
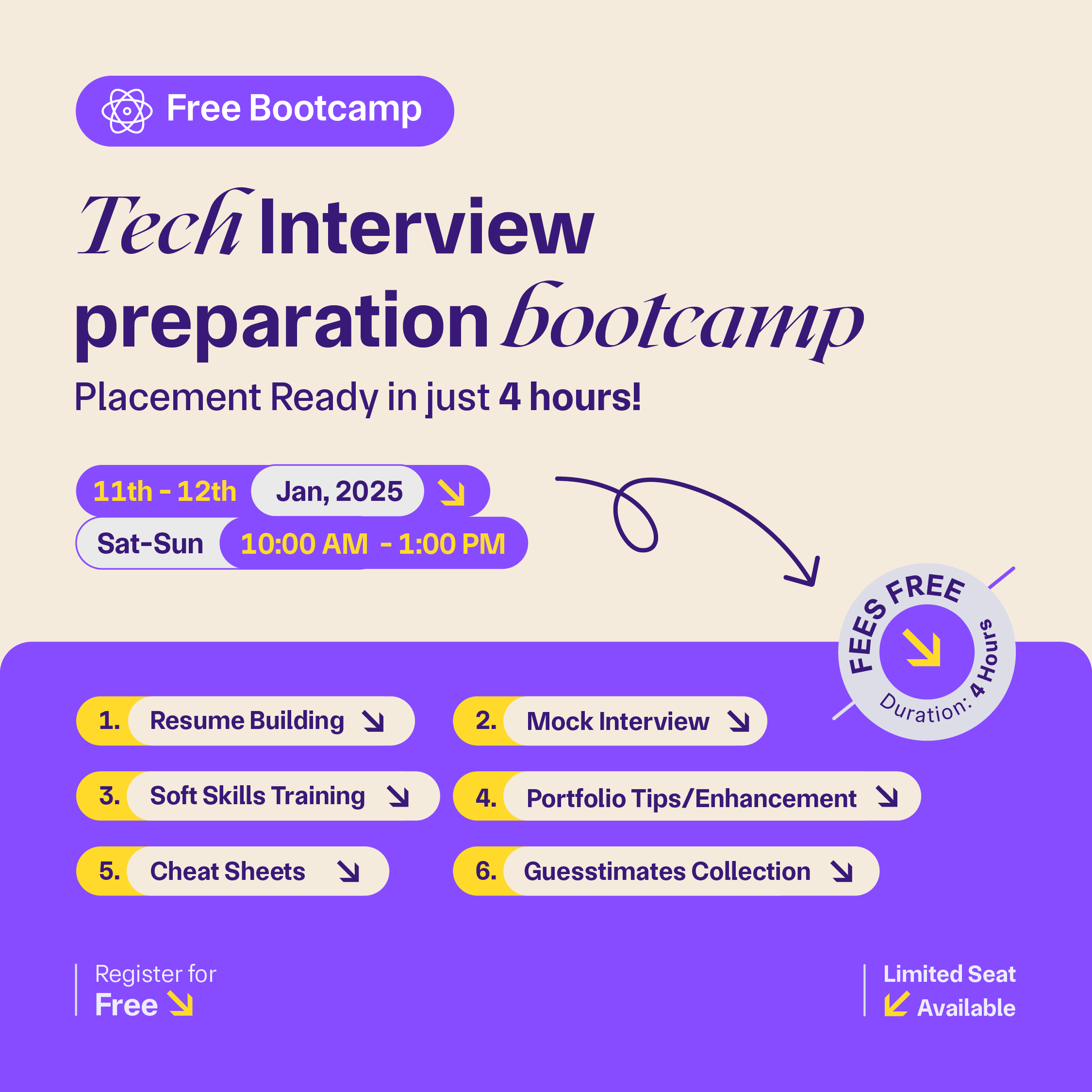
In today's data-driven world, the ability to analyze large volumes of data effectively is crucial for organizations to make informed decisions and maintain a competitive edge. Big Data Analytics tools are essential in managing, processing, and analyzing vast datasets to uncover hidden patterns, correlations, and insights. These tools help businesses transform raw data into actionable information, enabling better decision-making, enhanced operational efficiency, and innovative solutions to complex problems.
Big Data Analytics tools come in various forms, each designed to handle specific aspects of data processing and analysis. Some tools focus on data storage and management, while others specialize in data processing, visualization, or advanced analytics. Key tools in this domain include Hadoop, Apache Spark, Tableau, and SAS. Hadoop provides a framework for distributed storage and processing of large data sets, while Apache Spark offers fast, in-memory data processing capabilities. Tableau excels in data visualization, making it easier to interpret and communicate findings, and SAS provides robust analytics capabilities for statistical analysis and predictive modeling.
