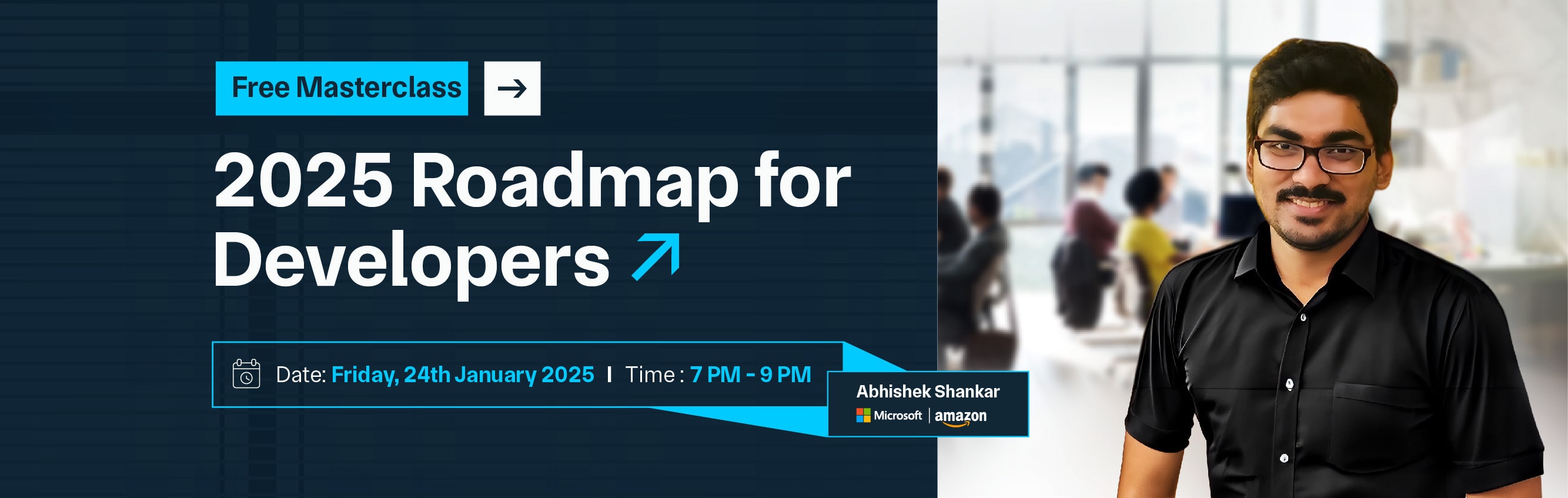

Business Intelligence (BI) and Big Data are critical elements of modern data-driven decision-making, yet they serve distinct purposes in the analytics ecosystem. BI focuses on analyzing structured data to provide actionable insights for businesses. It emphasizes the historical and current performance of an organization, utilizing tools like dashboards, reports, and visualizations to support strategic planning. BI is tailored for business users, helping them make informed decisions based on well-organized data.
In contrast, Big Data encompasses the vast, complex, and unstructured datasets generated from various sources such as social media, sensors, and transactions. These datasets are characterized by their volume, variety, velocity, and veracity, making them challenging to process using traditional tools. Big Data employs advanced technologies like Hadoop, Spark, and machine learning to uncover patterns and trends that traditional BI tools might overlook. It is often exploratory, enabling predictive and prescriptive analytics.
While BI is more about making sense of existing data to answer specific business questions, Big Data aims to harness massive datasets for discovering new opportunities and solving complex problems. Together, they complement each other, as Big Data can fuel BI systems with deeper insights, and BI frameworks provide a foundation for understanding and visualizing Big Data outputs in a meaningful way.
Business Intelligence (BI) refers to the processes, technologies, and tools used to analyze and transform raw data into meaningful insights for business decision-making. BI systems focus on structured data, often gathered from internal databases, spreadsheets, and reporting systems, to provide a clear view of an organization’s performance. By utilizing dashboards, reports, and data visualization tools, BI enables companies to identify trends, monitor key performance indicators (KPIs), and support strategic planning.
