engineers can increase productivity and reduce the likelihood of human error. Furthermore, building automated testing frameworks and monitoring tools ensures that data workflows run smoothly and issues can be detected and resolved promptly.
Ensuring the security and privacy of data is paramount for data engineers. They need to implement encryption techniques, secure data storage, and adhere to privacy regulations such as GDPR. Understanding data governance and best practices for data access management helps data engineers safeguard sensitive information. Data engineers must also collaborate with security teams to identify vulnerabilities, implement access controls, and maintain compliance with industry standards to protect data from breaches and misuse.
Additionally, they must stay informed about emerging security technologies to protect data from new threats. Ensuring compliance with data protection laws requires careful attention to detail and the integration of security practices into every stage of the data engineering lifecycle. Data engineers must proactively manage and audit data access and usage to prevent unauthorized data exposure.
Strong analytical skills are crucial for data engineers to troubleshoot issues and optimize data workflows. Data engineers need to understand complex systems and quickly identify bottlenecks, errors, or inefficiencies in data pipelines. Problem-solving abilities enable them to devise solutions for data storage, retrieval, and processing challenges.
A data engineer’s ability to think critically and apply systematic approaches to solving problems is key to ensuring data systems run smoothly and deliver timely insights for business decisions. Additionally, having an analytical mindset allows engineers to improve existing systems and design better solutions for data processing workflows. When encountering data inconsistencies or system failures, data engineers must be able to quickly identify the root cause and implement a solution to prevent future issues.
Data engineers often work in cross-functional teams with data scientists, analysts, and business stakeholders. Effective communication skills are essential for understanding requirements, explaining technical concepts to non-technical audiences, and ensuring smooth collaboration across teams. Data engineers need to translate business needs into technical solutions and collaborate closely to ensure data pipelines and storage solutions align with business objectives.
Being able to present complex data engineering processes clearly and concisely is key to success in this role. Moreover, strong collaboration skills are necessary for understanding how data systems align with business goals and how to make data available for decision-making processes. Being proactive in communicating data infrastructure challenges ensures that teams can work together to find solutions effectively.
Certifications play a crucial role in securing a data engineering job as they validate the technical expertise and knowledge of candidates, making them stand out in a competitive job market. While a degree can provide a solid foundation, certifications demonstrate specialized skills that align with industry requirements. In the fast-evolving field of data engineering, certifications also show that professionals are keeping up with the latest tools, technologies, and best practices.
They not only increase a candidate's credibility but also help potential employers gauge their expertise in specific areas like cloud platforms, big data technologies, and data pipelines. With the growing demand for skilled data engineers, certifications can be the differentiator between being considered or passed over for a job.
In addition to boosting employability, certifications help professionals deepen their knowledge, fill gaps in their skills, and ensure they are equipped to handle the challenges of modern data engineering roles. Below are the key ways in which certifications can benefit aspiring data engineers.
Certifications provide a formal validation of the skills a candidate claims to have, making them more attractive to hiring managers. A certified data engineer has demonstrated proficiency in key areas such as database management, data modeling, and cloud computing platforms. Certifications show that candidates not only possess theoretical knowledge but have also mastered practical applications of these skills.
By passing certification exams, data engineers prove their competency in using industry-standard tools and technologies, which boosts their credibility in the job market. Moreover, certifications offer a competitive edge in crowded job markets where employers are looking for proven expertise to handle large-scale data systems and manage complex data workflows efficiently. As such, certifications act as a form of professional endorsement, reassuring employers about the candidate's capabilities.
Data engineering requires hands-on experience with a variety of tools and technologies, many of which are constantly evolving. Certifications, especially those from recognized organizations such as AWS, Google Cloud, or Microsoft, expose professionals to the latest industry tools and best practices. For example, certifications in cloud platforms provide deep insights into how to leverage cloud-based infrastructure for data storage, processing, and analysis. Candidates preparing for certifications learn the technicalities of tools like Apache Hadoop, Apache Spark, and database technologies, which are critical for the day-to-day responsibilities of a data engineer.
