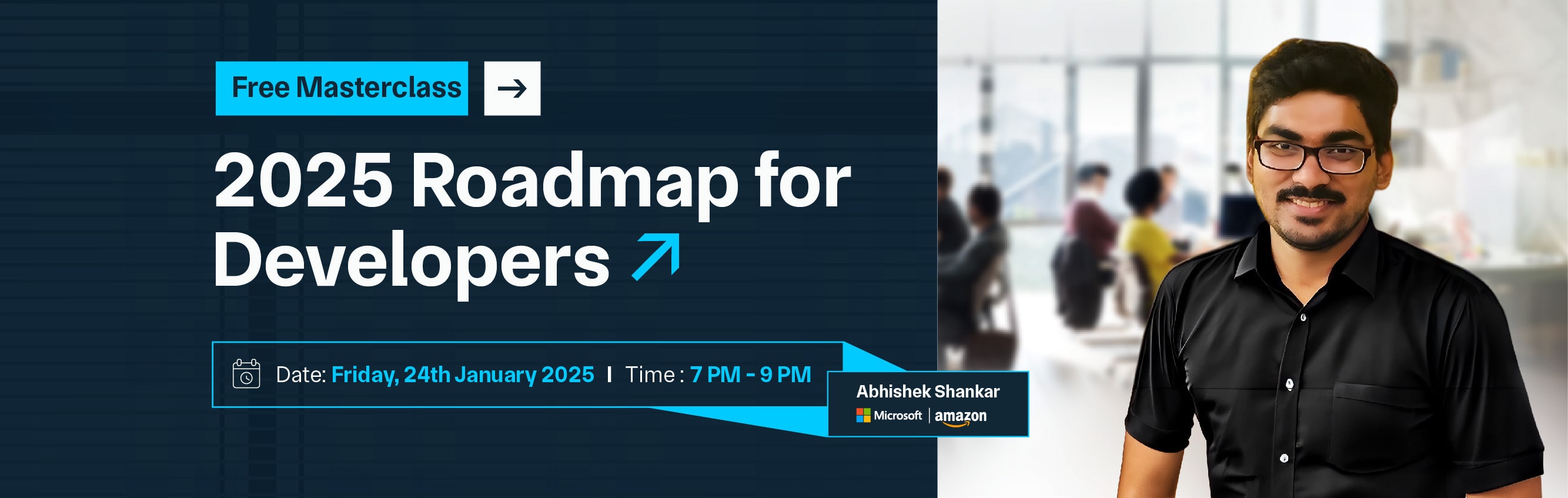

Big Data refers to the vast volume of structured and unstructured data generated daily from various sources, such as social media, sensors, and transactions. As organizations increasingly rely on data to drive decision-making and gain insights, understanding the main components of Big Data becomes essential. These components encompass the technologies, processes, and frameworks that enable the collection, storage, processing, and analysis of massive datasets. The primary components of Big Data include data sources, data storage, data processing, and data analytics.
Data sources can range from user-generated content on social media platforms to sensor data collected from IoT devices. Each of these sources contributes to the ever-growing volume of data, necessitating robust storage solutions capable of accommodating vast amounts of information. Technologies such as distributed file systems and cloud storage play a crucial role in managing these data repositories.
Moreover, the processing of Big Data involves complex algorithms and frameworks that facilitate the analysis of massive datasets. Tools like Apache Hadoop and Apache Spark are instrumental in processing data quickly and efficiently. Finally, data analytics transforms raw data into actionable insights using advanced statistical methods and machine learning techniques. By comprehending these components, organizations can leverage Big Data to drive innovation, improve operational efficiency, and enhance customer experiences.
Big Data refers to the massive volume of data generated from various sources, including social media interactions, online transactions, sensor data, and more. This data is characterized by its high volume, velocity, and variety, often referred to as the "three Vs." Volume refers to the sheer amount of data produced daily, while velocity denotes the speed at which this data is generated and processed. Variety highlights the diverse formats of data, ranging from structured data in databases to unstructured data like text, images, and videos.