The importance of Big Data Analytics tools cannot be overstated, as they empower organizations to harness the full potential of their data. By leveraging these tools, businesses can gain deeper insights into customer behavior, optimize operations, and develop new revenue streams. As data continues to grow exponentially, the role of Big Data Analytics tools will become increasingly vital in helping organizations stay agile and responsive to market changes and emerging trends.
Big Data Analytics is the process of examining large and varied data sets—referred to as big data—to uncover hidden patterns, unknown correlations, market trends, customer preferences, and other useful business information.
This process leverages advanced analytical techniques, including machine learning, statistical analysis, and data mining, to transform vast amounts of structured, semi-structured, and unstructured data into actionable insights. Big data encompasses data that is too large, fast, or complex for traditional data processing tools.
This includes data generated from various sources such as social media, sensors, mobile devices, and transactional applications. Big Data Analytics involves several key steps: data collection, data storage, data cleaning and preparation, data analysis, and data visualization. These steps ensure that the raw data is converted into meaningful insights that can drive decision-making and strategy.
In 2025, big data analytics tools play a pivotal role in enabling organizations to extract actionable insights from large datasets, enhancing decision-making and operational efficiencies. These tools encompass a diverse range of capabilities, including robust data processing, efficient storage solutions, and advanced analytics functionalities.
From machine learning algorithms to sophisticated visualization tools, each offers unique strengths tailored to specific business requirements. Understanding the distinctive features and strengths of these tools empowers businesses to select the most suitable options, facilitating effective utilization of data-driven strategies to drive growth and competitive advantage in the digital age.
Apache Hadoop is an open-source framework designed for distributed processing of large datasets across clusters of computers. It utilizes Hadoop Distributed File System (HDFS) for scalable storage and MapReduce for parallel data processing, dividing tasks into smaller sub-tasks executed across nodes for efficient data handling.
Additional tools like Hive and Pig extend its functionality, supporting data warehousing and flow scripting within the Hadoop ecosystem. Hadoop's fault tolerance ensures data reliability by replicating data across nodes, and its active community contributes to continuous improvements and support. These features make Hadoop essential for enterprises managing vast amounts of data effectively.
Apache Spark is a fast, distributed computing system known for its in-memory data processing capabilities, significantly speeding up computations compared to disk-based systems like Hadoop's MapReduce. Supporting Java, Scala, Python, and R, Spark offers high-level APIs for diverse tasks such as SQL queries, machine learning with MLlib, graph processing using GraphX, and real-time analytics through Spark Streaming.
Its scalability and fault tolerance enable distributed data processing across clusters, recovering from node failures with checkpointed data. Spark's adoption in industry and academia is driven by its performance, versatility, and robust community support for integrating with other big data technologies.
Google BigQuery is a serverless, multi-cloud data warehouse designed for fast SQL queries and seamless integration with Google Cloud Platform services. It enables rapid analysis of large datasets without infrastructure management, supporting standard SQL for scalable and cost-effective data analytics and machine learning tasks.
BigQuery's serverless architecture scales automatically to handle varying workloads, leveraging Google's infrastructure for high performance and reliability. Its integration with other Google Cloud services simplifies data processing and management, making it a powerful choice for organizations seeking efficient cloud-based solutions for data-driven insights and decision-making.
AWS Redshift is a fully managed cloud data warehouse optimized for online analytical processing (OLAP), designed to handle complex queries on large datasets efficiently. Utilizing columnar storage for storing data by columns rather than rows, Redshift enhances query performance by minimizing disk I/O. It integrates seamlessly with AWS services such as S3 for data storage and IAM for security management, ensuring data durability, availability, and compliance with industry standards.
Redshift's automatic and manual concurrency scaling adjusts query processing slots based on workload demand, optimizing performance for thousands of concurrent queries. Its advanced security features and performance optimizations make it suitable for mission-critical big data analytics applications in cloud environments.
Tableau is a leading data visualization tool known for its intuitive interface and powerful analytics capabilities. It enables users to create interactive dashboards and visualizations from various data sources through drag-and-drop functionality. Supporting real-time data analytics and wide integration with databases, spreadsheets, and cloud services, Tableau facilitates comprehensive data exploration and reporting.
Collaboration features in Tableau Server and Online enhance teamwork by sharing visualizations and insights across organizations, promoting data-driven decision-making. Scalability and performance optimizations ensure Tableau handles large datasets efficiently, empowering businesses to derive meaningful insights and drive strategic initiatives with data visualization and analysis.
Microsoft Power BI is a robust business analytics service that empowers organizations to make informed decisions through interactive dashboards and reports. Integrated deeply with Microsoft Office and Azure services, Power BI offers AI-driven insights and natural language querying. It supports extensive connectivity options, making it versatile for data visualization and analytics across organizational functions.