Through certification preparation, individuals familiarize themselves with the most recent developments in the field, ensuring they stay up-to-date and equipped to tackle the challenges that come with managing modern data environments. This knowledge translates directly into real-world applications, where candidates can effectively design, optimize, and maintain complex data systems.
As data engineering continues to grow in importance, the competition for roles in this field has become more intense. A certification can provide the necessary edge to stand out from other candidates. In many cases, companies prefer hiring candidates who have demonstrated their knowledge through certifications, as they guarantee that the individual has undergone rigorous testing in specialized areas. When hiring managers have numerous resumes to sift through, a certification often makes the difference between two equally qualified candidates.
Additionally, certifications in popular data engineering platforms like AWS, Google Cloud, and Microsoft Azure indicate that the candidate is familiar with tools widely used across industries. Employers are more likely to hire data engineers with certifications because it indicates that they are capable of working with enterprise-level technologies and can add immediate value to the team. The enhanced competitiveness opens doors to more opportunities and roles in the data engineering field.
Data engineers with certifications are often able to negotiate better salaries due to their specialized skill set. The added knowledge and proficiency demonstrated through certification programs justify higher compensation, as employers are willing to pay a premium for certified professionals who can manage critical data infrastructure and complex workflows. A certification in a high-demand tool or technology, such as big data processing platforms or cloud services, can lead to salary increases or offer access to higher-paying roles.
Moreover, certifications are often seen as a commitment to ongoing learning, which indicates that a candidate is proactive in staying updated and improving their skill set. This professional growth is valuable to employers, who are often willing to invest more in certified data engineers. Certified professionals are also viewed as being more productive, making them worthy of higher pay compared to those without certifications.
Certifications can provide data engineers with access to exclusive professional networks, which can open doors to further career opportunities. Many certification programs, especially those offered by major technology providers like AWS or Microsoft, come with access to a community of professionals, online forums, and events. These networks allow certified individuals to exchange ideas, collaborate, and stay updated on the latest trends in the industry. Networking within these communities can lead to job opportunities, mentorship, and partnerships.
Additionally, certifications often come with badges or recognition that can be showcased on LinkedIn profiles or resumes, helping to make the certified individual more visible to recruiters and potential employers. For professionals looking to advance in their careers, certifications can help them transition into leadership roles, such as data engineering team leads or architects, by showcasing their expertise and commitment to continuous learning.
Pursuing certifications provides a structured path for learning and skill development. Certification programs are typically designed to cover essential topics and technologies that are relevant to the data engineering profession. By following a certification curriculum, candidates gain a comprehensive understanding of the foundational concepts, industry practices, and specific tools that are required for the role. This structured approach helps ensure that individuals take advantage of key areas of knowledge that are vital for their career success.
The process of preparing for a certification exam encourages individuals to acquire in-depth knowledge of data engineering concepts, often filling knowledge gaps and reinforcing their existing skills. Structured learning also helps individuals keep track of their progress and ensures they stay on track to achieve their career goals. Through certifications, professionals are able to approach their learning methodically, optimizing their ability to master complex topics.
Certifications reflect a strong commitment to professional growth and continuous learning. In a rapidly evolving field like data engineering, staying current with the latest technologies and best practices is essential. By obtaining certifications, data engineers show employers that they are dedicated to improving their skills and advancing their knowledge. This proactive approach to learning enhances a candidate’s value to the organization, as it indicates a genuine interest in the role and a desire to contribute effectively.
Certifications can also demonstrate a data engineer's long-term commitment to their career, as they take the time and effort to invest in formal education beyond their initial degree. This dedication to professional development can build a data engineer’s reputation as a reliable and forward-thinking employee who is motivated to stay at the forefront of technological advancements.
For individuals looking to switch careers into data engineering, certifications can serve as a useful bridge. Certifications offer a tangible demonstration of relevant skills and knowledge, which can help professionals transition from another field into data engineering. For instance, someone with a background in software development or IT might pursue certifications in big data or cloud computing platforms to gain the necessary technical skills for a data engineering role.