Big Data has transformed how organizations operate, enabling them to gather insights and make data-driven decisions. The importance of Big Data lies in its potential to reveal patterns, trends, and correlations that were previously hidden. By leveraging advanced analytics and machine learning algorithms, businesses can extract valuable insights from large datasets, improving decision-making processes, enhancing customer experiences, and driving innovation.
Industries such as healthcare, finance, and retail are increasingly harnessing Big Data to predict customer behavior, optimize operations, and develop personalized services. As technology continues to evolve, the capacity to store, process, and analyze Big Data will only enhance, opening new avenues for research, development, and competitive advantage across various sectors.
Understanding the components of Big Data is essential for organizations aiming to leverage this resource effectively. Big Data consists of various elements that work together to collect, store, process, and analyze massive datasets. Each component plays a crucial role in transforming raw data into meaningful insights, driving informed decision-making and strategic planning.
This structured approach to managing Big Data can enhance operational efficiency, improve customer experiences, and foster innovation. With the exponential growth of data generated from various sources, organizations must grasp these components to implement effective Big Data strategies.
By doing so, they can ensure that they have the necessary tools and processes in place to capture valuable insights that drive business success. Understanding how these components interact and contribute to the overall Big Data ecosystem is vital for organizations looking to harness its full potential.
Data sources are the initial stage in the Big Data ecosystem, representing the diverse origins from which data is collected. These sources can be categorized into structured data, which is highly organized and easily searchable; unstructured data, which lacks a predefined format; and semi-structured data, which falls somewhere in between. Structured data often comes from traditional databases, while unstructured data can be generated from social media posts, emails, and multimedia content. Semi-structured data might include XML files and JSON documents.
The variety of data sources available to organizations allows them to gather a wealth of information that can be analyzed for insights. For example, social media platforms provide valuable data on customer sentiment and preferences, while Internet of Things (IoT) devices collect real-time data about processes and conditions. Understanding and integrating these various data sources enable businesses to create a comprehensive view of their operations and customer interactions, ultimately enhancing decision-making and driving innovation.
Once data is collected from various sources, the next critical component is data storage. Traditional databases often need help to handle the sheer volume and variety of Big Data, necessitating the use of more advanced storage solutions like distributed file systems and cloud storage. Technologies such as Hadoop Distributed File System (HDFS) facilitate efficient storage of large datasets across multiple nodes, ensuring scalability and fault tolerance.
Cloud storage options provide organizations with flexibility and accessibility, allowing them to store and retrieve data as needed without the burden of maintaining extensive hardware infrastructure. Effective data storage solutions not only accommodate large amounts of data but also ensure that it remains secure and easily accessible for processing and analysis. As data continues to grow exponentially, selecting the right storage solution becomes increasingly crucial for maintaining data integrity and supporting analytical efforts.
Data processing refers to the methods and techniques used to transform raw data into a format suitable for analysis. This stage is vital because it involves cleaning, transforming, and aggregating data from various sources to ensure that it is accurate, consistent, and relevant. Frameworks like Apache Hadoop and Apache Spark play a significant role in processing Big Data efficiently. Hadoop's MapReduce programming model allows for parallel processing of large datasets, while Spark offers in-memory processing capabilities for faster computation.
In addition to batch processing, real-time data processing is essential for applications that require immediate insights, such as fraud detection and online recommendation systems. Effective data processing ensures that organizations can derive valuable insights quickly, allowing them to make data-driven decisions in a timely manner. By investing in robust data processing solutions, organizations can enhance their analytics capabilities and stay ahead in today's competitive landscape.
Data analytics is the final component of the Big Data framework, focusing on extracting meaningful insights from processed data. This component encompasses various techniques, including statistical analysis, machine learning, and data visualization. Advanced analytics allows organizations to uncover patterns, correlations, and trends that can inform strategic decisions. For instance, predictive analytics can help businesses forecast customer behavior, while prescriptive analytics can recommend actions based on data insights.