Its interactive features facilitate quick decision-making by enabling users to explore data and uncover insights efficiently. Power BI's integration with Azure ensures scalability, security, and seamless deployment, enhancing its effectiveness in transforming data into actionable intelligence.
TensorFlow, developed by Google, is a versatile open-source machine learning framework renowned for building and training various models, including deep learning architectures. It supports deployment across CPUs, GPUs, and TPUs, making it scalable for diverse machine learning tasks. TensorFlow's integration with Google Cloud Platform ensures efficient utilization in cloud environments, enhancing its flexibility for research and production applications.
With extensive libraries and tools, TensorFlow simplifies complex machine learning workflows, empowering developers to create advanced models efficiently. Its robust ecosystem and community support further solidify TensorFlow's position as a leading framework for scalable and high-performance machine learning.
PyTorch is a flexible deep learning framework valued for its simplicity and seamless Python integration. It facilitates intuitive model development with dynamic computational graphs, supporting various neural network architectures like CNNs, RNNs, and Transformers.
PyTorch's deployment framework, TorchServe, ensures efficient model deployment in production settings, leveraging its research-friendly environment for practical AI applications. Supported by an active community, PyTorch accelerates the development cycle from research to deployment, making it favored for its ease of use and performance in building cutting-edge AI solutions.
Apache Kafka serves as a distributed event streaming platform designed to handle large-scale data streams with fault tolerance and horizontal scalability. It enables real-time data integration and processing through Kafka Streams, supporting operations like data transformations and aggregations across diverse data sources.
Kafka's integration with Apache ZooKeeper ensures reliable cluster management critical for maintaining data integrity and operational efficiency. Its architecture supports high throughput and low latency, making it ideal for applications requiring real-time data processing and stream analytics in distributed environments.
Splunk is a leading platform for real-time indexing, searching, and analysis of machine-generated data, essential for operational intelligence and security analytics. It excels in rapid data access and correlation across logs, metrics, and events, providing actionable insights for decision-making. Splunk's advanced machine learning capabilities enhance anomaly detection and predictive analytics, empowering organizations to detect and respond to issues proactively.
With customizable dashboards and comprehensive integration capabilities across cloud platforms and IoT devices, Splunk ensures scalable performance and compliance with security standards, making it indispensable for enterprise-level data operations and insights.
Elasticsearch is a highly scalable and distributed search and analytics engine known for its speed and ability to handle large volumes of data. It excels in real-time search scenarios, making it ideal for applications where fast query responses are crucial, such as logging and monitoring systems.
Elasticsearch uses a schema-free JSON (JavaScript Object Notation) document storage format, allowing it to index and search structured and unstructured data efficiently. It integrates seamlessly with Logstash for data ingestion and Kibana for data visualization, collectively forming the ELK stack (Elasticsearch, Logstash, and Kibana). This stack is widely used for log and event data analysis, offering powerful analytics and visualization capabilities.
MongoDB is a popular NoSQL database known for its flexibility, scalability, and performance. It uses a document-oriented data model, where data is stored in flexible, JSON-like documents, allowing for dynamic schema design. MongoDB supports horizontal scaling through sharding, distributing data across multiple nodes to handle large datasets and achieve high throughput.
It integrates well with big data processing frameworks like Hadoop and Spark, enabling real-time analytics and machine learning on MongoDB data. MongoDB's design focuses on ease of use and developer productivity, making it suitable for a wide range of applications requiring flexible data storage and high availability.
KNIME (Konstanz Information Miner) is an open-source data analytics and integration platform that simplifies the creation of data science workflows through a visual programming interface. It offers a drag-and-drop approach for building workflows, where users can connect nodes to perform tasks such as data preprocessing, modeling, and visualization.
KNIME supports collaboration among team members and provides an extensive library of nodes and integrations with various data sources and analytical tools. Its user-friendly interface makes it accessible for users with different levels of programming expertise, facilitating rapid development and deployment of data-driven solutions across industries.
RapidMiner is a data science platform that provides an integrated environment for data preparation, machine learning, and predictive model deployment. It features a visual workflow designer that allows users to create analytical workflows intuitively, without requiring extensive programming knowledge.
RapidMiner includes automated machine learning (AutoML) capabilities, which automate tasks such as model selection, hyperparameter optimization, and model deployment, speeding up the development cycle for machine learning projects. It integrates seamlessly with various data sources and platforms, supporting collaborative data science projects and enabling organizations to derive actionable insights from data efficiently.
Databricks is a unified analytics platform built on Apache Spark, designed for collaborative data science and machine learning at scale in cloud environments. It provides a unified workspace where data engineers, data scientists, and analysts can collaborate on data-driven projects.