By completing certifications, career switchers can showcase their expertise to potential employers, easing their entry into the field. Certifications help bridge the gap between prior experience and the specific demands of data engineering, providing a stepping stone toward a new career. They serve as proof that candidates are serious about making the transition and possess the required competencies to succeed in their new role.
The data engineering field is dynamic, with new tools, techniques, and technologies emerging regularly. Certifications ensure that data engineers stay updated with the latest industry standards and best practices. For example, certifications from major cloud providers or big data platforms are regularly updated to reflect the newest features and capabilities of these technologies. By obtaining these certifications, professionals can keep pace with the ongoing changes in the field.
Moreover, staying updated on industry trends through certifications ensures that data engineers remain relevant and capable of handling modern data challenges. Continuous learning and obtaining certifications allow data engineers to work with cutting-edge technologies, optimizing data pipelines, storage systems, and workflows using the best available solutions.
Certifications provide an added layer of job security, particularly in fields where technical skills are constantly evolving. By investing in certifications, data engineers demonstrate that they have the expertise necessary to meet the current and future demands of the job. Employers value professionals who are not only competent but also committed to improving their skills over time.
In an environment where technologies and tools evolve rapidly, certified data engineers are more likely to be seen as valuable, making it less likely that they will be replaced. Additionally, certifications in specific technologies often make data engineers more resilient to market changes, as their specialized skills are in demand across multiple industries and organizations. This increased job security ensures that certified professionals are better equipped to weather economic fluctuations or shifts in the job market.
Data engineering is rapidly evolving and will continue to play a critical role in the data-driven future of industries across the globe. As the amount of data generated grows exponentially, organizations will require advanced data engineering practices to manage, process, and analyze this information efficiently. Emerging technologies like artificial intelligence, machine learning, and advanced data pipelines are transforming the landscape, making data engineering more sophisticated.
The future of data engineering will also see a stronger emphasis on automation, cloud infrastructure, and real-time data processing to support faster decision-making and improved business intelligence. Data engineers will be expected to work closely with data scientists, analysts, and IT teams to ensure that organizations can harness the full potential of their data.
Additionally, privacy and security concerns will drive the development of new tools and methodologies to ensure compliance and safeguard sensitive information. Below are key trends that will shape the future of data engineering and its impact on the industry.
Cloud computing has already become a major player in data engineering, and its importance is only going to increase in the future. Cloud platforms like AWS, Google Cloud, and Microsoft Azure are offering scalable and cost-effective solutions that allow businesses to store, manage, and analyze vast amounts of data. As more organizations shift towards cloud infrastructure, data engineers will be expected to develop expertise in cloud-based tools and services. The future of data engineering will involve creating robust data pipelines that leverage cloud storage, processing, and analytics tools.
Data engineers will need to ensure data is seamlessly transferred between on-premise and cloud environments and optimize cloud infrastructure to handle large-scale data workloads. The demand for skilled data engineers proficient in cloud technologies will continue to rise as businesses move away from traditional on-premise solutions to more flexible, cloud-based models. Cloud platforms also support innovation in real-time data processing, which will become increasingly important in data engineering roles.
Automation is transforming data engineering, reducing manual effort, and improving the speed and efficiency of data processing tasks. In the future, more data engineering processes will be automated using advanced tools and frameworks. This includes automating data pipelines, data integration, and ETL (Extract, Transform, Load) processes, which are traditionally time-consuming and error-prone. Automation will also play a key role in data monitoring and data quality management, allowing data engineers to focus on more strategic tasks rather than manual maintenance.
The integration of artificial intelligence (AI) and machine learning (ML) with automation tools will allow for smarter and more adaptive data workflows. These intelligent systems will be able to detect anomalies, predict issues, and even optimize data pipelines automatically. As automation takes over routine tasks, data engineers will have more time to focus on complex problem-solving, system architecture, and innovation. Automation will enable data engineers to scale their operations, handle larger datasets, and maintain more efficient systems.