Data visualization tools, such as Tableau or Power BI, facilitate the presentation of complex data in an understandable format, enabling stakeholders to grasp insights quickly. By leveraging data analytics, organizations can enhance operational efficiency, improve customer experiences, and drive innovation, ultimately leading to a competitive advantage in their respective industries. The ability to analyze and interpret data effectively is crucial for success in today's data-driven world.
Data governance involves the management of data availability, usability, integrity, and security within an organization. It establishes policies, procedures, and standards for data management, ensuring that data is accurate, consistent, and trustworthy. A robust data governance framework provides clear guidelines on data access, compliance, and ownership, helping organizations mitigate risks related to data mismanagement.
By implementing strong data governance practices, organizations can better protect sensitive information and maintain regulatory compliance, particularly in industries like finance and healthcare. Ensuring data quality and security fosters a data-driven culture that encourages informed decision-making and enhances overall operational efficiency. Organizations with effective data governance can also facilitate better collaboration among teams and improve the reliability of their analytics efforts.
With the increasing volume of data generated and stored, data security has become a paramount concern for organizations. This component focuses on protecting data from unauthorized access, breaches, and various cyber threats. Effective data security strategies involve implementing measures such as encryption, access controls, and regular audits to ensure sensitive information is adequately safeguarded.
Organizations must also comply with data privacy regulations, such as the General Data Protection Regulation (GDPR) or the Health Insurance Portability and Accountability Act (HIPAA), which impose strict guidelines on data handling and protection. By prioritizing data security, organizations can build trust with their customers, safeguard their reputations, and minimize the risk of financial losses due to data breaches or compliance failures. A proactive approach to data security not only protects assets but also enhances the overall resilience of the organization in the face of growing cyber threats.
Data integration is the process of combining data from various sources to create a unified view of information. This component is critical in Big Data environments, where data is often fragmented across multiple systems and formats. Effective data integration enables organizations to merge disparate data sources, ensuring that comprehensive and coherent datasets are available for analysis. Techniques such as Extract, Transform, Load (ETL), and data warehousing play essential roles in facilitating data integration.
By effectively integrating data, organizations can enhance their analytics capabilities and derive valuable insights that support strategic decision-making. For example, integrating customer data from different channels allows businesses to create a more complete profile of customer preferences and behaviors. This holistic view enables organizations to tailor their products and services more effectively, leading to improved customer satisfaction and loyalty. Ultimately, data integration is crucial for maximizing the value of Big Data and driving business growth.
Data visualization is a crucial component that focuses on representing data visually to enhance understanding and insight. Effective visualization techniques transform complex datasets into intuitive graphical formats, such as charts, graphs, and dashboards. This allows stakeholders to quickly identify patterns, trends, and anomalies within the data, facilitating more informed decision-making.
Visualization tools, like Tableau and Microsoft Power BI, empower organizations to create interactive and dynamic representations of data, enabling real-time analysis and collaboration. By leveraging data visualization, organizations can communicate insights more effectively, making it easier for decision-makers to grasp complex information and act on it promptly. A well-designed data visualization can significantly improve comprehension and retention of insights, ultimately driving better business outcomes and fostering a data-driven culture within the organization.
As organizations continue to navigate the ever-evolving landscape of Big Data, several trends are emerging that promise to shape its future. The growing volume and complexity of data require advanced techniques and technologies to harness its potential effectively. Emerging trends in Big Data are not only enhancing data analytics capabilities but also addressing challenges related to data privacy and security.
Organizations that adapt to these trends will be better positioned to derive meaningful insights, drive innovation, and maintain a competitive edge in their industries. Understanding these future trends will enable businesses to make informed decisions about their data strategies, ensuring they remain relevant and successful in an increasingly data-driven world.