Databricks optimizes Spark performance with Databricks Runtime, enhancing the speed and efficiency of data processing and analytics tasks. It supports seamless integration with popular data sources and machine learning libraries, facilitating the deployment of scalable and efficient data workflows. Databricks also prioritizes data security with robust features for encryption, access control, and compliance, ensuring data protection in cloud-based deployments.
Big data analytics plays a pivotal role in today's digital landscape, offering organizations transformative capabilities to extract meaningful insights from vast and diverse datasets.
By employing advanced techniques such as machine learning, data mining, and statistical analysis, big data analytics enables businesses to uncover hidden patterns, correlations, and trends that traditional methods might overlook.
This process empowers informed decision-making, enhances operational efficiencies, improves customer experiences, and fosters innovation. Moreover, it facilitates proactive risk management, supports regulatory compliance, and cultivates a data-driven culture that drives continuous improvement and competitive advantage in an increasingly dynamic and data-rich environment.
Big data refers to exceptionally large and complex datasets that surpass the processing capabilities of traditional software. These datasets are characterized by three primary dimensions known as the 3Vs: Volume, Velocity, and Variety.
Volume refers to the sheer scale of data generated, Velocity denotes the speed at which data is produced and processed, and Variety encompasses the diverse types of data sources and formats. Big data requires specialized tools and techniques to store, manage, and analyze effectively, offering valuable insights that can inform decision-making and drive innovation across industries.
Big data is not just about the size of the data but also about extracting valuable insights from it. Organizations use big data analytics tools and technologies to uncover patterns, trends, and associations that can inform decision-making, improve processes, and drive innovation across various industries.
Big data analytics is instrumental in deriving actionable insights from vast and diverse datasets to inform decision-making and improve business outcomes. Organizations leverage advanced analytics techniques to extract valuable information from sources such as social media, sensors, and transactional data.
By processing and analyzing these datasets, businesses can uncover hidden patterns, trends, and correlations that traditional methods might overlook. This capability enables enhanced operational efficiency, better customer understanding, predictive analytics for forecasting, personalized marketing strategies, and proactive risk management. Examples range from optimising supply chains to enhancing healthcare delivery and improving cybersecurity measures.
The history of big data analytics traces back to the early 2000s when the term "big data" emerged to describe the challenges posed by the exponential growth of data volumes and complexities.
Initially, industries like internet search and e-commerce faced unprecedented amounts of data, prompting the development of new technologies and methodologies to manage and analyze it effectively.
Over the years, advancements in storage, processing power, and algorithms have fueled the evolution of big data analytics, enabling organizations to extract valuable insights from vast datasets to drive decision-making, innovation, and operational efficiencies.
Big data analytics offers significant advantages to organizations by harnessing large and diverse datasets to derive actionable insights. It enables businesses to make informed decisions, enhance operational efficiencies, and gain competitive advantages in their respective industries.
By leveraging advanced analytical techniques, organizations can uncover patterns, trends, and correlations that drive innovation and improve overall business performance.
Big data analytics empowers organizations to base decisions on comprehensive data insights rather than guesswork. By analyzing large datasets, businesses can identify trends, patterns, and correlations that inform strategic choices, optimize processes, and predict outcomes, leading to more effective decision-making and resource allocation.
Optimizing operations through big data analytics involves streamlining workflows, improving resource allocation, and reducing inefficiencies. This leads to cost savings, enhanced productivity, and better utilization of resources across various departments and processes.
Access to timely insights enables businesses to capitalize on market opportunities swiftly. Big data analytics helps organizations stay ahead of competitors by identifying emerging trends, understanding customer preferences, and adapting strategies in response to market dynamics.
Analyzing customer data allows businesses to create personalized experiences and targeted marketing campaigns. By understanding customer behaviors, preferences, and needs, organizations can improve customer satisfaction, loyalty, and retention rates, ultimately driving business growth and profitability.
Big data analytics fuels innovation by uncovering market gaps, identifying new product opportunities, and optimizing existing offerings based on consumer feedback and market demand. This iterative process of product development ensures that businesses deliver relevant and competitive solutions that meet evolving customer expectations.
Identifying and mitigating risks through big data analytics involves detecting anomalies, predicting potential threats, and implementing proactive measures to safeguard against operational disruptions, cybersecurity breaches, and financial losses. This proactive approach to risk management enhances organizational resilience and protects brand reputation.
Measuring performance metrics with big data analytics enables organizations to track key indicators, assess operational effectiveness, and identify areas for improvement. By analyzing data-driven insights, businesses can set benchmarks, monitor progress, and optimize strategies to achieve better outcomes and meet strategic goals effectively.
The capability to process and analyze data in real-time enables businesses to make immediate decisions, respond promptly to market changes, and address operational challenges swiftly. Real-time analytics enhances agility, facilitates proactive decision-making, and improves operational responsiveness across all levels of the organization.