It emphasizes past and current data analysis, offering actionable insights to improve efficiency and profitability. The goal of BI is to empower business users with real-time, accessible information that simplifies complex data into actionable formats. Modern BI systems integrate with various data sources, ensuring seamless and accurate reporting. While traditional BI was limited to descriptive analytics, contemporary tools also incorporate predictive capabilities, helping organizations anticipate market trends and adapt to dynamic business environments.
Big Data refers to massive volumes of data generated at high speed from diverse sources, including social media platforms, IoT devices, sensors, and transactional systems. Unlike traditional data, Big Data is characterized by its three Vs: Volume, Variety, and Velocity. Handling such data requires advanced tools and frameworks like Apache Hadoop, Apache Spark, and NoSQL databases that can process, store, and analyze these datasets efficiently.
Big Data goes beyond structured information, incorporating unstructured and semi-structured data like images, videos, and text. It is used to uncover patterns, correlations, and trends that were previously difficult to analyze due to the limitations of traditional tools. This data drives advanced analytics, such as machine learning and artificial intelligence, enabling predictive and prescriptive insights. Organizations leverage Big Data to enhance customer experiences, optimize operations, and create innovative solutions, ultimately gaining a competitive edge in the marketplace.
Business Intelligence (BI) is an indispensable tool for organizations seeking to leverage data for strategic decision-making. It transforms raw data into actionable insights, providing businesses with the ability to monitor key metrics, anticipate trends, and enhance operational efficiency. BI tools, such as dashboards and predictive analytics, enable data visualization and real-time reporting, allowing organizations to remain agile in dynamic markets.
Despite its numerous benefits, implementing BI comes with its challenges. The setup can be resource-intensive, requiring significant investment and expertise. Additionally, the reliability of BI insights hinges on the accuracy and completeness of the data being analyzed. Without proper integration, maintenance, and data governance, businesses may face issues such as inefficiency and security risks.
Big Data has revolutionized the way organizations analyze, process, and utilize data. With its ability to handle massive volumes of structured, semi-structured, and unstructured data, Big Data allows businesses to gain deeper insights into customer behavior, market trends, and operational efficiencies. By leveraging tools like Hadoop and Spark, companies can perform predictive and prescriptive analytics to make proactive decisions and create innovative solutions.
However, managing Big Data comes with its own set of challenges. Its sheer volume, variety, and velocity demand advanced technologies, skilled professionals, and significant financial investment. Additionally, the complexity of integrating and analyzing diverse datasets can lead to inefficiencies if not handled properly. Issues like data security and privacy also pose significant concerns as organizations deal with sensitive information.
Big Data and Business Intelligence (BI) are powerful data-centric approaches, but they serve distinct purposes in the analytics ecosystem. Big Data focuses on collecting, processing, and analyzing massive, diverse datasets from multiple sources, including unstructured and semi-structured data. It leverages advanced technologies like machine learning and distributed computing to identify patterns and predict future trends, offering exploratory insights that drive innovation and strategic planning.
On the other hand, Business Intelligence concentrates on structured data analysis to provide actionable insights for immediate decision-making. BI systems utilize tools such as dashboards and reports to present historical and current performance metrics, helping businesses make data-driven decisions efficiently. Unlike Big Data, which emphasizes discovering new trends, BI focuses on summarizing data for operational and strategic clarity.
Business Intelligence (BI) and Big Data are two significant approaches in data analysis, each playing a unique role in driving business decisions. BI focuses on analyzing historical and current structured data to provide actionable insights for immediate decision-making. It uses tools like dashboards and reports to optimize operations and track performance effectively. In contrast, Big Data deals with massive and complex datasets from diverse sources, including unstructured and semi-structured data.
It uses advanced technologies to identify trends, predict future outcomes, and explore innovative opportunities. Big Data emphasizes scalability and predictive analytics to uncover hidden patterns in large-scale data environments. While both aim to empower organizations with data-driven insights, their methodologies, tools, and objectives differ. Below is a detailed explanation of their key differences:
Business Intelligence (BI) focuses primarily on providing actionable insights by analyzing structured, historical, and current data. It helps organizations track performance, measure efficiency, and monitor trends through dashboards, reports, and visualizations. This structured approach allows businesses to quickly assess their operations and make data-driven decisions for immediate outcomes. BI's main goal is to support decision-making by providing clear, consistent insights into the organization's performance, such as sales reports, financial metrics, and customer behavior analytics. The insights offered by BI are typically retrospective, designed to optimize ongoing operations or solve specific problems faced by the business at the moment.