From artificial intelligence and machine learning to real-time analytics and enhanced data governance, the evolution of Big Data presents both opportunities and challenges for organizations. As we explore these trends, we will uncover how they are expected to influence the way businesses operate and leverage data moving forward.
Big Data architecture plays a crucial role in how organizations manage and analyze large volumes of data effectively. With the rapid growth of data generation, having a robust architecture enables businesses to harness the power of Big Data to drive decision-making and innovation.
By establishing a structured framework, organizations can optimize data storage, improve accessibility, and enhance data processing capabilities. Furthermore, an effective Big Data architecture supports scalability, allowing businesses to adapt to evolving data requirements without significant infrastructure changes. Additionally, it promotes data integration from various sources, enabling organizations to gain comprehensive insights and foster data-driven cultures.
The benefits of implementing a solid Big Data architecture extend beyond operational efficiencies; they also include improved customer experiences and better strategic planning. As organizations increasingly rely on data analytics for competitive advantage, investing in a robust Big Data architecture becomes essential for sustainable growth.
Implementing an effective Big Data architecture is essential for organizations seeking to leverage the power of data analytics. However, several challenges can arise in this complex process, impacting data management, processing, and security. These challenges often stem from the sheer volume, velocity, and variety of data generated in today's digital landscape.
Organizations must navigate issues related to data integration, storage, processing, and governance while ensuring compliance with regulations and maintaining data security. Additionally, the rapid pace of technological advancements necessitates continuous updates and adaptations to existing architectures.
Addressing these challenges requires a strategic approach that includes selecting the right tools, technologies, and frameworks to support Big Data initiatives. Organizations that can effectively overcome these challenges will position themselves to unlock the full potential of their data, leading to improved decision-making, enhanced operational efficiency, and competitive advantage.
Big Data has transformed various industries by enabling organizations to gather, process, and analyze vast amounts of data to derive actionable insights. These insights drive innovation, improve operational efficiency, and enhance decision-making processes. The applications of Big Data are extensive and span numerous sectors, including healthcare, finance, retail, and transportation.
By leveraging advanced analytics, businesses can better understand customer behaviors, predict market trends, and optimize resource allocation. In this section, we will explore several compelling examples of how Big Data is utilized across different industries.
These examples illustrate the diverse applications and the potential of Big Data to revolutionize traditional practices and improve overall outcomes. From personalized marketing strategies to predictive maintenance, the impact of Big Data is profound, making it an essential component of modern business strategies.
In the healthcare sector, Big Data analytics has revolutionized patient care and outcomes. By harnessing the power of vast patient datasets, healthcare providers can identify trends, track disease outbreaks, and improve treatment protocols. Predictive analytics allows hospitals to forecast patient admissions based on historical data, facilitating better resource allocation and staffing decisions. Additionally, the integration of wearable devices collects real-time health data, enabling continuous monitoring of patients.
This technology allows healthcare providers to implement personalized care plans tailored to individual needs. Overall, this data-driven approach enhances patient care quality, reduces operational costs, and improves health outcomes. Furthermore, by analyzing patterns in treatment effectiveness, healthcare organizations can refine their practices, leading to better patient satisfaction and efficiency in service delivery. This holistic approach to health management is increasingly vital in today's data-centric healthcare landscape.
In the financial services industry, Big Data is instrumental in enhancing risk management, fraud detection, and customer personalization. Banks and financial institutions utilize advanced analytics to scrutinize transaction data, identifying unusual patterns that may indicate fraudulent activity. Machine learning algorithms are employed to predict and mitigate potential risks before they escalate, improving the safety and security of financial transactions. Moreover, personalized marketing strategies are developed based on comprehensive customer data analysis.
This allows institutions to tailor products and services to meet individual customer needs effectively. As a result, financial organizations can enhance customer satisfaction and loyalty while optimizing their operational efficiency. The ability to analyze vast amounts of data in real time empowers financial institutions to make informed decisions, thus improving overall profitability. By leveraging Big Data, these organizations are not only better equipped to manage risks but also to seize new opportunities in a competitive marketplace.