Big data analytics contributes to cost efficiency by optimizing resource allocation, reducing wastage, and enhancing operational workflows. By identifying cost-saving opportunities and streamlining processes, organizations can achieve significant savings while maintaining or improving service delivery and customer satisfaction.
Scalability in big data analytics platforms allows businesses to handle increasing data volumes and complexities as they grow. Flexible infrastructure and scalable solutions ensure that organizations can expand their data capabilities seamlessly, supporting ongoing business growth and adapting to changing operational needs and demands.
The lifecycle of big data analytics encompasses structured phases that guide the process of deriving insights from large and complex datasets. It begins with data acquisition and preparation, progresses through analysis and interpretation, and concludes with action and continuous monitoring. Each phase plays a crucial role in leveraging data effectively to inform decision-making, optimize operations, and drive business outcomes in diverse industries.
This initial phase involves sourcing data from various internal and external sources, including databases, IoT devices, social media platforms, and more. Data integration ensures that diverse datasets are combined and structured in a format suitable for analysis, laying the foundation for meaningful insights.
In this stage, raw data undergoes cleaning, filtering, and transformation to ensure accuracy, consistency, and relevance. Data preparation tasks include handling missing values, removing duplicates, standardizing formats, and aggregating data points as necessary to facilitate efficient analysis.
Data analysis encompasses applying statistical techniques, machine learning algorithms, and visualization tools to uncover patterns, trends, and correlations within the dataset. This phase aims to extract actionable insights that address specific business questions or objectives identified earlier in the process.
Interpreting the results of data analysis involves understanding the implications of findings in relation to business goals. It requires domain expertise to contextualize insights and derive actionable recommendations that drive strategic decisions and operational improvements.
Based on insights gained, organizations take action to implement changes, strategies, or initiatives aimed at capitalizing on opportunities or addressing challenges identified through data analysis. This phase may involve deploying new processes, products, or marketing campaigns informed by data-driven insights.
The final phase involves ongoing monitoring of implemented strategies and performance metrics to assess effectiveness and identify further optimization opportunities. Continuous monitoring ensures that insights remain relevant and that organizations can adapt swiftly to evolving business conditions and new data trends.
Big data analytics encompasses various types of analytical approaches that organizations use to derive insights from large and complex datasets. These approaches differ in their objectives, methodologies, and applications, catering to different business needs and industry requirements.
From descriptive analytics that summarize historical data to predictive analytics that forecast future trends, each type serves specific purposes in informing decision-making, improving operational efficiencies, and driving innovation across diverse sectors.
Descriptive analytics involves summarizing historical data to understand past trends and events. It utilizes techniques such as data aggregation, visualization, and reporting to provide insights into what has happened within an organization or market.
By examining historical data patterns, businesses can gain a comprehensive overview of their operations and performance, facilitating informed decision-making and strategic planning based on past outcomes and trends.
Diagnostic analytics goes beyond descriptive analytics by identifying the root causes of past events or outcomes. It involves deeper analysis to uncover relationships, patterns, and anomalies in data, helping organizations understand why certain events occurred.
This type of analytics is crucial for troubleshooting issues, optimizing processes, and improving performance based on a thorough understanding of historical data insights.
Predictive analytics utilizes statistical models and machine learning algorithms to forecast future trends and behaviors based on historical data patterns.
By analyzing past data, predictive analytics enables organizations to anticipate outcomes, mitigate risks, and identify opportunities before they arise. This proactive approach to decision-making empowers businesses to optimize strategies, allocate resources effectively, and stay ahead in competitive markets.
Prescriptive analytics integrates predictive analytics with optimization techniques to recommend actions that optimize desired outcomes. It not only predicts what is likely to happen but also suggests the best course of action to achieve specific business objectives.
By considering various constraints and objectives, prescriptive analytics helps organizations make data-driven decisions that maximize efficiency, profitability, and customer satisfaction.
Text analytics focuses on extracting insights and meaning from unstructured textual data sources such as customer reviews, social media posts, and documents. Using techniques like natural language processing (NLP), text analytics analyzes sentiment, identifies entities, and extracts topics to uncover valuable insights.
Organizations can use these insights to understand customer feedback, trends in public opinion, and emerging issues, guiding strategic decisions and enhancing customer engagement strategies.
Spatial analytics analyzes geographic or location-based data to uncover insights related to spatial patterns, relationships, and trends. It is used in various fields such as urban planning, logistics optimization, and environmental monitoring where geographic context is crucial for decision-making.
By visualizing and analyzing spatial data, organizations can optimize resource allocation, improve operational efficiency, and make informed decisions based on geographic insights.
Streaming analytics processes and analyzes real-time data streams to provide immediate insights and enable timely actions. It is essential for applications where data needs to be processed and acted upon in real-time, such as real-time fraud detection, IoT data processing, and operational monitoring.