The future of data engineering will focus heavily on real-time data processing and streaming as businesses strive to make faster, data-driven decisions. Traditional batch processing methods are needed to handle the growing demand for timely, actionable insights. Technologies like Apache Kafka, Apache Flink, and AWS Kinesis are enabling real-time data processing, allowing organizations to collect and analyze data as it is generated. In the future, data engineers will need to design and maintain systems that can process streaming data in real time, integrating it seamlessly with data warehouses and databases.
This shift toward real-time analytics will require data engineers to become proficient in handling high-velocity data, as well as optimizing systems to ensure low-latency processing. Real-time data pipelines will allow businesses to respond immediately to customer needs, market changes, and operational demands. Data engineers will be at the forefront of developing and managing these systems, ensuring that data flows efficiently and is processed instantly.
As organizations deal with increasingly complex data from a wide variety of sources, the need for advanced data pipelines and data integration techniques will continue to grow. In the future, data engineers will need to design pipelines that are capable of handling diverse data types, such as structured, semi-structured, and unstructured data, all while ensuring seamless data flow across different systems and platforms. Integrating data from disparate sources will be a major focus, as businesses need to combine data from IoT devices, social media, transactional systems, and more to gain a holistic view of their operations.
Data engineers will play a key role in developing efficient ETL and ELT processes, enabling organizations to extract, transform, and load data into centralized repositories like data lakes and warehouses. These pipelines must also be optimized for scalability and speed, ensuring they can handle increasing volumes of data without sacrificing performance. The development of more flexible and scalable data architectures will be a critical skill for data engineers in the coming years.
With data breaches and privacy concerns becoming more prevalent, data engineers will face increasing pressure to ensure that sensitive data is handled securely and in compliance with regulations like GDPR and CCPA. The future of data engineering will see a heightened focus on data security and privacy. Data engineers will need to design systems that incorporate strong encryption, access control, and data masking techniques to safeguard data both at rest and in transit. Additionally, as organizations store more personal and sensitive data in the cloud, ensuring compliance with data privacy regulations will become a crucial responsibility.
Data engineers will be expected to implement security measures that protect against unauthorized access and prevent data leaks. With the growing importance of cybersecurity, professionals in data engineering will need to collaborate closely with security teams to develop solutions that balance operational efficiency with robust data protection. This will include adopting best practices for secure data storage, handling, and sharing across cloud environments.
The integration of artificial intelligence (AI) and machine learning (ML) into data engineering workflows will become a dominant trend. Data engineers will need to build systems that can handle and process data to support AI and ML models effectively. This includes creating data pipelines that can feed clean, structured data into machine learning algorithms for training, as well as managing the data lifecycle throughout the ML process.
As AI and ML models are increasingly used for tasks like predictive analytics, recommendation engines, and anomaly detection, data engineers will be tasked with optimizing data storage, retrieval, and processing to meet the specific needs of these models. Additionally, data engineers will collaborate with data scientists to ensure that the infrastructure is in place to support complex ML workflows. The future of data engineering will require a blend of traditional engineering skills and knowledge of AI/ML concepts, making it essential for data engineers to expand their skills in these areas.
The future of data engineering will involve greater collaboration between data engineers and data scientists. As data engineering becomes more complex and data science techniques grow in sophistication, both teams will need to work together more closely to create seamless, end-to-end data workflows. Data engineers will be responsible for building the infrastructure that allows data scientists to access and analyze large datasets efficiently.
They will also ensure that the data is clean, reliable, and in a format suitable for analysis. In the future, data engineers will need to adopt a more cross-disciplinary approach, collaborating with data scientists, software engineers, and business analysts to deliver data-driven solutions. This collaboration will drive better business outcomes as data engineering and data science teams work together to create high-performance data systems and advanced analytics models. As data complexity increases, the ability of these teams to work cohesively will be essential for success.
As organizations collect and store more data, ensuring proper data governance and compliance will become an increasingly important aspect of data engineering. Data engineers will need to ensure that data is properly categorized, tagged, and stored in accordance with governance policies, ensuring it is accessible, traceable, and secure. This will involve creating metadata management systems and data lineage tracking tools to maintain an accurate record of data movements and transformations.