In contrast, Big Data focuses on analyzing massive, complex datasets, including unstructured and semi-structured data, from multiple sources. This could include data from social media, IoT devices, logs, and transactional data, all of which contribute to uncovering hidden trends and patterns that can drive future business decisions. Big Data isn't only about historical performance; it's about forecasting and predicting trends based on vast, diverse datasets. Its focus lies in innovation and forward-looking strategies, enabling organizations to discover opportunities and make decisions that shape their long-term success. The scalability of Big Data allows businesses to gain insights from diverse, large-scale datasets, unlocking value from previously untapped sources of information.
BI works predominantly with structured data that is neatly organized into tables and columns within traditional databases and data warehouses. This type of data is highly organized, making it easy to analyze and visualize using BI tools. Structured data includes numerical data, customer information, transaction records, and other datasets that follow a consistent and predefined format. The clarity of structured data allows BI tools to create concise reports, dashboards, and visualizations, enabling decision-makers to monitor business health and track key performance indicators (KPIs). BI tools excel when working with this type of data, delivering clear and accurate insights.
Big Data, however, is designed to handle a much broader range of data types. It processes structured, semi-structured, and unstructured data, which could include text files, images, videos, social media content, and sensor data. Big Data tools are capable of parsing and analyzing diverse datasets from different sources. By integrating and processing unstructured data, Big Data can generate more comprehensive insights, such as predicting trends or uncovering patterns from social interactions, customer feedback, or machine-generated data. This flexibility allows Big Data to operate in dynamic environments, addressing complex business challenges that require multifaceted data types for analysis.
The goal of Business Intelligence is to provide organizations with insights from structured data to improve decision-making, streamline operations, and boost performance. BI tools aim to monitor the current status of business operations, track performance metrics, and identify areas for improvement. By focusing on structured, historical data, BI helps businesses optimize existing processes, improve customer experiences, and support tactical decisions. For example, BI might help companies track sales trends, monitor inventory levels, or analyze employee performance. Its main goal is to enhance operational efficiency and inform day-to-day decision-making based on existing data.
On the other hand, Big Data’s goal extends beyond optimizing current processes to predicting future trends and uncovering hidden patterns. Big Data aims to provide organizations with the ability to anticipate market shifts, identify new business opportunities, and innovate in real time. With the ability to analyze unstructured data and vast datasets, Big Data supports strategic decisions that help businesses remain competitive in rapidly changing markets. By uncovering correlations and trends that were previously invisible, Big Data allows businesses to make forward-looking, data-driven decisions, often leading to transformative innovations. The goal of Big Data is to create new value from large-scale, complex datasets.
Business Intelligence tools primarily include platforms like Tableau, Power BI, QlikView, and traditional reporting tools that focus on data visualization, reporting, and analysis of structured datasets. These tools are designed to simplify the process of data extraction, cleaning, and reporting, making it accessible to business users without the need for deep technical expertise. BI tools provide dashboards, charts, and graphs to track business performance, KPIs, and trends in a user-friendly format. They are optimized for analyzing historical data and summarizing it into digestible insights. Additionally, BI tools allow easy access to historical reports, helping users identify trends and areas for improvement.
In contrast, Big Data tools are built for processing large volumes of diverse data types. Common tools used in Big Data environments include Apache Hadoop, Apache Spark, NoSQL databases, and machine learning algorithms. These tools allow businesses to process unstructured, semi-structured, and structured data on a large scale. Big Data platforms also incorporate advanced technologies like machine learning, predictive analytics, and artificial intelligence to analyze and predict trends. Tools such as MapReduce, Hadoop Distributed File System (HDFS), and machine learning models are essential for managing and analyzing massive datasets in real time. Big Data tools are specifically designed to handle the volume, velocity, and variety of data encountered in modern business environments.