In the retail and e-commerce sectors, Big Data plays a crucial role in understanding consumer behavior and optimizing inventory management. Retailers analyze extensive datasets that include purchase histories, online browsing patterns, and demographic information to create personalized shopping experiences. This data-driven approach helps businesses identify trends and make informed decisions regarding product offerings, pricing strategies, and marketing campaigns. For example, by leveraging predictive analytics, retailers can anticipate demand for certain products and adjust inventory levels accordingly.
This ensures that popular items are readily available while minimizing excess inventory costs. Additionally, retailers use customer data to tailor promotions and discounts to specific segments, enhancing customer engagement and driving sales. Ultimately, the integration of Big Data in retail leads to improved customer satisfaction and loyalty, higher sales conversion rates, and a more efficient supply chain. This data-centric strategy is essential for staying competitive in a rapidly evolving market landscape.
In the transportation and logistics industry, Big Data analytics is utilized to enhance route optimization, improve supply chain management, and increase operational efficiency. Companies analyze a plethora of data, including traffic patterns, weather conditions, and historical delivery records, to determine the most efficient routes for their vehicles. This approach not only reduces fuel consumption and delivery times but also minimizes carbon emissions, contributing to sustainability efforts. Predictive analytics further allows companies to anticipate maintenance needs for vehicles and equipment.
This reduces downtime and improves service reliability. By leveraging real-time data, organizations can adapt quickly to changing circumstances, such as traffic disruptions or adverse weather, ensuring timely deliveries. Furthermore, advanced analytics can optimize inventory management and demand forecasting, leading to more responsive supply chains. This data-driven approach ultimately results in cost savings, improved customer satisfaction, and a more agile logistics network capable of meeting modern demands.
Big Data is a pivotal component in the development of smart cities, where data-driven technologies enhance urban living and governance. Cities collect and analyze data from various sources, including sensors, cameras, and public transportation systems, to optimize city services and improve the quality of life for residents. For instance, real-time traffic data can be utilized to manage traffic lights efficiently, reducing congestion and improving commute times. Smart waste management systems equipped with sensors optimize waste collection schedules by monitoring bin levels.
This leads to cost savings and improved efficiency. Additionally, public safety can be enhanced through the analysis of crime patterns, allowing law enforcement agencies to allocate resources more effectively. By leveraging Big Data, cities can implement sustainable practices, improve energy management, and enhance public services. The integration of technology and data analysis enables smart cities to become more livable, efficient, and responsive to the needs of their residents, fostering a better urban environment.
In the age of digital communication, social media platforms generate vast amounts of data daily, providing valuable insights into consumer preferences, trends, and behavior. Businesses analyze this data to gauge brand sentiment, identify market trends, and engage with customers effectively. For example, companies can track mentions of their products or services across various social media channels to understand public perception and sentiment. This real-time feedback allows brands to respond to customer inquiries, complaints, or praise promptly.
Additionally, businesses use social media analytics to identify potential influencers and key opinion leaders within their target demographics. By understanding the dynamics of social media conversations, companies can refine their marketing strategies and optimize content for maximum impact. This data-driven approach not only enhances customer engagement but also strengthens brand loyalty and market positioning. Ultimately, social media analytics plays a crucial role in shaping modern marketing strategies and fostering deeper connections with audiences.
In the realm of sports, Big Data is transforming performance analysis, player scouting, and fan engagement. Teams collect and analyze data on player performance, game statistics, and even fan behavior to make informed decisions. For instance, analyzing player movements during games provides coaches with insights into performance strengths and weaknesses, allowing for the development of tailored training programs. Additionally, teams utilize data analytics to enhance fan experiences by offering personalized content, promotions, and engagement opportunities based on fan preferences.