Furthermore, compliance with regulations such as GDPR, HIPAA, and others will be a key responsibility, as failure to adhere to these laws can result in costly penalties. Data engineers will need to stay informed of regulatory changes and adapt their processes to ensure that their organizations remain compliant. Implementing robust data governance practices will help mitigate risks and ensure that organizations can confidently leverage their data while protecting privacy and meeting legal obligations.
The future of data engineering will also focus on democratizing data access, enabling business users to make data-driven decisions without needing technical expertise. Data engineers will need to design systems that allow non-technical users to query and analyze data through user-friendly interfaces or self-service analytics platforms. This trend, known as data democratization, empowers business stakeholders to access real-time data and generate insights on their own, accelerating decision-making processes.
Data engineers will need to ensure that data is accessible, clean, and structured in a way that supports self-service tools. They will also need to implement data catalogs and other tools to make data discovery easier for non-technical users. This shift will require data engineers to create systems that balance ease of access with data security and privacy concerns, ensuring that sensitive information is only available to those who need it. The role of the data engineer will evolve to include enabling data literacy across the organization.
As data engineering continues to evolve, the roles and specializations within the field will also change. Data engineers will need to become more adaptable, learning new tools, technologies, and methodologies to keep pace with industry changes. We may see the emergence of new specialized roles within data engineering, such as data infrastructure engineers, real-time data engineers, and AI/ML data engineers.
As organizations adopt more complex data architectures, data engineers will need to develop niche expertise in areas like edge computing, blockchain-based data management, and federated learning. The future of data engineering will likely require professionals to have diverse skills and be able to work across different domains. This will open up new career opportunities and paths for data engineers, who will need to stay agile and continuously develop their expertise to keep up with the evolving demands of the industry.
The data engineer career path offers vast opportunities for growth and specialization. With the increasing reliance on data across industries, data engineers are essential for building and maintaining robust data infrastructures that ensure data is processed, stored, and analyzed efficiently. From entry-level roles to leadership positions, professionals can continuously advance by enhancing their technical skills, gaining experience, and pursuing certifications.
As the demand for data-driven decision-making continues to rise, pursuing a career in data engineering is not only a rewarding choice but also one that promises exciting challenges and opportunities for innovation in the ever-evolving tech landscape.
Copy and paste below code to page Head section
A data engineer is responsible for designing, building, and maintaining the infrastructure for data generation, collection, and storage. They focus on creating pipelines that allow data to flow seamlessly from sources to analytical systems, ensuring that data is cleaned, processed, and accessible for analysis by data scientists and other stakeholders.
To become a data engineer, one needs a strong knowledge of programming languages like Python, Java, or Scala. Expertise in database management, ETL processes, data modeling, and big data technologies like Hadoop and Spark are also essential. Cloud platforms, SQL/NoSQL, and data pipeline tools are key components of a data engineer’s skill set.
The salary of a data engineer varies based on experience, location, and the company. Entry-level data engineers can earn around $70,000 to $90,000 annually, while senior engineers or specialists in big data can earn upwards of $150,000. In cities with high demand, salaries may be higher due to the competitive market.
Data engineers are responsible for developing and managing the data architecture, including creating data pipelines that automate data flow and ensure the data is ready for analysis. They work closely with data scientists and analysts, ensuring clean, structured, and accessible data for decision-making, predictive modeling, and reporting.
To start a career in data engineering, pursuing a degree in computer science, information technology, or a related field is helpful. Gaining proficiency in programming, database management, and tools like SQL, Hadoop, and Spark is crucial. Additionally, gaining hands-on experience through internships or projects can significantly boost your skills and employability.
Data engineers focus on building the infrastructure and pipelines needed to collect, store, and manage data, while data scientists analyze this data to derive insights and build models. Data engineers ensure that data is clean, accessible, and ready for analysis, whereas data scientists use statistical techniques and machine learning algorithms to make predictions from the data.