By continuously analyzing streaming data, organizations can detect anomalies, respond quickly to changing conditions, and optimize processes in dynamic environments, enhancing operational agility and decision-making capabilities.
Big data analytics involves the collection, processing, and analysis of large volumes of data to uncover insights and trends that can inform decision-making. This field utilizes various tools and technologies designed to handle the complexities of big data, including storage, processing, and visualization.
Companies employ tools like Hadoop, Spark, and Kafka for data processing and real-time analytics. Data warehouses such as Amazon Redshift and Google BigQuery enable efficient storage and retrieval.
Visualization tools like Tableau and Power BI help interpret data visually. Machine learning frameworks like TensorFlow and PyTorch enhance predictive analytics capabilities, making big data analytics pivotal in modern business strategies.
These tools and technologies form the backbone of modern big data analytics infrastructures, enabling organizations to process, store, analyze, and visualize massive amounts of data efficiently and effectively.
Big data applications span across various industries, revolutionizing how organizations manage and utilize information. This field leverages advanced analytics to extract valuable insights from vast datasets, driving decision-making and innovation. Industries such as healthcare, finance, retail, and manufacturing benefit significantly from big data's capabilities.
By analyzing customer behaviour, optimizing supply chains, improving healthcare outcomes, and enhancing financial risk management, big data enables businesses to streamline operations, personalize customer experiences, and gain competitive advantages in today's data-driven economy.
Big data analytics tools encompass a range of technologies and frameworks designed to process, analyse, and derive insights from vast volumes of data that traditional data processing software and databases cannot handle effectively. These tools are essential for organizations looking to extract valuable insights, make data-driven decisions, and gain competitive advantages in various industries.
Big data analytics tools continue to evolve rapidly, leveraging innovations in cloud computing, artificial intelligence, and machine learning to meet the growing demands of organisations seeking to harness the power of their data for strategic decision-making and operational efficiencies.
Selecting the best big data analytics tools involves considering several critical factors that align with the specific needs and objectives of an organization. These factors ensure that the chosen tools can effectively handle the volume, variety, velocity, and veracity of data, while also supporting analytical needs and business goals.
1. Data Types and Variety: Consider the diversity and complexity of your data, including structured, semi-structured, and unstructured formats. Choose a tool that can effectively handle the specific types of data you work with, ensuring compatibility and optimal performance.
2. Data Volume and Scalability: Assess the volume and growth rate of your data. Select a tool that scales seamlessly to manage large datasets without compromising performance or requiring extensive infrastructure upgrades.
3. Analytical Requirements: Determine the complexity and depth of analysis needed for your business objectives. Opt for tools that offer advanced analytics capabilities such as machine learning, predictive modeling, and real-time analytics, aligned with your analytical requirements.
4. Budget and Cost Considerations: Evaluate the total cost of ownership, including licensing fees, infrastructure costs, and maintenance expenses. Choose a tool that fits within your budget constraints while offering the necessary features and scalability to support your analytics initiatives.
5. Integration with Existing Systems: Ensure compatibility and smooth integration with your existing IT ecosystem, including databases, data warehouses, and other analytics platforms. Look for tools with robust APIs, connectors, and support for data integration protocols.
6. User Experience and Ease of Use: Consider the tool's user interface, ease of deployment, and usability features. Opt for tools that offer intuitive dashboards, interactive visualisations, and user-friendly functionalities to empower data analysts and business users.
By carefully evaluating these factors, organizations can make informed decisions when selecting big data analytics tools that effectively meet their specific data analytics requirements, operational needs, and strategic objectives.
Data analysis tools are utilized by a diverse range of professionals and organizations across industries to derive insights, make informed decisions, and solve complex problems using data-driven approaches.
These tools enable users to process, manipulate, and interpret large volumes of data efficiently, uncovering patterns, trends, and correlations that inform strategic business decisions and operational improvements.
Data analysis in business involves leveraging tools and techniques to analyze market trends, customer preferences, and operational data. It helps businesses make informed decisions, improve efficiency in processes like supply chain management, and optimize marketing strategies based on consumer behavior and competitor analysis. By interpreting data insights, businesses can identify growth opportunities, mitigate risks, and enhance overall performance in a competitive market landscape.
Executives: Use data analysis tools to make strategic decisions, track company performance, and identify growth opportunities. By analyzing sales figures, market trends, and operational metrics, executives can develop informed strategies to drive business growth. Data insights help in identifying new market opportunities, improving product offerings, and enhancing overall company performance.
Operations Managers: Optimize processes, improve efficiency, and reduce costs by analyzing operational data. Data analysis enables operations managers to identify bottlenecks, streamline workflows, and enhance productivity. By monitoring key performance indicators (KPIs), they can implement data-driven decisions to improve operational efficiency and cost-effectiveness.