Scalability in Business Intelligence is limited by the size and structure of the data being processed. Since BI mainly focuses on structured data, it generally operates within the confines of traditional databases and data warehouses. While BI tools can scale to some degree, they are optimized for smaller datasets or operational data that do not require extensive processing power. As businesses grow and accumulate more data, BI systems may require additional infrastructure. Still, they are usually designed to scale within known, predefined limits, offering performance at a moderate to large scale for structured data analysis.
Big Data, however, is specifically designed to scale efficiently to handle the vast amount of data generated by modern organizations. Big Data technologies, like Hadoop and Apache Spark, are built for distributed computing, allowing them to process petabytes of data across multiple servers. The scalability of Big Data platforms means they can handle not just large volumes of data but also the speed and variety that comes with it. This allows Big Data systems to scale horizontally, expanding resources as data grows. It makes them ideal for organizations dealing with massive datasets from various sources such as social media, IoT devices, and other large-scale data inputs.
Business Intelligence focuses on descriptive and diagnostic analytics. It examines historical data and identifies trends and patterns that inform decision-making. By analyzing structured data from various business functions like sales, finance, and HR, BI tools provide insights into what happened in the past and why. For instance, BI can help businesses understand why sales dropped in a specific quarter by comparing historical data against performance metrics. This analysis type is ideal for improving current processes and operations, providing insights into areas where the business is performing well or needs improvement.
Big Data, on the other hand, is more focused on predictive and prescriptive analytics. It leverages advanced algorithms, machine learning, and AI to forecast future trends, behaviors, and outcomes. Big Data analysis allows businesses to predict customer behavior, identify potential risks, and optimize operations before problems arise. By processing large datasets, Big Data helps organizations explore scenarios that were previously out of reach, identifying new opportunities and strategies. This type of analysis is crucial for businesses looking to innovate, anticipate market shifts, and create long-term value through data.
Business Intelligence tools are designed to deliver insights in real-time or near real-time by working with structured data that has already been processed and stored. Since BI focuses on analyzing pre-organized datasets, it typically provides quick access to reports, dashboards, and visualizations. These tools enable decision-makers to act swiftly based on current data, tracking performance metrics and operational KPIs. While BI can process data quickly, its speed is limited by the scale and structure of the data being analyzed. BI is best suited for businesses that need to analyze historical data and make timely decisions based on clear, predefined information.
Big Data, by contrast, is optimized for both real-time and batch processing of vast amounts of unstructured, semi-structured, and structured data. Big Data platforms like Hadoop and Apache Spark can process large datasets at high speeds, enabling businesses to analyze data as it is generated. For example, Big Data can analyze IoT sensor data or social media content in real-time, identifying emerging trends and anomalies. The processing speed of Big Data systems is essential for businesses that need to respond quickly to dynamic conditions and leverage predictive analytics to drive future strategies.
The complexity of Business Intelligence lies in its ability to work with structured data and derive meaningful insights using established methods. While BI tools are user-friendly and accessible to non-technical users, the process of transforming raw data into actionable insights requires considerable expertise in data visualization and interpretation. Users need to understand how to configure reports, design dashboards, and interpret metrics to create value. Additionally, while the complexity of BI tools is often manageable, it can be challenging for businesses to scale their BI systems without adding additional infrastructure or tools.
Big Data, on the other hand, is inherently more complex due to the sheer volume, variety, and velocity of data it processes. It requires specialized knowledge in data science, machine learning, and advanced analytics to exploit the value of the data fully. Big Data platforms are built for large-scale data processing, requiring skilled professionals to set up, maintain, and analyze vast datasets. The complexity also extends to data storage, integration, and security, as managing unstructured data from various sources requires careful attention to detail. Businesses leveraging Big Data often need to invest heavily in infrastructure, data management processes, and highly skilled personnel.
Business Intelligence is commonly used for operational tasks, such as performance monitoring, financial analysis, sales tracking, and reporting. It helps organizations track KPIs, monitor budgets, and evaluate product performance. For example, a retailer can use BI to monitor sales by region, identify high-performing products, and make data-driven decisions to optimize their inventory. BI is also used for analyzing customer behavior and generating periodic reports that help managers make tactical decisions. It is ideal for companies focused on improving efficiency and gaining real-time insights into their day-to-day operations.