This data-centric approach not only drives ticket sales but also fosters loyalty among supporters. Moreover, sports organizations analyze data to optimize strategies for player recruitment, assessing potential signings based on performance metrics. The integration of Big Data into sports enables teams to make strategic decisions that enhance competitiveness while providing fans with a more immersive experience. As the industry evolves, the reliance on data-driven insights continues to shape the future of sports.
Big Data analytics is significantly transforming the manufacturing industry by enhancing production processes, improving efficiency, and minimizing downtime. Manufacturers collect extensive data from machinery, supply chains, and quality control systems to optimize operations. By employing predictive maintenance techniques, companies can anticipate equipment failures before they occur, reducing costly downtimes and unplanned maintenance. This proactive approach allows manufacturers to schedule maintenance during non-peak hours, ensuring that production remains uninterrupted.
Additionally, real-time monitoring of production lines helps identify bottlenecks and inefficiencies, enabling manufacturers to streamline operations and boost productivity. By analyzing data trends, organizations can make informed decisions about resource allocation, workforce management, and production schedules. Ultimately, the integration of Big Data into manufacturing processes not only enhances operational efficiency but also fosters innovation, competitiveness, and responsiveness in a dynamic market environment. This data-driven strategy is essential for manufacturers aiming to succeed in today’s fast-paced industrial landscape.
Big Data is characterized by five critical dimensions commonly known as the "Vs": Volume, Velocity, Variety, Veracity, and Value. These dimensions not only highlight the unique challenges organizations face but also provide a framework for understanding how to leverage Big Data effectively.
In an era where data generation is exponential, organizations must grasp these dimensions to navigate the complexities of data management and analytics. Recognizing the Vs of Big Data enables companies to unlock valuable insights that drive innovation, enhance decision-making, and improve overall business performance.
Each dimension offers distinct characteristics that influence data storage, processing, and analysis strategies. As we delve into each of these dimensions, it becomes clear that understanding them is essential for businesses looking to gain a competitive edge in today’s data-driven landscape.
Volume refers to the sheer amount of data generated daily from diverse sources such as social media, sensors, transactions, and more. Organizations are now dealing with terabytes to petabytes of data, which presents significant challenges in terms of storage, processing, and analysis. Managing such vast quantities of data requires advanced storage solutions and scalable architectures capable of handling the increased demand. Moreover, the rising volume of data necessitates the use of distributed computing technologies, such as cloud storage and big data frameworks like Hadoop and Apache Spark.
These technologies allow organizations to process and analyze data in parallel across multiple nodes, improving efficiency and speed. Companies must also consider data retention policies and regulatory compliance, ensuring that they can manage and store large volumes of data responsibly. Overall, the volume of data emphasizes the need for robust data management strategies to unlock valuable insights.
Velocity pertains to the speed at which data is generated, processed, and analyzed. In today’s fast-paced digital environment, data is continuously flowing in real time from various sources, including IoT devices, social media platforms, and transaction systems. Organizations must respond quickly to this data influx to gain timely insights and maintain a competitive edge. To manage the high velocity of data, businesses employ real-time data processing technologies and streaming analytics.
This enables organizations to capture and analyze data as it arrives, allowing for immediate decision-making. For instance, in finance, real-time data analysis can help detect fraudulent transactions and mitigate risks swiftly. However, processing high-velocity data also poses challenges, such as ensuring data quality and accuracy. Organizations need to develop strategies for managing streaming data efficiently, ensuring that they can derive insights promptly without sacrificing data integrity.
Variety refers to the diverse types and formats of data generated from various sources, including structured, semi-structured, and unstructured data. Structured data is organized and easily searchable, such as data stored in databases. In contrast, unstructured data includes text, images, videos, and other formats that do not have a predefined structure, making it more challenging to analyze. The growing variety of data presents opportunities for organizations to gain deeper insights by integrating multiple data types.
For example, combining social media sentiment analysis with traditional sales data can provide a comprehensive view of consumer behavior and preferences. However, managing this variety requires advanced analytics tools and techniques capable of processing different data types effectively. Businesses need to invest in data integration solutions that can aggregate and harmonize diverse datasets, enabling them to extract meaningful insights from a wide range of information.