Sales and Marketing Teams: Identify customer trends, track campaign performance, and personalize marketing efforts. Data analysis tools allow sales and marketing teams to segment their audience, tailor marketing campaigns, and measure their effectiveness. By understanding customer behavior, they can enhance engagement, boost sales, and achieve better marketing ROI.
Data analysis tools in healthcare enable professionals to analyze patient records, treatment outcomes, and medical research data. This analysis aids in diagnosing diseases, predicting health trends, and improving clinical decision-making.
By harnessing data, healthcare providers can personalize patient care, streamline operations, and allocate resources effectively to enhance patient outcomes and reduce healthcare costs.
Doctors and Clinicians: Analyze patient data for better diagnosis and treatment plans. By leveraging data analysis, healthcare professionals can identify patterns in patient records, track treatment outcomes, and make evidence-based decisions. This helps in providing personalized care, improving patient outcomes, and optimizing treatment protocols.
Healthcare Administrators: Improve hospital operations, reduce costs, and enhance patient care through data insights. Administrators use data analysis to monitor hospital performance, manage resources efficiently, and streamline administrative processes. This leads to cost savings, improved patient care, and better overall hospital management.
Researchers: Conduct medical research by analyzing clinical trial data and health records. Data analysis tools enable researchers to identify trends, correlations, and causal relationships in medical data. This supports the development of new treatments, medical advancements, and a deeper understanding of health conditions.
In finance, data analysis plays a critical role in assessing market trends, managing investment portfolios, and minimizing financial risks. It involves analyzing economic indicators, stock market data, and customer financial behaviors to optimize investment strategies and ensure regulatory compliance.
Data analysis tools in finance also facilitate fraud detection, credit scoring, and real-time monitoring of financial transactions, contributing to financial stability and profitability.
Financial Analysts: Predict market trends, assess investment opportunities, and manage risks. Data analysis tools help financial analysts to evaluate historical data, forecast future trends, and make informed investment decisions. This aids in portfolio management, risk assessment, and identifying profitable opportunities.
Accountants: Ensure compliance, conduct audits, and improve financial reporting accuracy. By analyzing financial data, accountants can detect discrepancies, ensure regulatory compliance, and enhance the accuracy of financial statements. Data analysis supports effective financial management and auditing processes.
Banking Professionals: Enhance customer services, detect fraud, and manage credit risks. Data analysis tools enable banking professionals to analyze customer data, monitor transactions, and identify suspicious activities. This helps in improving customer experience, preventing fraud, and managing financial risks effectively.
Data analysis in marketing revolves around understanding customer behavior, measuring campaign performance, and optimizing marketing spend. Marketers use data analytics to segment audiences based on demographics and behaviors, personalize marketing messages, and forecast sales trends.
This insights-driven approach helps in identifying the most effective marketing channels, improving customer engagement, and maximizing return on investment (ROI) through targeted marketing campaigns.
Digital Marketers: Track online campaign performance, optimize advertising spends, and improve customer engagement. By analyzing data from digital channels, marketers can measure the effectiveness of campaigns, adjust strategies in real-time, and enhance customer interactions. This leads to better ROI and more targeted marketing efforts.
Market Researchers: Conduct market analysis, understand consumer behavior, and forecast market trends. Data analysis allows market researchers to gather insights on market dynamics, competitor strategies, and consumer preferences. This information is crucial for making informed marketing decisions and staying ahead in the market.
Product Managers: Use data to refine product strategies, improve user experience, and drive innovation. Data analysis tools help product managers to gather user feedback, analyze usage patterns, and identify areas for improvement. This supports the development of better products, enhanced user satisfaction, and innovative solutions.
Data analysis is fundamental in the technology sector for optimizing software development processes, improving user experiences, and enhancing cybersecurity measures. It involves analyzing system performance metrics, user feedback, and security logs to identify vulnerabilities, optimize software functionalities, and ensure seamless operation of digital services.
Data-driven insights also drive innovation by guiding product development decisions and predicting future technological trends, enabling organizations to stay competitive and meet evolving consumer demands.
Data Scientists: Develop algorithms, build predictive models, and uncover hidden patterns in data. Data scientists use advanced analysis techniques to transform raw data into actionable insights. This involves creating models that can predict trends, identify anomalies, and provide strategic recommendations.
Software Engineers: Enhance software functionality by analyzing user data and application performance. By leveraging data analysis, software engineers can optimize code, improve application performance, and enhance user experience. This leads to more efficient, reliable, and user-friendly software solutions.
IT Managers: Monitor and improve IT infrastructure performance and security. Data analysis tools enable IT managers to track system performance, identify potential issues, and implement security measures. This ensures a robust, secure, and efficient IT environment.