Big Data, in contrast, is often used in use cases that require deeper analysis, predictive capabilities, and innovation. Industries such as healthcare, finance, manufacturing, and marketing use Big Data for fraud detection, predictive maintenance, customer segmentation, and demand forecasting. For example, e-commerce companies use Big Data to personalize customer experiences by analyzing browsing behavior, purchase history, and social media activity. Healthcare providers analyze patient data to predict disease outbreaks or optimize treatment plans. Big Data is critical for industries looking to innovate, predict future trends, and optimize large-scale processes.
Business Intelligence is widely used across industries, especially those with well-defined data structures and established reporting needs, such as retail, finance, and manufacturing. These industries benefit from BI's ability to monitor operational data, track performance, and analyze customer behavior. In retail, for example, BI tools can optimize inventory management by providing insights into sales trends helping companies make data-driven decisions. Similarly, financial institutions rely on BI to monitor transactions, track investments, and analyze customer accounts. BI is best suited for industries where decision-making is based on historical data and structured reporting.
Big Data, however, is more commonly found in industries that require real-time data analysis, complex datasets, and predictive modeling. Sectors such as technology, healthcare, telecommunications, and energy use Big Data to optimize operations, forecast demand, and improve services. For instance, telecom companies use Big Data to monitor network performance and detect anomalies, while healthcare providers analyze patient data to predict outcomes and optimize treatments. Big Data is crucial for industries with vast amounts of unstructured data and a need for advanced analytics to uncover patterns, predict trends, and foster innovation.
Business Intelligence (BI) and Big Data share several common characteristics that enable businesses to derive valuable insights from data. Both BI and Big Data aim to improve decision-making processes, drive business growth, and optimize operations by analyzing vast amounts of data. While they operate in different environments and handle varying data types, both BI and Big Data use advanced analytics to uncover patterns, trends, and opportunities. These insights help businesses make more informed, data-driven decisions that lead to better outcomes.
Additionally, both BI and Big Data emphasize the importance of data-driven culture in organizations, empowering teams across various departments to harness the potential of data for competitive advantage. They both rely on cutting-edge tools and technologies, fostering collaboration between business stakeholders and IT professionals to extract insights from complex datasets. Despite their differences in scale and complexity, BI and Big Data are complementary, providing a holistic approach to data analysis that drives business success.
Choosing between Business Intelligence (BI) and Big Data depends largely on the goals, size, and scope of your business needs. While both offer valuable insights and improve decision-making, they cater to different aspects of data analysis. BI is typically used by businesses that rely on structured, historical data to monitor and optimize current operations. In contrast, Big Data is essential for handling large volumes of unstructured data and is often used for more complex analytics, like predictive and real-time decision-making.
It is important to understand your business's specific needs and capabilities before selecting the right approach. If you're aiming for operational efficiency and reporting based on past data, BI might be the better option. However, if your goal is to handle large datasets, uncover trends, and predict future outcomes, Big Data may be the better choice. The decision ultimately boils down to the types of data you need to process and the level of complexity required to support your business strategy.
In today's digital era, data is being generated at an unprecedented rate. From social media interactions to IoT devices and e-commerce transactions, the world is producing vast amounts of data every second. Big Data refers to datasets that are so large, complex, and dynamic that traditional data processing methods are inadequate to handle them. This explosion of data has led businesses, governments, and organizations to look for advanced technologies and techniques to analyze, store, and derive insights from these vast datasets.
Big Data is not just about the volume of data but also the variety, velocity, and integrity of data, which must be processed efficiently to unlock its full potential. As industries continue to embrace Big Data, it has become clear that managing and analyzing these datasets is essential for gaining a competitive edge, driving innovation, and improving decision-making. Understanding Big Data in this context is crucial for organizations aiming to leverage its power to drive growth and success.
Business Intelligence (BI) plays a crucial role in transforming Big Data into meaningful insights. While Big Data involves vast amounts of information that are often complex and unstructured, BI systems focus on analyzing and visualizing that data in ways that are understandable and actionable for businesses. BI tools help organizations uncover patterns, trends, and correlations hidden within Big Data, which can drive better decision-making, operational improvements, and strategic growth.