Veracity refers to the quality, accuracy, and trustworthiness of data. With the vast amounts of data being generated, ensuring its integrity becomes critical. Poor quality data can lead to inaccurate insights and flawed decision-making, undermining the benefits of Big Data initiatives. Organizations must implement rigorous data validation and cleansing processes to ensure that the information they rely on is accurate and reliable.
Data integrity also encompasses the need to evaluate the sources of data. Trustworthy sources contribute to higher data quality, while unreliable or biased sources can compromise the integrity of insights. Businesses must develop strategies to assess data quality continually and implement mechanisms for data governance. By prioritizing data integrity, organizations can enhance their decision-making processes, build trust in their data-driven initiatives, and ultimately improve business outcomes.
Value refers to the actionable insights and benefits derived from analyzing Big Data. More than simply simply having access to large volumes of data is required; organizations must effectively analyze and interpret this data to generate meaningful insights that drive strategic decisions. The true value of Big Data lies in its ability to inform and guide business strategies, optimize operations, and enhance customer experiences.
To unlock the value of Big Data, organizations must invest in advanced analytics tools and skilled data scientists capable of deriving insights from complex datasets. Moreover, fostering a data-driven culture within the organization is crucial for ensuring that insights are integrated into everyday decision-making processes. By focusing on the value dimension, businesses can leverage Big Data to enhance efficiency, drive innovation, and create a competitive advantage in their respective markets.
Big Data works by leveraging various technologies, tools, and methodologies to process and analyze vast amounts of data generated from numerous sources. The objective is to extract valuable insights that can drive decision-making and strategic planning for businesses. It involves a cycle that includes data collection, storage, processing, analysis, and visualization. Each of these steps plays a critical role in transforming raw data into actionable information.
As organizations increasingly rely on data-driven strategies, understanding how Big Data works becomes essential for harnessing its potential. The process starts with data collection, which can come from various sources like social media, IoT devices, transactions, and more. Once collected, the data is stored in systems designed to handle large volumes, such as cloud storage or distributed databases.
Following storage, the data undergoes processing, which prepares it for analysis. This step may involve cleaning, filtering, and transforming data into a usable format. Finally, advanced analytics and visualization techniques help in interpreting the data, providing insights that can influence business strategies.
Data-driven businesses are achieving remarkable success by leveraging analytics and insights to make informed decisions. These organizations harness the power of data to enhance their operational efficiency, improve customer experiences, and drive innovation.
By analyzing market trends, consumer behaviors, and internal processes, data-driven companies can develop strategies that align with their goals and adapt to changing environments.
As a result, they often outperform their competitors, achieve higher profitability, and foster a culture of continuous improvement. The shift towards data-driven decision-making not only enhances performance but also positions businesses to thrive in an increasingly competitive landscape.
In today's data-driven landscape, effective big data strategies are essential for organizations seeking to leverage vast amounts of information for competitive advantage. These strategies encompass various methodologies, tools, and practices designed to collect, store, process, and analyze data.
By implementing robust solutions tailored to their unique needs, businesses can unlock insights that drive innovation, enhance decision-making, and improve operational efficiency. The integration of advanced technologies, such as machine learning and artificial intelligence, further empowers organizations to transform raw data into actionable intelligence.
Ultimately, a well-defined big data strategy enables organizations to adapt to market changes, optimize customer experiences, and position themselves as leaders in their respective industries.
Lambda architecture is a data-processing architecture designed to handle massive quantities of data by leveraging both batch processing and real-time streaming. It consists of three layers: the batch layer, which stores the master dataset and pre-computes batch views; the speed layer, which processes real-time data streams to provide low-latency updates; and the serving layer, which combines outputs from both the batch and speed layers to serve requests.