Big data analytics tools are essential for processing and deriving insights from vast amounts of data. However, their implementation and use come with significant challenges. Organizations often struggle with data integration, storage scalability, and the complexity of managing large datasets.
Additionally, ensuring data quality, maintaining data security, and finding skilled personnel to operate these tools are ongoing concerns. These challenges can hinder the effectiveness of big data initiatives and require strategic planning and resource allocation to overcome.
1. Data Integration: Combining data from various sources is complex and time-consuming. Different data formats and structures require robust data integration tools and techniques to ensure seamless data flow and consistency.
2. Scalability: Managing and scaling infrastructure to handle the increasing volume of big data is challenging. Organizations need to invest in scalable storage solutions and distributed computing frameworks to accommodate growing data needs.
3. Data Quality: Ensuring data accuracy, completeness, and consistency is critical for meaningful analysis. Poor data quality can lead to incorrect insights and decisions, necessitating rigorous data cleansing and validation processes.
4. Data Security and Privacy: Protecting sensitive data from breaches and ensuring compliance with privacy regulations is a major concern. Implementing robust security measures and maintaining data privacy is essential to safeguard data integrity.
5. Skilled Workforce: There is a high demand for professionals skilled in big data analytics, but the supply is limited. Organizations often face challenges in hiring and retaining qualified data scientists, analysts, and engineers to manage and analyze big data.
6. Cost Management: Implementing and maintaining big data analytics tools can be expensive. Costs associated with infrastructure, software licenses, and skilled personnel can strain budgets, requiring careful cost management and resource allocation.
7. Data Governance: Establishing effective data governance policies and procedures is crucial for managing data integrity, security, and compliance. Organizations must ensure that data governance frameworks are in place to manage data assets responsibly.
8. Complexity of Tools: Big data analytics tools often have steep learning curves and require specialized knowledge. Training staff to effectively use these tools and keeping up with rapid technological advancements can be challenging.
9. Real-Time Processing: Processing and analyzing data in real-time requires advanced tools and infrastructure. Ensuring that systems can handle real-time data streams and provide timely insights is a technical and logistical challenge.
10. Interoperability: Ensuring that different big data tools and systems can work together seamlessly is crucial for efficient data processing. Organizations must address interoperability issues to enable smooth data integration and analysis across various platforms.
Addressing these challenges requires a comprehensive approach, including investing in the right technologies, developing skilled teams, and establishing robust data management practices.
Big data analytics tools are indispensable in today's data-driven world, enabling organizations to extract valuable insights from vast and complex datasets. These tools offer capabilities for data processing, storage, visualization, and advanced analytics, helping businesses to make informed decisions, enhance operational efficiency, and drive innovation. However, their implementation is fraught with challenges such as data integration, scalability, data quality, security, and the need for skilled professionals.
Successfully leveraging big data analytics tools requires strategic planning, investment in technology and talent, and robust data governance practices. By addressing these challenges, organizations can harness the full potential of big data analytics, turning raw data into actionable insights and achieving a competitive advantage in their respective industries. As the field of big data continues to evolve, staying abreast of technological advancements and best practices will be crucial for maximizing the benefits of these powerful tools.
Copy and paste below code to page Head section
Big data analytics tools are specialized software and frameworks designed to process and analyze large volumes of data that traditional databases and software cannot manage effectively. They enable organizations to extract valuable insights and patterns from complex datasets, supporting informed decision-making and strategic planning.
These tools are crucial because they empower businesses to uncover hidden patterns, trends, and correlations within vast amounts of data. By processing data in real-time or near-real-time, organizations can gain actionable insights faster, optimize operations, and enhance customer experiences, ultimately driving competitive advantage in today's data-driven economy.
Key features include scalability to handle massive datasets, advanced analytics capabilities such as machine learning and predictive modeling, integration with diverse data sources, real-time data processing, and intuitive interfaces for data visualization and exploration.
Unlike traditional analytics tools that handle structured data in smaller volumes, big data analytics tools excel in processing large volumes of structured, semi-structured, and unstructured data at high velocities. They leverage distributed computing and advanced algorithms to derive insights from complex data sets that traditional tools may struggle to handle.
Industries such as finance, healthcare, retail, telecommunications, and manufacturing benefit significantly from big data analytics tools. These tools enable industries to improve operational efficiencies, enhance customer insights, optimize supply chain management, and drive innovation through data-driven decision-making processes.
Popular tools include Apache Hadoop, known for its distributed processing capabilities; Apache Spark, favored for its speed and in-memory processing; Google BigQuery, recognized for its serverless and scalable data warehousing; AWS Redshift, valued for its data warehousing and analytics capabilities; and Tableau and Power BI, renowned for their interactive data visualization and business intelligence features.