By integrating and processing large datasets, BI enables businesses to make sense of overwhelming amounts of data and extract valuable insights to optimize their operations. With the ability to create reports, dashboards, and visualizations, BI helps businesses leverage Big Data for competitive advantage. In essence, Business Intelligence serves as a bridge between raw Big Data and practical, data-driven decisions that enhance overall business performance.
Business Intelligence (BI) and Big Data projects require a team of skilled professionals with expertise in various domains to ensure successful implementation, management, and analysis of data. These professionals come from diverse backgrounds such as data analysis, software development, and project management. BI and Big Data professionals play an essential role in collecting, processing, analyzing, and interpreting vast datasets to help organizations make data-driven decisions.
The complexity and scope of such projects demand a combination of technical, analytical, and business knowledge to drive growth, improve efficiency, and ensure data security. Each professional in the team contributes specific skills that support the overall objective of transforming raw data into valuable insights that are actionable and lead to better strategic decisions. Here’s a breakdown of the key professionals required for these projects:
Data scientists are essential in BI and Big Data projects because they are responsible for analyzing large and complex datasets to derive actionable insights. They have expertise in statistics, machine learning, and predictive modeling. Data scientists use advanced algorithms and techniques to explore data, identify patterns, and build predictive models that help businesses make informed decisions.
Their work is crucial in unlocking the potential of Big Data, ensuring that the data is not only collected but also analyzed to provide valuable insights. Data scientists often use programming languages such as Python, R, and SQL, along with tools like Hadoop and Spark, to manage and process massive datasets.
Data engineers focus on the infrastructure and architecture needed to collect, store, and process Big Data. They are responsible for building and maintaining the pipelines that allow data to flow from multiple sources into databases and analytics platforms. Data engineers ensure that the data is structured and organized in a way that makes it easy for analysts and data scientists to work with.
They typically work with technologies like Hadoop, Apache Kafka, and SQL databases and have a deep understanding of cloud platforms like AWS, Google Cloud, and Azure. By optimizing the data architecture, they ensure the scalability and efficiency of Big Data systems.
BI developers design and develop solutions that allow businesses to analyze data and make informed decisions. They create dashboards, reports, and visualizations that transform raw data into understandable insights for business users. BI developers are skilled in BI tools like Tableau, Power BI, and QlikView and have expertise in writing SQL queries to extract data from databases.
Their work involves collaborating with business stakeholders to understand reporting requirements and delivering user-friendly, actionable data visualizations. The role of BI developers is crucial in turning data into strategic business intelligence that can drive performance improvements and business growth.
Data analysts play a vital role in BI and Big Data projects by helping businesses interpret and analyze data to uncover trends and insights. They clean, process, and analyze data from various sources, providing actionable reports that help organizations make better business decisions. Unlike data scientists, who often build predictive models, data analysts focus more on descriptive analytics summarizing and presenting historical data.
They are proficient in using data analysis tools such as Excel, and SQL and visualization tools like Power BI and Tableau. By delivering actionable insights, data analysts help businesses optimize their operations and improve customer experiences.
Data architects are responsible for designing and maintaining the data infrastructure that supports Big Data and BI projects. They design data models and databases that ensure data is stored, accessed, and processed efficiently. Data architects work closely with data engineers and data scientists to develop systems that support large-scale data analytics.
They also ensure that data is secure and complies with data governance policies. Data architects must have expertise in database management systems (DBMS), data warehousing, and cloud platforms. Their work ensures that Big Data and BI systems are scalable, reliable, and accessible for analysis.
Project managers are crucial to the success of BI and Big Data projects, as they oversee the entire project lifecycle. They ensure that the project stays on track, within budget, and meets deadlines. Project managers coordinate the efforts of data scientists, engineers, analysts, and developers, ensuring that each team member is aligned with the project’s goals.
They also manage communication with stakeholders and ensure that the project meets the business requirements. In BI and Big Data projects, project managers need to have a solid understanding of both the technical and business aspects of the project to guide the team effectively.