This hybrid approach enables organizations to benefit from the accuracy and completeness of batch processing while maintaining the responsiveness and timeliness of real-time analytics. One of the key advantages of Lambda architecture is its fault tolerance and scalability, which allows it to accommodate varying data loads and processing requirements.
However, it can be complex to implement, as it requires maintaining two separate processing systems and ensuring that they work seamlessly together. Additionally, managing the dual systems can lead to increased operational overhead. Despite these challenges, Lambda architecture remains popular for applications requiring both historical and real-time data processing, such as in e-commerce, fraud detection, and social media analytics.
Kappa architecture is an alternative to Lambda architecture that simplifies the data processing pipeline by eliminating the batch processing layer. Instead, it focuses solely on real-time stream processing, treating all data as a continuous stream. In Kappa architecture, all data flows through a single processing layer, allowing organizations to perform real-time analytics on streaming data while storing the data in a distributed log system.
This streamlined approach reduces complexity by eliminating the need for batch jobs, resulting in a more straightforward architecture that is easier to maintain and scale. One of the significant benefits of Kappa architecture is its ability to handle data changes efficiently, as it allows organizations to reprocess historical data simply by replaying the data from the distributed log.
This capability ensures that all data is processed consistently and that the system can quickly adapt to new requirements or changes in business logic. However, Kappa architecture may only be suitable for some use cases, particularly those that require extensive historical data analysis or complex batch computations. It is particularly well-suited for applications focused on real-time analytics, such as monitoring systems, recommendation engines, and IoT applications.
Understanding the main components of big data is essential for organizations aiming to leverage vast amounts of information effectively. Each component, including volume, velocity, variety, integrity, and value, plays a critical role in how data is collected, processed, and analyzed. By comprehensively grasping these elements, businesses can develop robust data strategies that enable them to extract actionable insights and drive innovation. As technology continues to evolve, so will the methods and tools used to manage big data, making it crucial for organizations to stay informed and adaptable in their approaches.
Moreover, successfully integrating these components can lead to enhanced decision-making and improved operational efficiency. By prioritizing data governance and implementing the right infrastructure, organizations can ensure data quality and reliability, which are vital for achieving meaningful results. Ultimately, embracing the complexities of big data and its components will empower businesses to navigate an increasingly data-driven world and gain a competitive edge in their respective industries.
Copy and paste below code to page Head section
The main components of big data include volume, velocity, variety, integrity, and value. Volume refers to the large amounts of data generated; velocity pertains to the speed at which data is processed; variety indicates the different formats and types of data. Integrity relates to the accuracy and trustworthiness of the data. And the value represents the insights and benefits derived from data analysis.
Volume significantly impacts big data processing as it determines the storage capacity and processing power required. Large datasets necessitate robust infrastructure and scalable solutions to handle the influx of data efficiently. With adequate resources, organizations may be able to analyze and derive meaningful insights from their data, potentially leading to missed opportunities.
Velocity is crucial in big data because it refers to the speed at which data is generated and needs to be processed. In today’s fast-paced environment, organizations require real-time or near-real-time analytics to respond quickly to market changes, customer behavior, and operational issues. High velocity enables timely decision-making and enhances competitiveness.
Data variety pertains to the diverse formats and types of data generated from various sources, including structured, semi-structured, and unstructured data. Understanding data variety is essential because it influences how data is collected, stored, and analyzed. Organizations must have the tools and strategies in place to handle different data types effectively to gain comprehensive insights.
Veracity refers to the accuracy and reliability of data. High integrity ensures that the insights derived from data analysis are trustworthy and valid. If data quality is maintained, organizations may make better decisions based on accurate information. Ensuring high veracity involves implementing data governance and cleansing processes to maintain data integrity.
The value of big data lies in the actionable insights it provides, which can drive business growth, improve operational efficiency, and enhance customer experiences. By analyzing large datasets, organizations can identify trends, optimize processes, and make data-driven decisions that lead to a competitive advantage. Ultimately, the value is realized through effective data utilization and strategic implementation.