Business analysts act as the bridge between technical teams and business stakeholders in BI and Big Data projects. They help define the business requirements for data analysis and ensure that the outcomes of the project align with organizational goals. Business analysts translate business needs into technical specifications for BI developers and data engineers, helping ensure that data solutions meet the needs of end-users.
They also assist in interpreting data insights and making recommendations for business strategies. A deep understanding of business operations, along with a strong grasp of data, makes business analysts essential for the success of BI and Big Data projects.
Database administrators (DBAs) are responsible for ensuring that the databases used in Big Data and BI projects are secure, reliable, and optimized for performance. They manage the creation, maintenance, and performance of databases, ensuring that data is stored and retrieved efficiently. DBAs play a critical role in ensuring that the data systems are scalable, secure, and compliant with regulations.
They are experts in database management systems like Oracle, SQL Server, and MySQL and often work closely with data engineers to optimize the performance of data infrastructure. Their work ensures that the data systems remain functional and meet business needs.
Machine learning engineers are responsible for designing and implementing algorithms that allow systems to learn from data and improve their performance over time. In BI and Big Data projects, machine learning engineers use large datasets to build models that can automate decision-making processes and predict future outcomes.
They work closely with data scientists to develop algorithms and ensure that the models are scalable and efficient. Machine learning engineers must have a strong background in programming, statistics, and data science tools like Python, R, and TensorFlow.
Data security specialists are responsible for ensuring that the data in BI and Big Data projects is protected from unauthorized access and breaches. As organizations handle vast amounts of sensitive data, the role of security specialists becomes increasingly important. They design and implement security protocols, manage encryption methods, and ensure compliance with data privacy regulations like GDPR and HIPAA.
Security specialists also monitor data systems for vulnerabilities and perform audits to prevent potential threats. Their expertise is crucial in ensuring that data remains safe, confidential, and compliant with regulatory requirements.
Business Intelligence (BI) and Big Data both play pivotal roles in transforming raw data into valuable insights for organizations. BI focuses on analyzing structured data to support decision-making through reporting, dashboards, and visualizations. It helps businesses understand past trends and performance. On the other hand, Big Data deals with large, complex datasets, including unstructured data, and requires advanced analytics like machine learning and predictive modeling. While BI helps streamline business processes with historical data, Big Data opens new avenues for innovation and future predictions. Both approaches are complementary and essential for modern businesses.
The choice between BI and Big Data depends on the specific needs of a business. For organizations seeking to improve operational efficiency and gain insights from historical data, BI is ideal. However, for those aiming to uncover patterns, predict future trends, and drive innovation, Big Data is a game-changer. In many cases, integrating both BI and Big Data strategies allows organizations to gain a holistic view of their operations, enabling them to make informed decisions and stay ahead of the competition.
Copy and paste below code to page Head section
Business Intelligence (BI) refers to the technologies, tools, and strategies used by businesses to analyze data and make informed decisions. BI systems help companies track key performance indicators (KPIs), identify trends, and uncover insights from data, enabling data-driven decision-making and improving business performance.
Big Data refers to vast and complex datasets that cannot be processed with traditional data processing methods. It includes structured, semi-structured, and unstructured data from various sources, such as social media, sensors, and weblogs. Big Data analysis helps organizations extract insights and patterns for improved decision-making and forecasting.
BI focuses on analyzing historical data to support decision-making, while Big Data involves the analysis of large, complex datasets, including real-time and unstructured data. BI uses traditional tools for analysis, whereas Big Data relies on advanced analytics, machine learning, and specialized tools to process massive datasets.
Key components of BI include data collection, data warehousing, data mining, reporting, dashboards, and data visualization. BI integrates these components to provide businesses with actionable insights from their data. It helps companies understand past performance and trends for better decision-making.
Common tools used in Big Data analysis include Apache Hadoop, Apache Spark, MongoDB, and NoSQL databases. These tools help process and analyze massive datasets in a scalable and efficient manner. Big Data platforms also include machine learning and artificial intelligence algorithms for predictive analytics.
Business Intelligence helps businesses by providing insights from data that drive informed decision-making. It allows companies to track and analyze performance metrics, optimize operations, and identify market trends. With BI, businesses can make data-driven decisions that improve profitability, efficiency, and customer satisfaction.